Deciphering Tissue Relaxation Parameters From A Single Mr Image Using Deep Learning
MEDICAL IMAGING 2020: COMPUTER-AIDED DIAGNOSIS(2020)
摘要
Despite its superior soft tissue contrast, conventional MRI is qualitative in nature and this presents a bottleneck problem in quantitative image analysis and data-driven medicine. Various investigations have been devoted to overcoming this limitation, but practical solutions remain elusive. Leveraging from the unique ability of emerging deep learning in feature extraction, we investigate a data-driven strategy of separating contributions of various contributing factors intertwined in a single T-1 weighted image to derive quantitative T-1 and rho maps without any additional image acquisition. Furthermore, in the proposed deep learning framework, compensation for radiofrequency field inhomogeneities is automatically achieved without extra measurement of B-1 map. The tasks are accomplished using self-attention deep convolutional neural networks, which make efficient use of both local and non-local information. The premise of the approach is that qualitative and quantitative MRI, named Q(2)MRI, can be attained simultaneously without changing the existing imaging protocol. Q(2)MRI lays foundation for next generation of digital medicine and provides a promising quantitative imaging tool for a wide spectrum of biomedical applications, ranging from disease diagnosis, treatment planning, prognosis to assessment of therapeutic response.
更多查看译文
AI 理解论文
溯源树
样例
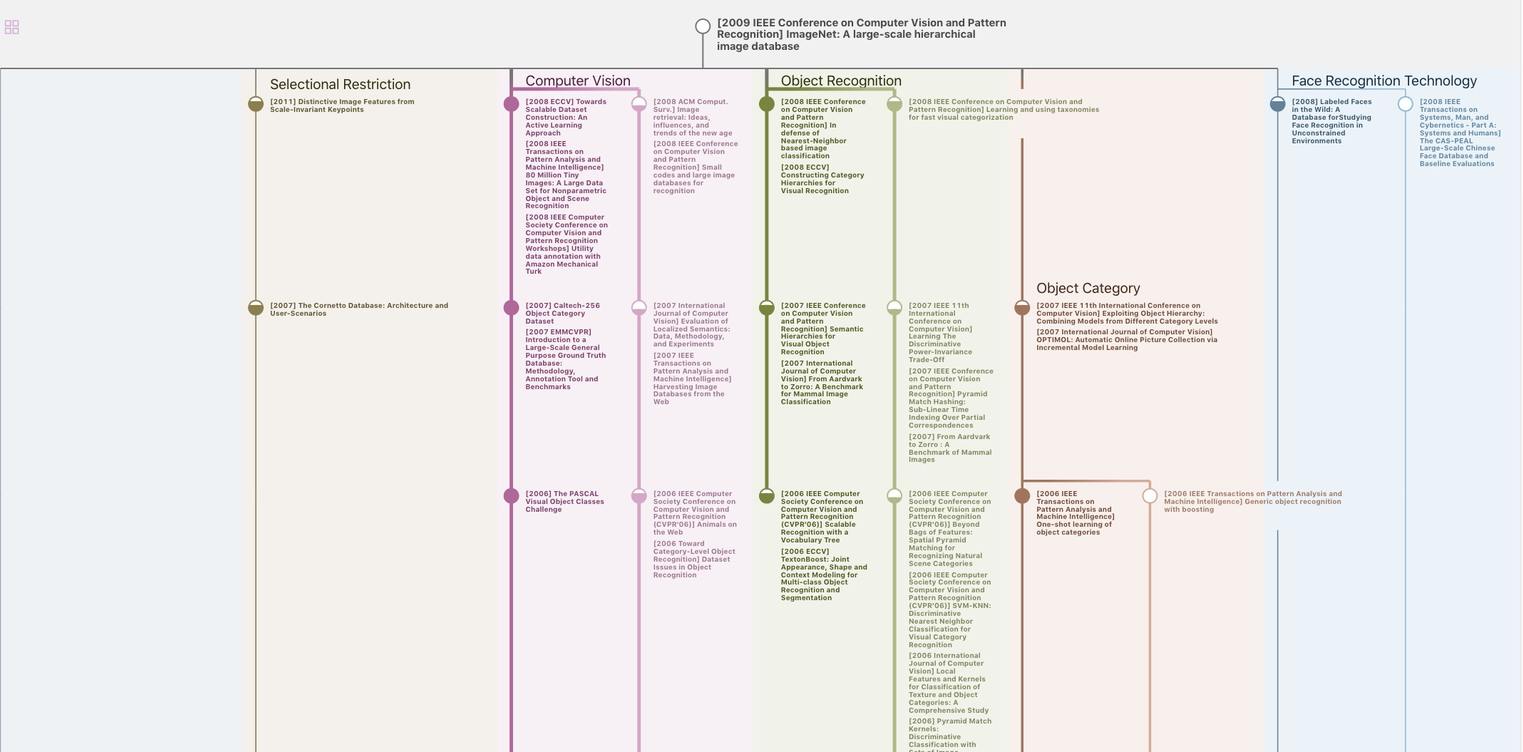
生成溯源树,研究论文发展脉络
Chat Paper
正在生成论文摘要