Hyperparameter Free Mee-Fp Based Localization
IEEE SIGNAL PROCESSING LETTERS(2021)
摘要
In the context of outdoor localization over systems impaired by non-line of sight (NLoS), the minimum error entropy with fiducial points (MEE-FP) based methods have emerged as promising due to their excellent generalization and independence to statistics of NLoS. However, the performance of these approaches are well-known to depend on hyperparameters, such as, the spread parameter of the MEE-FP criterion. To enable MEE-FP based hyperparameter-free localization, we propose a modified Gauss-Newton based localization algorithm based on sampled kernel-widths. Next, analytical results are derived to demonstrate the asymptotic equivalence of the proposed kernel-width sampling based localization algorithm to its ideal fixed kernel width based counterpart. This equivalence is validated through computer simulations assuming typical non-Gaussian NLoS distributions, which motivates the hyperparameter-independence of the proposed localization algorithm and its generalization to different NLoS statistics.
更多查看译文
关键词
Location awareness, Kernel, Signal processing algorithms, Probabilistic logic, Newton method, Entropy, Steady-state, Localization, information theoretic learning, minimum error entropy, hyperparameter-free learning
AI 理解论文
溯源树
样例
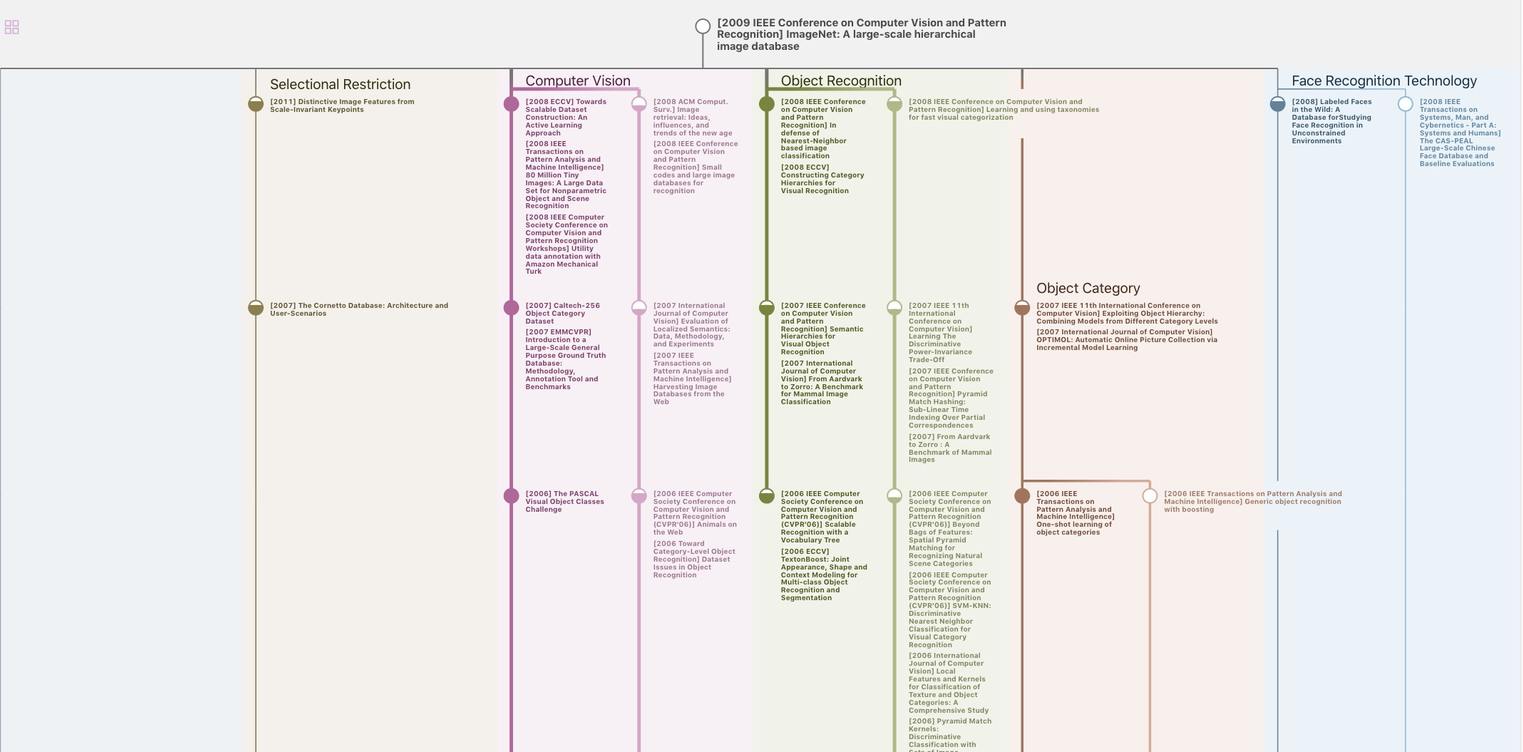
生成溯源树,研究论文发展脉络
Chat Paper
正在生成论文摘要