The systematic development of attributes and levels for a discrete choice experiment of HIV patient preferences for long-acting antiretroviral therapies in the United States
MEDICAL DECISION MAKING(2022)
摘要
Introduction Patient preferences for long-acting antiretroviral therapies (LA-ART) should inform development of regimens with optimal adherence and acceptability. We describe a systematic process used to identify attributes and levels for a discrete choice experiment (DCE) designed to elicit preferences for potential LA-ART options in the US. Methods Our approach was conducted in four stages: data collection, data reduction, removing inappropriate attributes, and optimizing wording. We started with 8 attributes defining potential LA-ART products based on existing literature and knowledge of products in development. We conducted 12 key informant interviews with experts in HIV treatment. The list of attributes, the set of plausible levels for each attribute, and restrictions on combinations of attribute levels were updated iteratively. Results Despite uncertainty about which products will become available, key informant discussions converged on 4 delivery modes (infusions and patches were not considered immediately feasible) and 6 additional attributes. Treatment effectiveness and frequency of clinical monitoring were dropped. Oral lead-in therapy was split into two attributes: pre-treatment time undetectable and pre-treatment negative reaction testing. We omitted product-specific systemic and local side effects. In addition to mode, the final set of attributes included: frequency of dosing; location of treatment; pain; pre-treatment time undetectable; pre-treatment negative reaction testing; and late-dose leeway. Conclusions A systematic process successfully captured elements that are both feasible and relevant to evaluating the acceptability of potential LA-ART alternatives to patients.
更多查看译文
关键词
hiv patient preferences,antiretroviral therapies,discrete choice experiment,long-acting
AI 理解论文
溯源树
样例
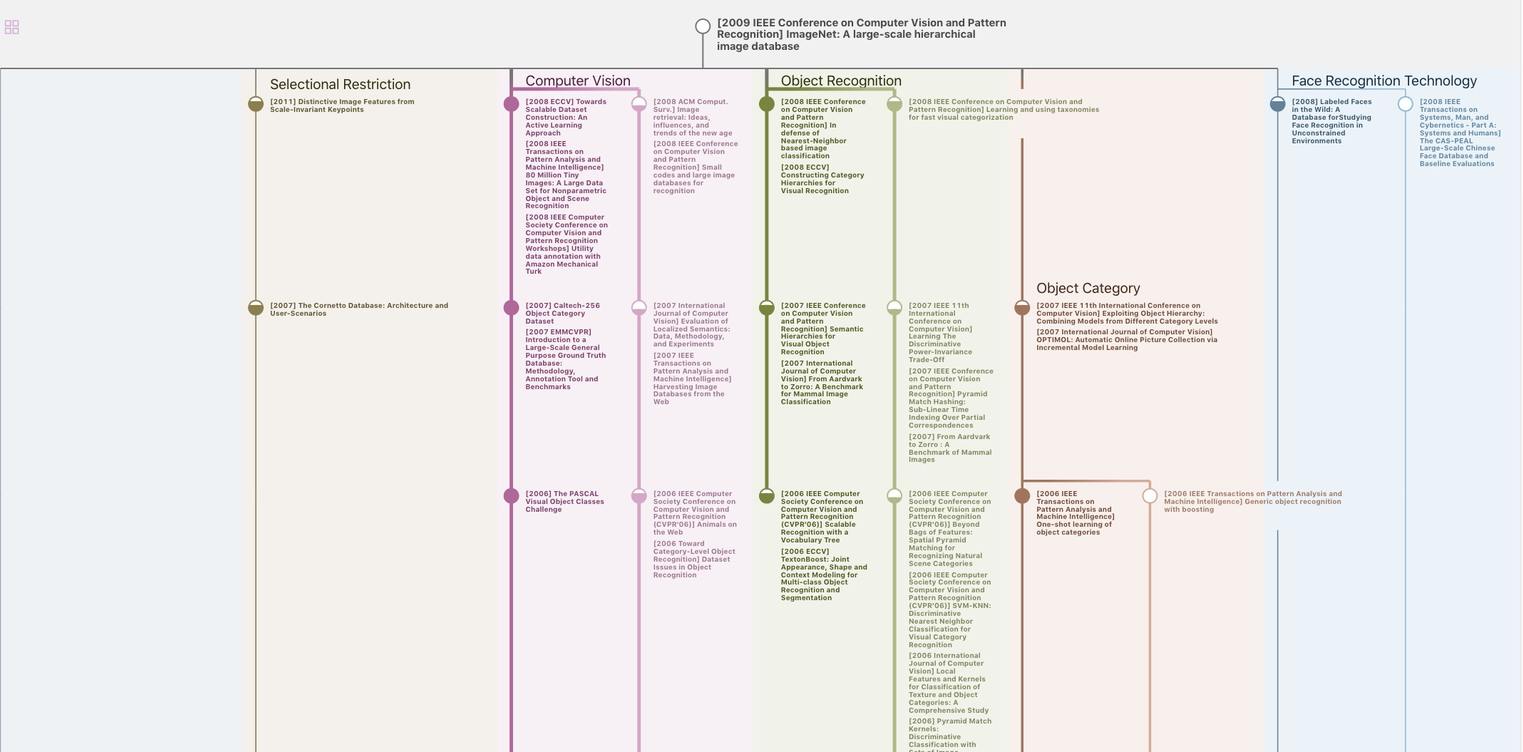
生成溯源树,研究论文发展脉络
Chat Paper
正在生成论文摘要