Attending From Foresight: A Novel Attention Mechanism For Neural Machine Translation
IEEE-ACM TRANSACTIONS ON AUDIO SPEECH AND LANGUAGE PROCESSING(2021)
摘要
Machines translation (MT) is an essential task in natural language processing or even in artificial intelligence. Statistical machine translation has been the dominant approach to MT for decades, but recently neural machine translation achieves increasing interest because of its appealing model architecture and impressive translation performance. In neural machine translation, an attention model is used to identify the aligned source words for the next target word, i.e., target foresight word, to select translation context. However, it does not make use of any information about this target foresight word at all. Previous work proposed an approach to improve the attention model by explicitly accessing this target foresight word and demonstrating substantial alignment tasks. However, this approach cannot be applied in machine translation tasks where the target foresight word is unavailable. This paper proposes several novel enhanced attention models by introducing hidden information (such as part-of-speech) of the target foresight word for the translation task. We incorporate the novel enhanced attention employing hidden information about the target foresight word into both recurrent and self-attention-based neural translation models and theoretically justify that such hidden information can make translation prediction easier. Empirical experiments on four datasets further verify that the proposed attention models deliver significant improvements in translation quality.
更多查看译文
关键词
Predictive models, Machine translation, Task analysis, Context modeling, Recurrent neural networks, Decoding, Unemployment, NMT, Attention, Word Alignment
AI 理解论文
溯源树
样例
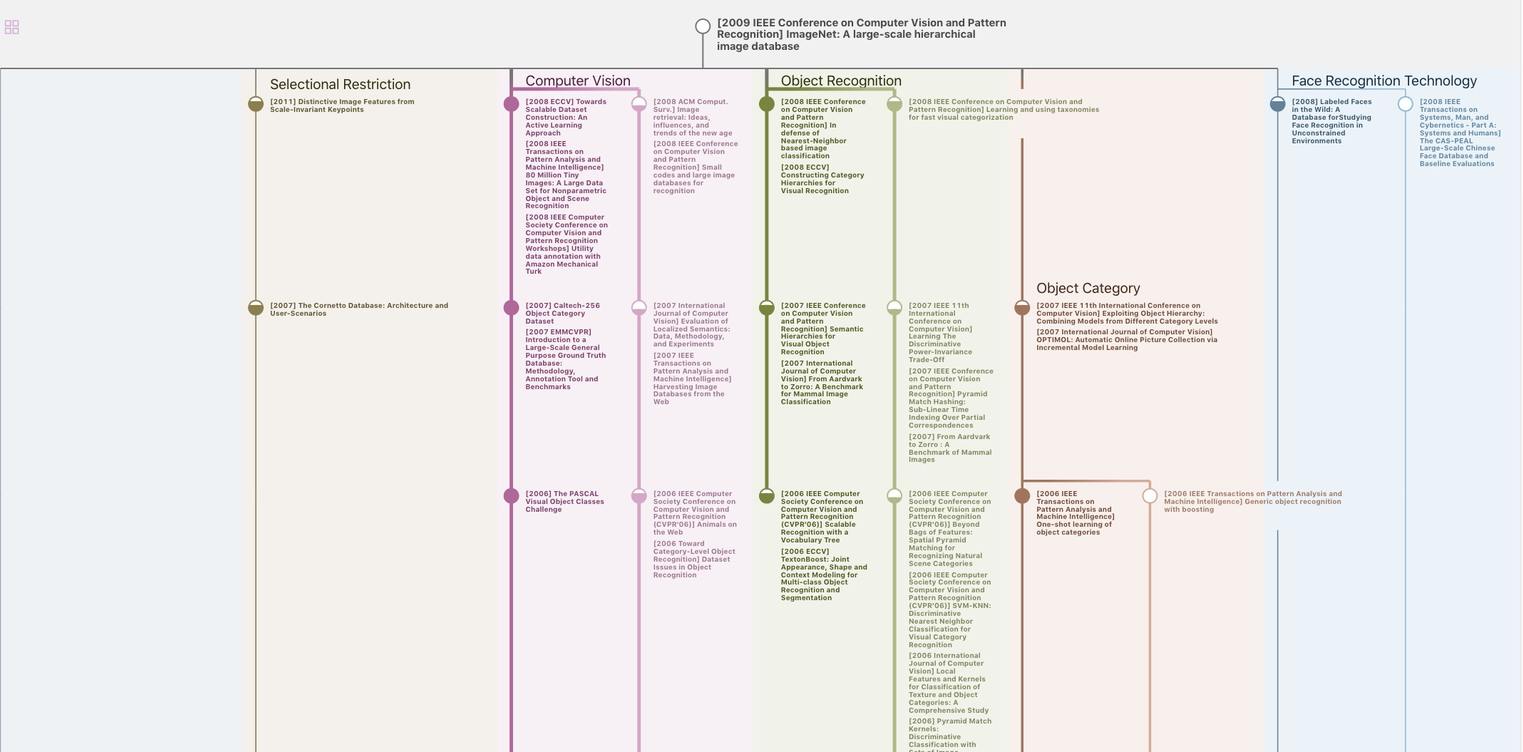
生成溯源树,研究论文发展脉络
Chat Paper
正在生成论文摘要