Adversarial Machine Learning In The Physical Domain
JOHNS HOPKINS APL TECHNICAL DIGEST(2021)
摘要
With deep neural networks (DNNs) being used increasingly in many applications, it is critical to improve our understanding of their failure modes and potential mitigations. A Johns Hopkins University Applied Physics Laboratory (APL) team successfully inserted a backdoor (train-time attack) into a common object detection model. In conjunction with this research, they developed a principled methodology to evaluate patch attacks (test-time attacks) and the factors impacting their success. Their approach enabled the creation of a novel optimization framework for the first-ever design of semitransparent patches that can overcome scale limitations while retaining desirable factors with regard to deployment and detectability.
更多查看译文
AI 理解论文
溯源树
样例
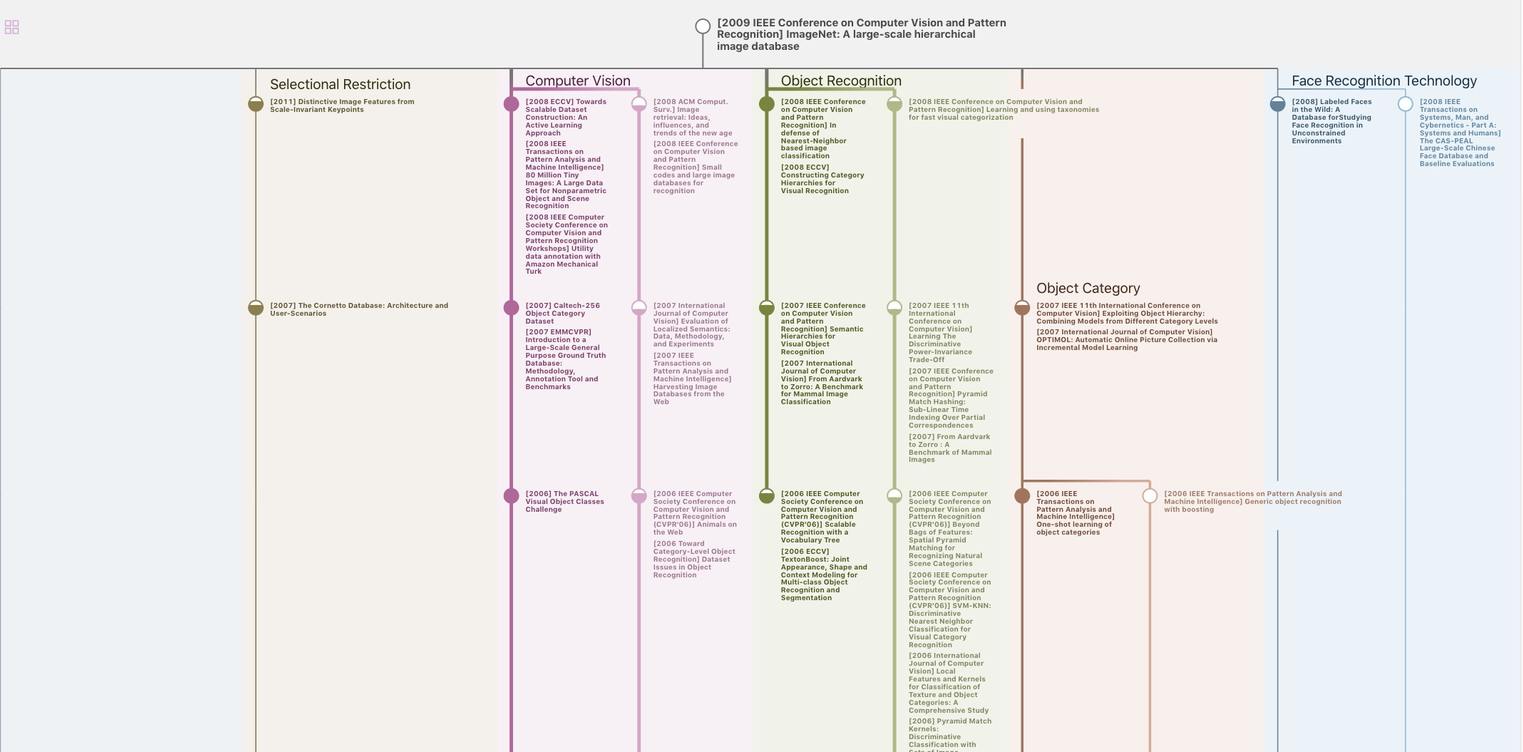
生成溯源树,研究论文发展脉络
Chat Paper
正在生成论文摘要