A Deep Learning Based Integration Of Multiple Texture Patterns From Intensity, Gradient And Curvature Glcms In Differentiating The Malignant From Benign Polyps
MEDICAL IMAGING 2020: COMPUTER-AIDED DIAGNOSIS(2020)
摘要
Deep learning such as Convolutional Neural Network (CNN) has demonstrated its superior in the field of image analysis. However, in the medical imaging field, deep learning faces more challenges for tumor classification in computer-aided diagnosis due to uncertainties of lesions including their size, scaling factor, rotation, shapes, etc. Thus, instead of feeding raw images, texture-based CNN model has been designed to classify the objects with their good attributes. For example, gray level co-occurrence matrix (GLCM) can be chosen as the descriptor of the texture pattern for many good properties such as uniform size, shape invariance, scaling invariance. However, there are many different texture metrics to measure the different texture patterns. Thus, an effective and efficient integration model is essential to further improve the classification performance from different texture patterns. In this paper, we proposed a multi-channel texture-based CNN model to effectively integrate intensity, gradient and curvature texture patterns together for differentiating the malignant from benign polyps. Performance was evaluated by the merit of area under the curve of receiver operating characteristics (AUC). Around 0.3 similar to 4.8% improvement has been observed by combining different texture patterns together. Finally, classification performance of AUC=86.7% has been achieved for a polyp mass dataset of 87 samples, which obtains 1.8% improvement compared with a state-of-the-art method. The results indicate that texture information from different metrics could be fused and classified with a better classification performance. It also sheds lights that data integration is important and indispensable to pursuit improvement in classification task.
更多查看译文
关键词
Polyp Classification,Deep Learning,Texture GLCM,Data Integration
AI 理解论文
溯源树
样例
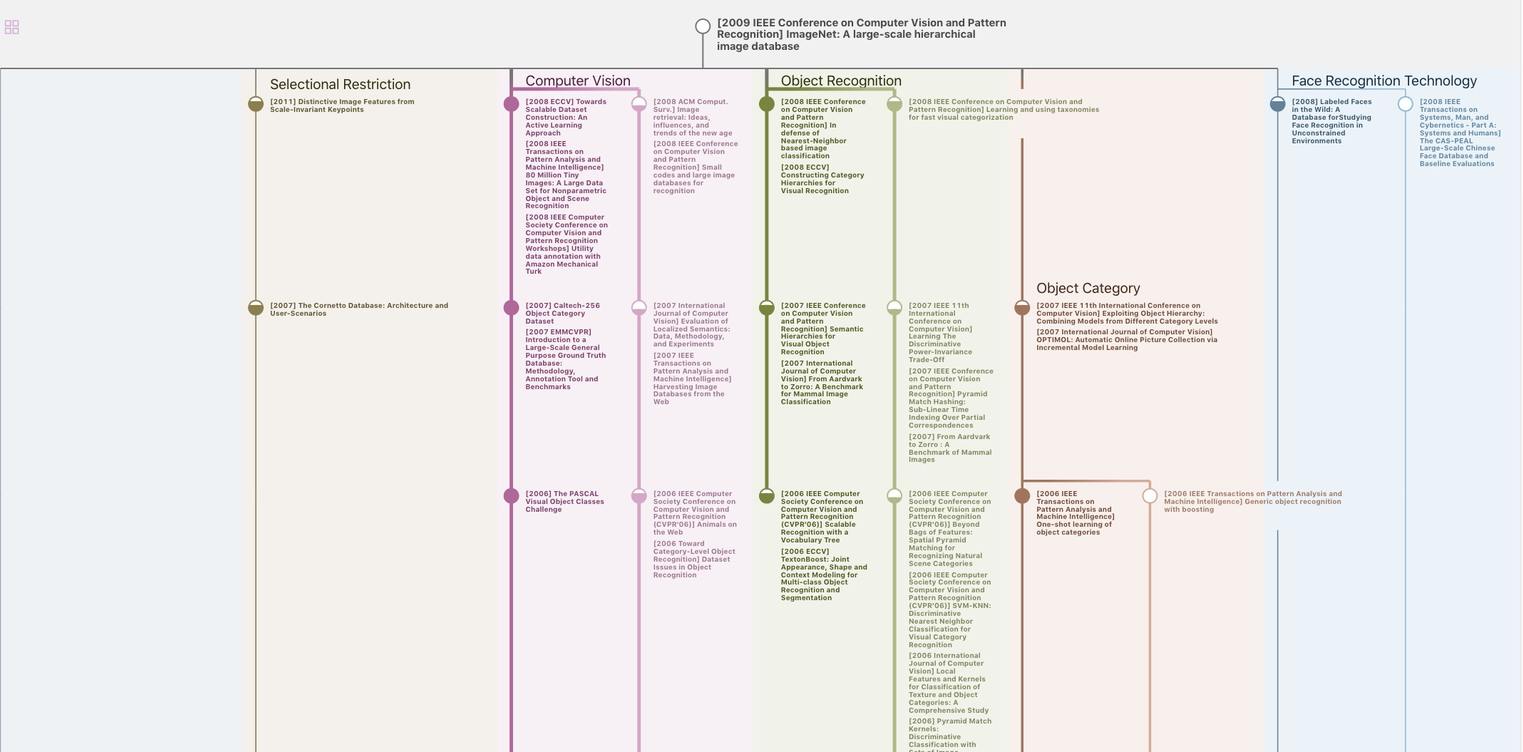
生成溯源树,研究论文发展脉络
Chat Paper
正在生成论文摘要