Hamiltonian Monte Carlo for Simultaneous Interface and Spatial Field Detection (HMCSISFD) and its application to a piping zone interface detection problem
INTERNATIONAL JOURNAL FOR NUMERICAL AND ANALYTICAL METHODS IN GEOMECHANICS(2021)
摘要
In practice, inverse problems related to explicit interface detection rely on scarcely available information of the background continuous spatial fields. To accurately carry on inversion in such cases, a method called Hamiltonian Monte Carlo for Simultaneous Interface and Spatial Field Detection (HMCSISFD) is developed. Update of the interface parameters is done in a reversible mesh moving framework leading to a change in domain geometry which forces a recomputation of the spatial field covariance function as it is domain dependent. The eigenvalue problem and its derivatives w.r.t. the parameters are solved again on the updated domain to enable dimensionality reduction of the spatial field parameters through the use of the truncated Karhunen-Loeve expansion. This simultaneous parameter update procedure is applied to the detection of the interface and spatial field properties of a piping zone. Inversion is done considering hydraulic head and discharge rate data, with a priori known observation noise, from a carefully designed laboratory seepage flow experiment in a domain consisting a predefined piping zone. The static pipe in the experiment is representative of a pipe in equilibrium beneath an embankment/levee where the current hydraulic gradient isn't sufficient to cause further pipe progression. Markov chains obtained from the HMCSISFD method show convergence and the true interface and spatial field parameters are found to lie well within the 95% high probability density regions and the 0.05 and 0.95 quantiles, respectively, provided a proper choice for the observation noise is made.
更多查看译文
关键词
Hamiltonian Monte Carlo, interface detection, inverse problems, piping, probabilistic methods, spatial field estimation
AI 理解论文
溯源树
样例
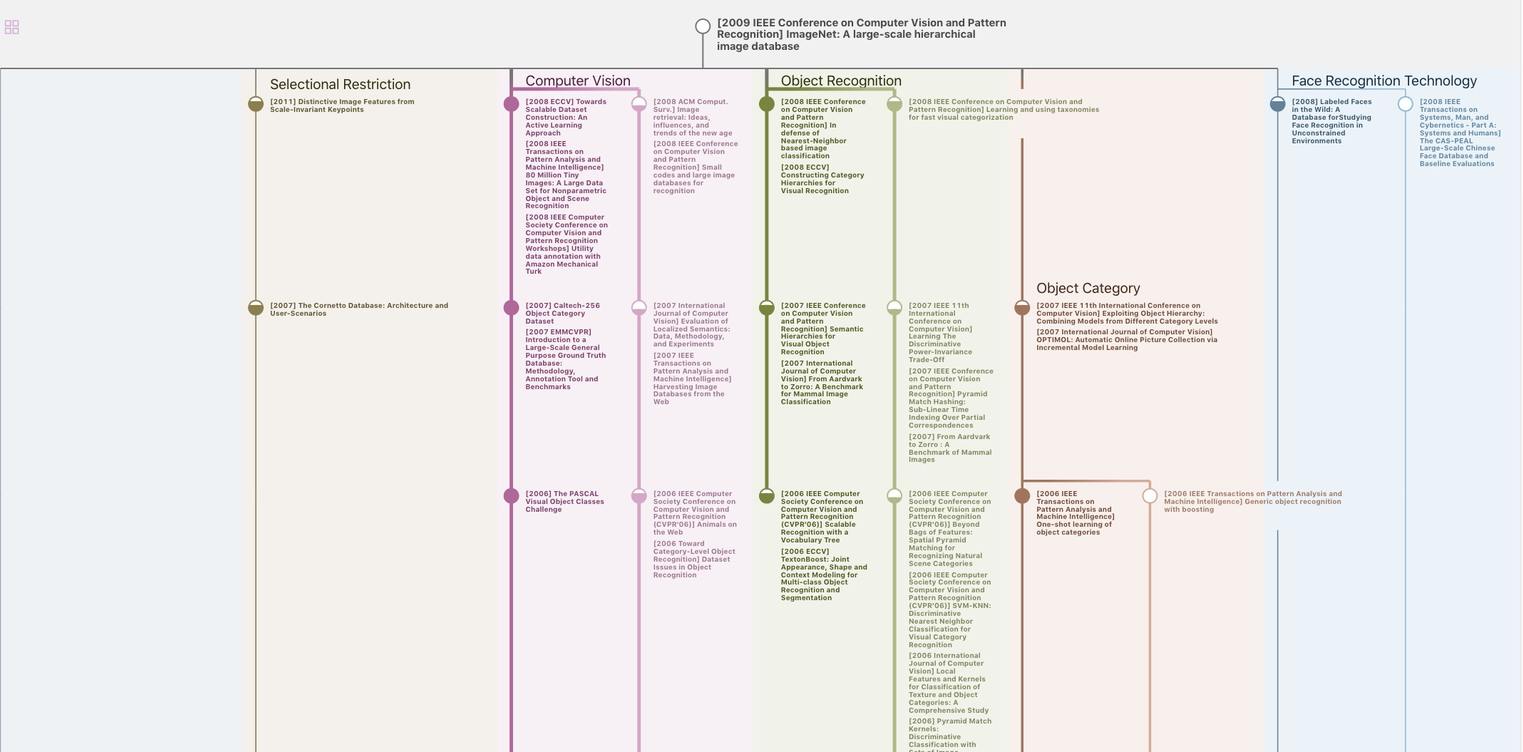
生成溯源树,研究论文发展脉络
Chat Paper
正在生成论文摘要