Forecasting Short-Term Electricity Load Using Hybrid Support Vector Regression With Grey Catastrophe And Random Forest Modeling
UTILITIES POLICY(2021)
摘要
This paper develops a novel short-term load forecasting model that hybridizes several machine learning methods, such as support vector regression (SVR), grey catastrophe (GC (1,1)), and random forest (RF) modeling. The modeling process is based on the minimization of both SVR and risk. GC is used to process and extract catastrophe points in the long term to reduce randomness. RF is used to optimize forecasting performance by exploiting its superior optimization capability. The proposed SVR-GC-RF model has higher forecasting accuracy (MAPE values are 6.35% and 6.21%, respectively) using electric loads from Australian-Energy-Market-Operator; it can provide analytical support to forecast electricity consumption accurately.
更多查看译文
关键词
Support vector regression (SVR), Grey catastrophe (GC), Random forest (RF), Short term load forecasting
AI 理解论文
溯源树
样例
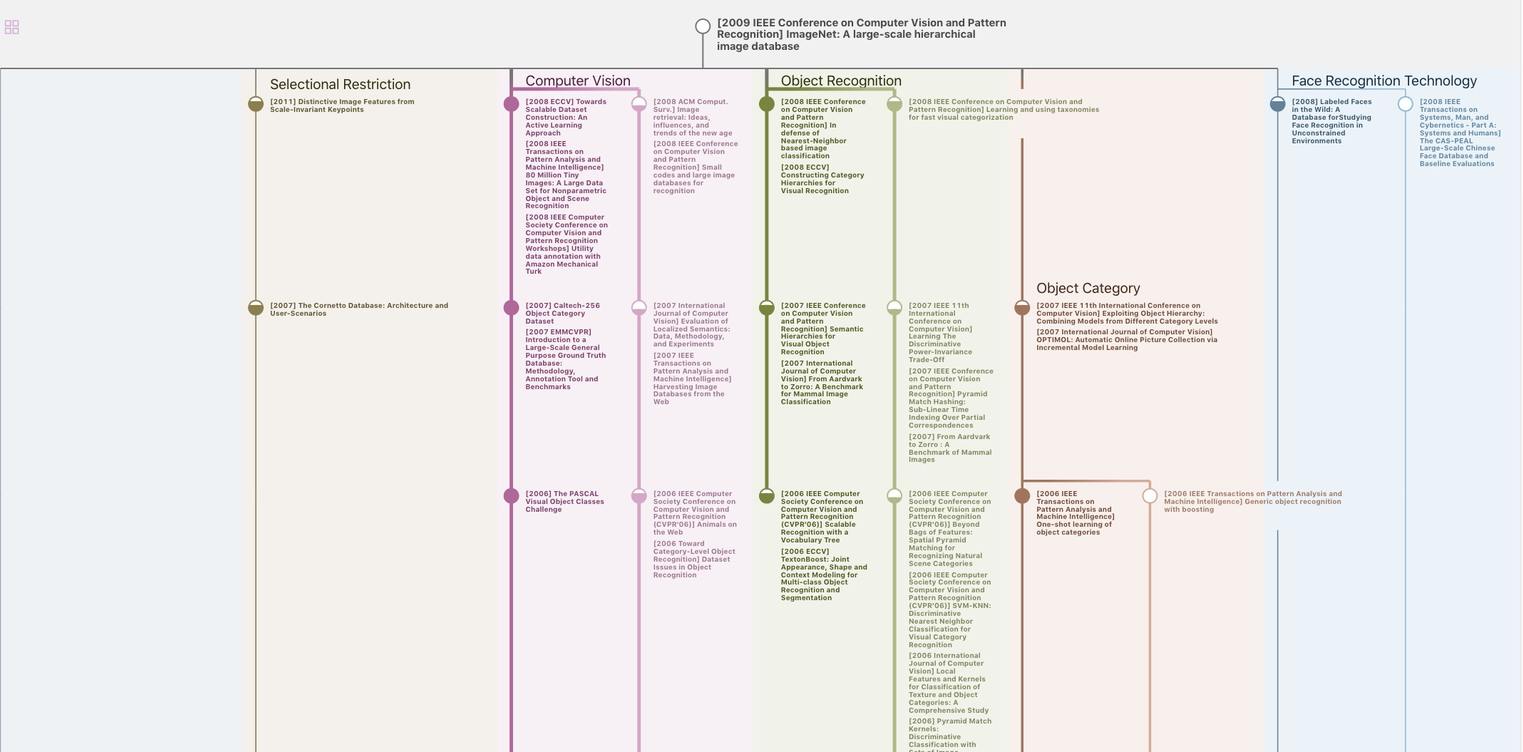
生成溯源树,研究论文发展脉络
Chat Paper
正在生成论文摘要