Learning to Walk: Modeling Transportation Mode Choice Distribution Through Neural Networks
Environment and planning B, Urban analytics and city science/Environment & planning B, Urban analytics and city science(2019)
摘要
Transportation mode distribution has a large implication on the resilience, economic output, social cost of cities and the health of urban residents. Recent advances in artificial intelligence and the availability of remote sensing data have opened up opportunities for bottom-up modeling techniques that allow understanding of how subtle differences in the urban fabric can impact transportation mode share distribution. This project presents a novel neural network-based modeling technique capable of predicting transportation mode distribution. Trained with millions of images labeled with information from a georeferenced transportation survey, the resulting model is able to infer transportation mode share with high accuracy ( R2 = 0.58) from satellite images alone. Additionally, this method can disaggregate data in areas where only aggregated information is available and infer transportation mode share in areas without underlying information. This work demonstrates a new and objective method to evaluate the impact of the urban fabric on transportation mode share. The methodology is robust and can be adapted for cases around the world as well as deployed to evaluate the impact of new developments on the transportation mode choice.
更多查看译文
关键词
Transportation modeling,neural networks,machine learning,transportation mode share,walkability
AI 理解论文
溯源树
样例
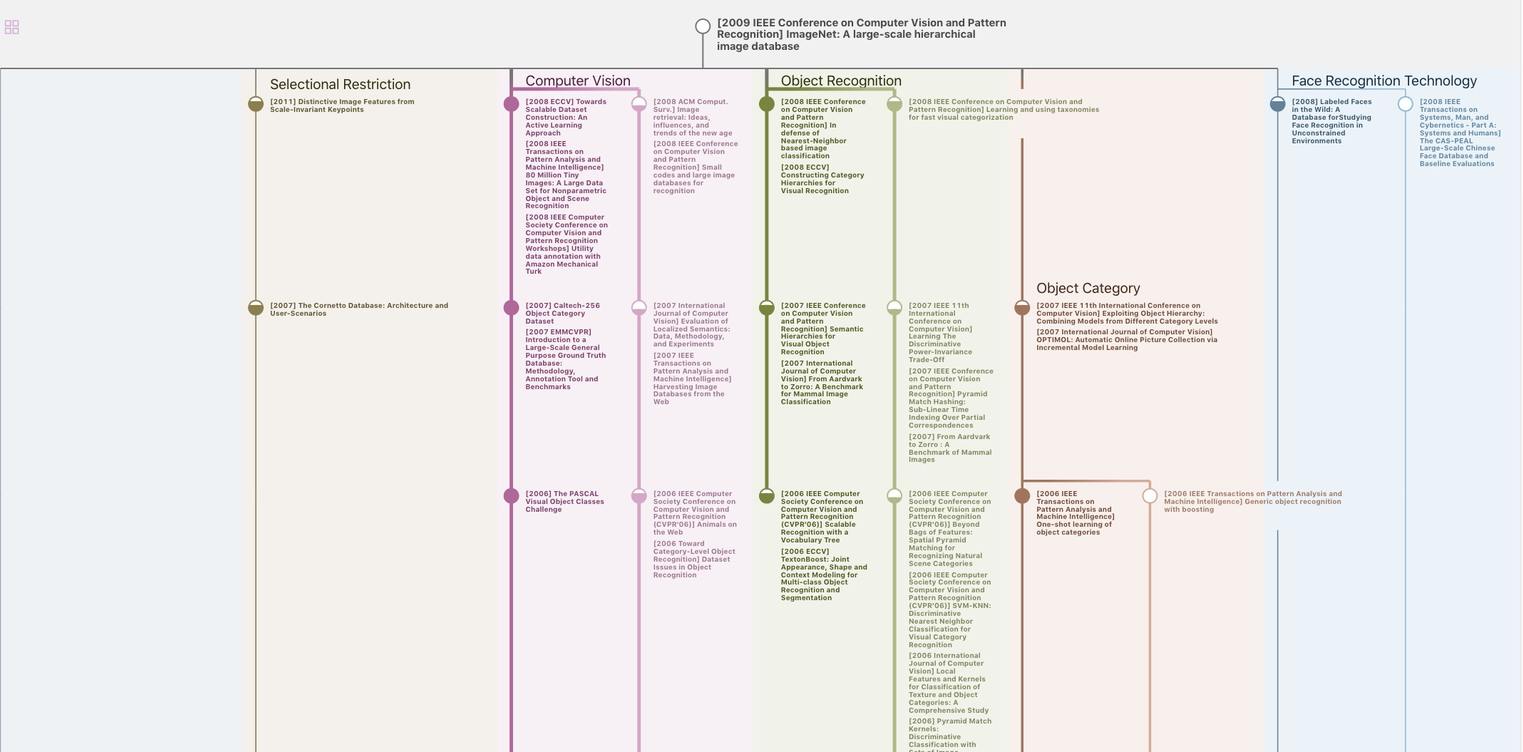
生成溯源树,研究论文发展脉络
Chat Paper
正在生成论文摘要