Productivity Modeling Enhancement of a Solar Desalination Unit with Nanofluids Using Machine Learning Algorithms Integrated with Bayesian Optimization
Energy technology(2021)
摘要
Herein, double slope solar still (DSSS) performance is accurately forecast with the aid of four different machine learning (ML) models, namely, artificial neural network (ANN), random forest (RF), support vector regression (SVR), and linear SVR. Furthermore, the tuning of ML models is optimized using the Bayesian optimization algorithm (BOA) to get the optimal performance of all models and identify the best predictive one. All the models are trained, tested, and validated depending on experimental data acquired under Egyptian climatic conditions. The results reveal that ML models can be a powerful tool to forecast DSSS performance. Among them, RF is the most potent ML model obtaining the highest determination coefficient (R2) and the lowest absolute error percentage of 0.997% and 2.95%, respectively. Furthermore, the experimental results also show that the mean value of accumulated (daily) freshwater productivity from DSSS is 4.3 L m−2.
更多查看译文
关键词
artificial neural network,Bayesian optimization,Ddouble slope solar stills,machine learning,nanofluids,random forest
AI 理解论文
溯源树
样例
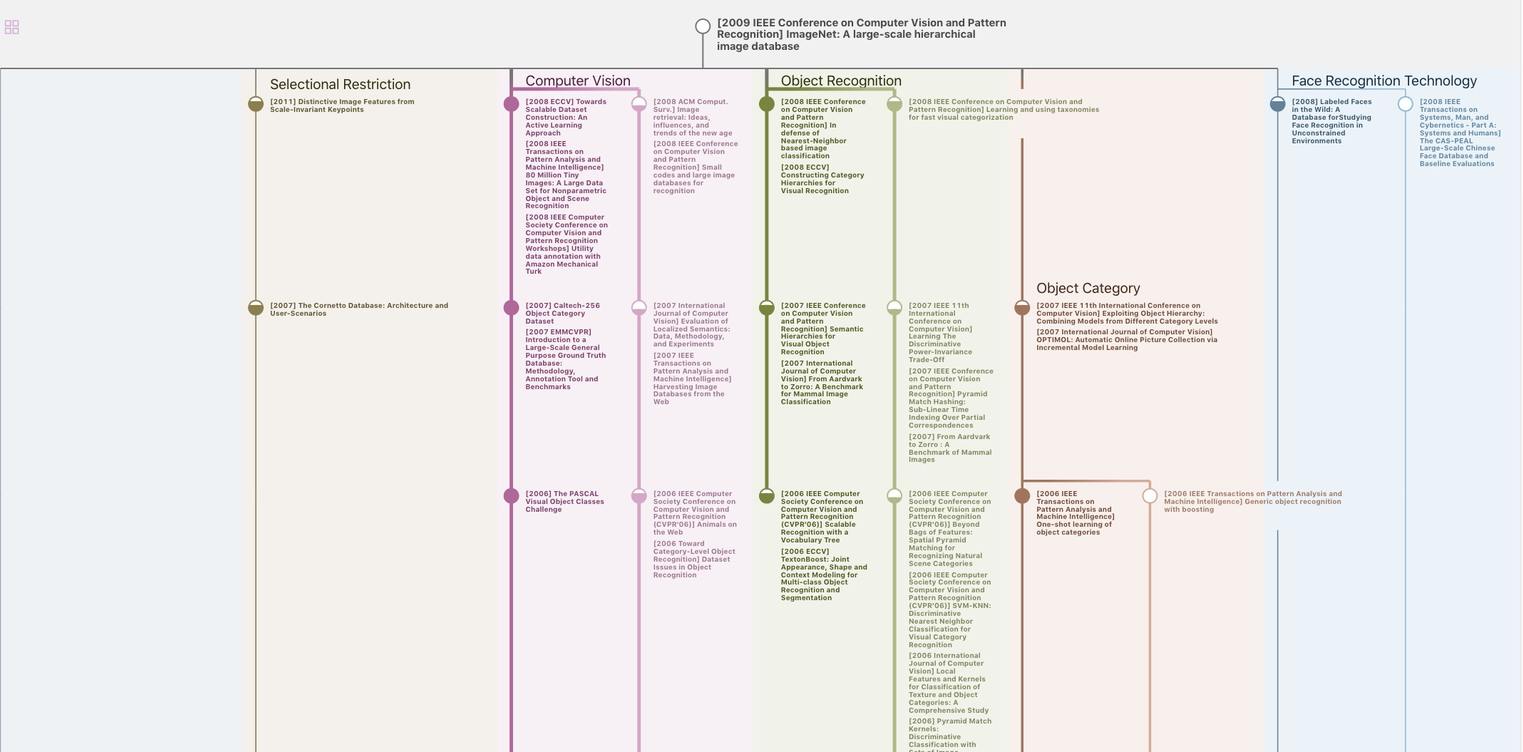
生成溯源树,研究论文发展脉络
Chat Paper
正在生成论文摘要