Learning Individual Class Representation From Biased Multi-Label Data
IEEE ACCESS(2021)
摘要
Image recognition is a popular and important research field of computer vision. Recently with the development of deep learning technology, image recognition performance has been improved significantly. However with multi-label images, recognizing individual category is a challenging task. In order to address the problem, we propose a Feature Disintegrator (FD) that decomposes co-occurred instance features of multi-label into individual categories. In experimental evaluation, proposed method achieves the gains of mean average precision (mAP) up to 18.67% and 29.05% over baseline networks in ML-MNIST and ML-CIFAR, respectively. It shows 5.76% and 6.65% higher mAP than baseline on PASCAL VOC-2007 and MS-COCO data set respectively.
更多查看译文
关键词
Training, Image recognition, Task analysis, Toy manufacturing industry, Correlation, Training data, Semantics, Computer vision, deep learning, supervised learning, image classification, image recognition
AI 理解论文
溯源树
样例
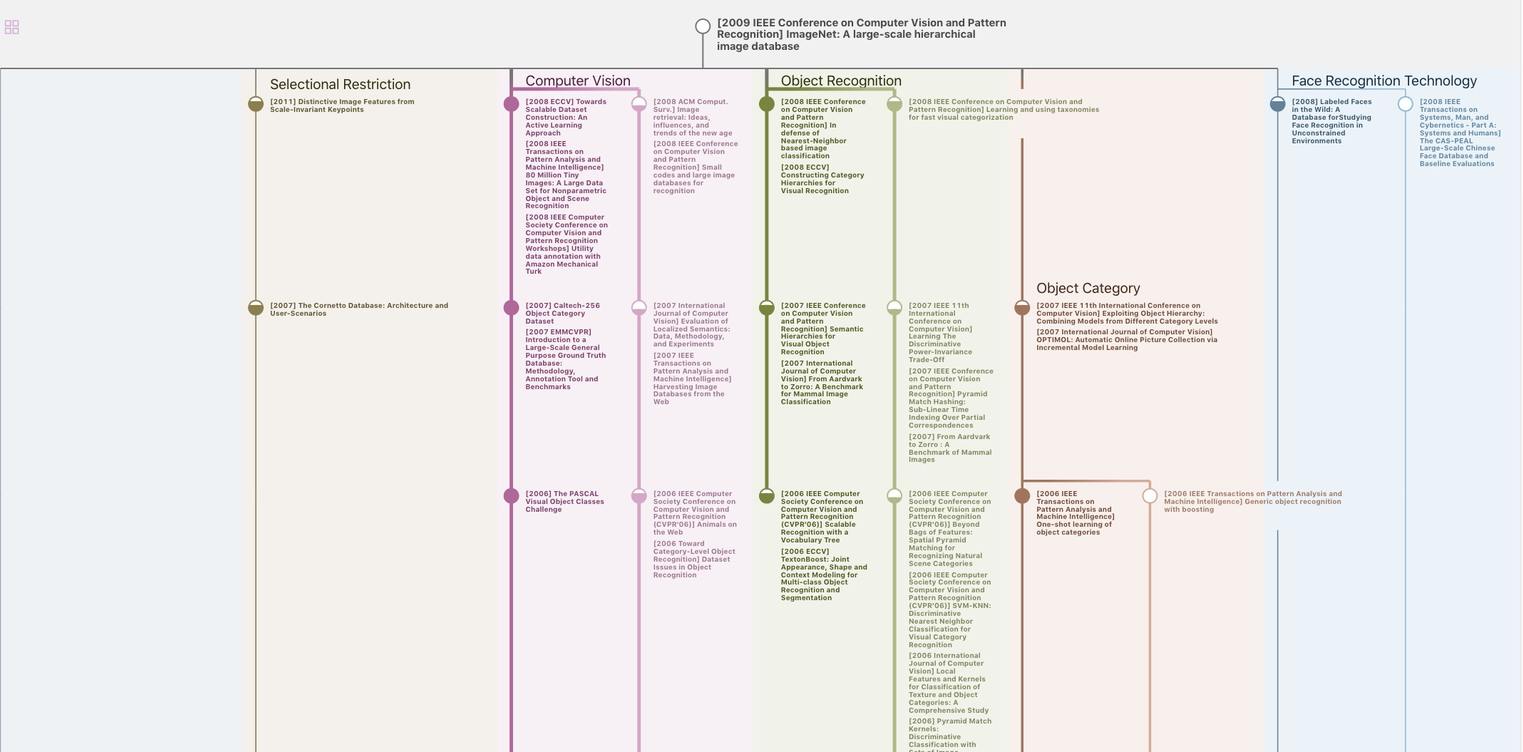
生成溯源树,研究论文发展脉络
Chat Paper
正在生成论文摘要