Phase Segmentation Of Uncured Prepreg X-Ray Ct Micrographs
COMPOSITES PART A-APPLIED SCIENCE AND MANUFACTURING(2021)
摘要
This paper explores methods to investigate voids and dry fibre areas in pre-impregnated aligned carbon fibre reinforced epoxy lay-ups. A deep learning segmentation approach was compared to conventional thresholding techniques to characterise the interlaminar voids (entrapped air) and dry areas (unsaturated fibre bed) phases obtained by micro-CT scanning of samples from uncured laminates. The performance of both approaches was quantitatively assessed in three regions of interest having different levels of porosity, ranging from a low 1% to a high 25%. Deep learning consistently outperformed thresholding in the segmentation of both interlaminar voidage and dry areas. Furthermore, deep learning improved the ability to detect small voids and was able to accurately segment voids in volumes with less than 2% voidage, whereas thresholding techniques fail in this task. Finally, the application of deep learning to the segmentation of dry areas in micro-CT scans provided sharper results than thresholding, without needing filtering.
更多查看译文
关键词
A, Prepreg, B, Porosity, D, CT analysis, Machine learning
AI 理解论文
溯源树
样例
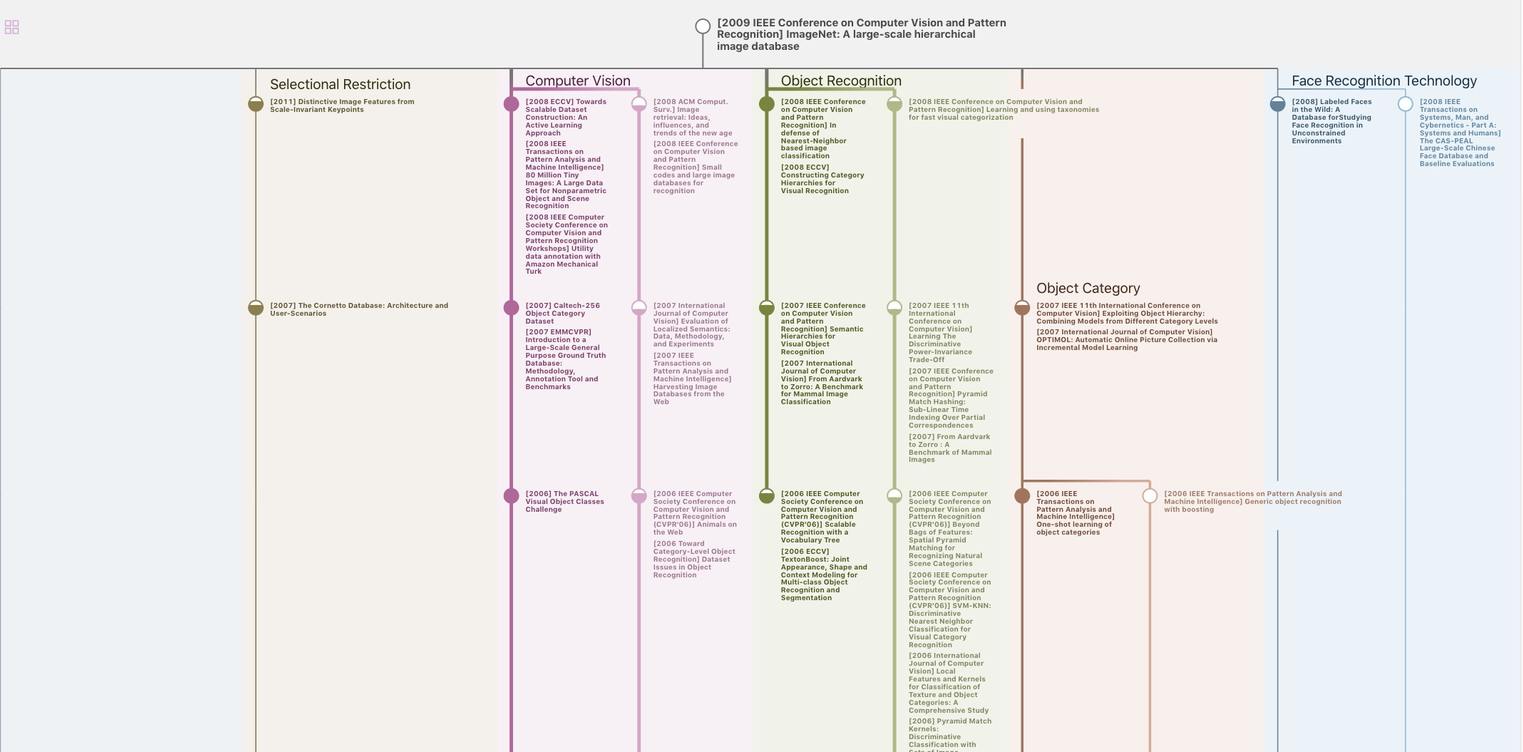
生成溯源树,研究论文发展脉络
Chat Paper
正在生成论文摘要