CNN based Anthropomorphic Model Observer for Defect Localization
MEDICAL IMAGING 2021: IMAGE PERCEPTION, OBSERVER PERFORMANCE, AND TECHNOLOGY ASSESSMENT(2021)
摘要
Model Observers (MO) are algorithms designed to evaluate and optimize the parameters of newly developed medical imaging technologies by providing a measure of human accuracy for a given diagnostic task. If designed well, these algorithms can expedite and reduce the expenses of coordinating sessions with radiologists to evaluate the diagnosis potential of such reconstruction technologies. During the last decade, classic machine learning techniques along with feature engineering have proved to be a good MO choice by allowing the models to be trained to detect or localize defects and therefore potentially reduce the extent of needed human observer studies. More recently, and with the developments in computer processing speed and capabilities, Convolutional Neural Networks (CNN) have been introduced as MOs eliminating the need of feature engineering. In this paper, we design, train and evaluate the accuracy of a fully convolutional U-Net structure as a MO for a defect forced-localization task in simulated images. This work focuses on the optimization of parameters, hyperparameters and choice of objective functions for CNN model training. Results are shown in the form of human accuracy vs model accuracy as well as efficiencies with respect to the ideal observer, and reveal a strong agreement between the human and the MO for the chosen defect localization task.
更多查看译文
关键词
Model observer,medical image quality assessment,machine learning,deep learning,CNN,U-Net
AI 理解论文
溯源树
样例
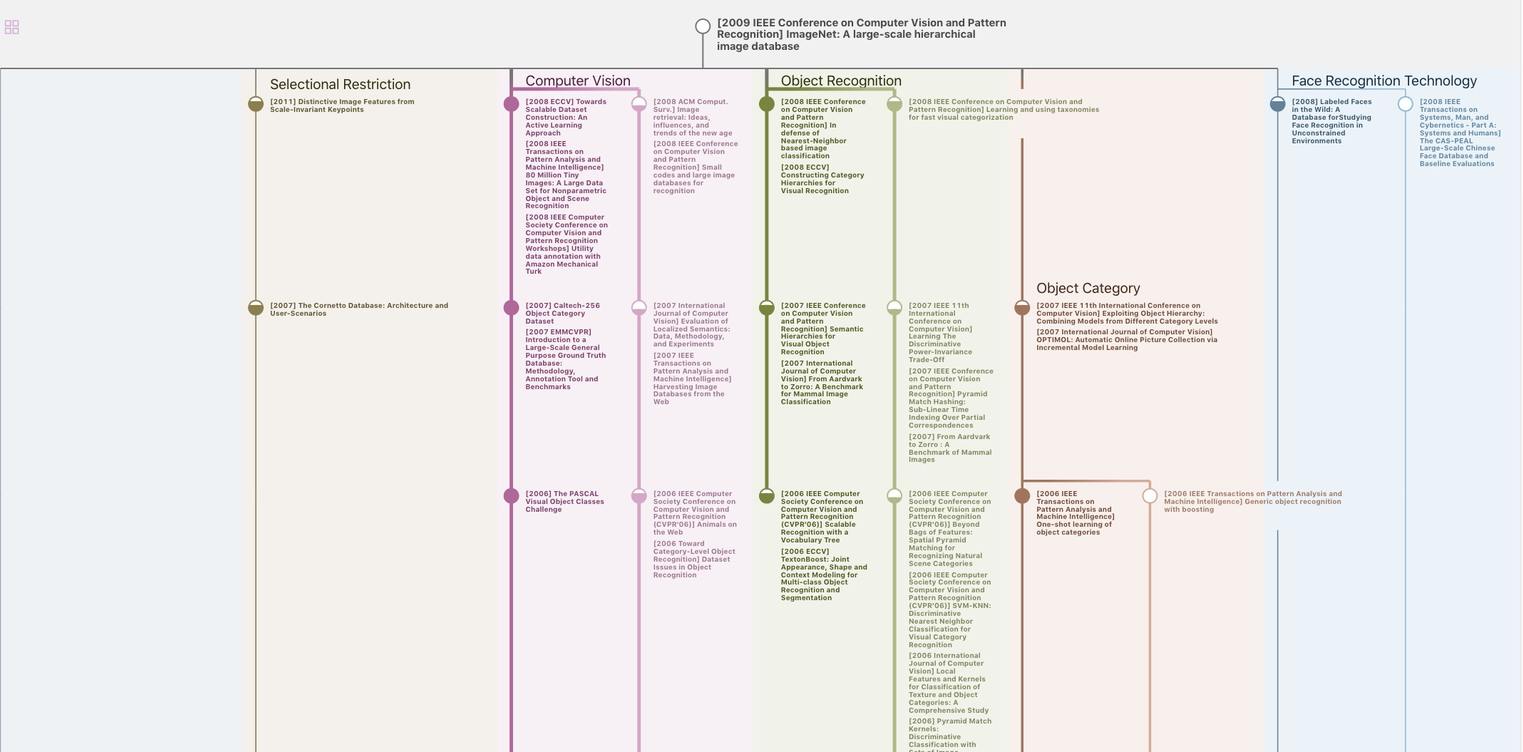
生成溯源树,研究论文发展脉络
Chat Paper
正在生成论文摘要