Deep Learning-Based High Throughput Inspection In 3d Nanofabrication And Defect Reversal In Nanopillar Arrays: Implications For Next Generation Transistors
ACS APPLIED NANO MATERIALS(2021)
摘要
Densely packed high-aspect-ratio (HAR) nanostructures are the core elements of future microelectronics components. Manufacturing these nanostructures for device applications requires multiple fabrication steps involving wet processes, followed by a drying step. During drying, these nanostructures experience strong capillary forces that induce their bending and cause them to permanently stick to their neighbors, a phenomenon often referred to as pattern collapse. The pattern collapse and the difficulty in reliably identifying damaged nanostructures pose a critical challenge for the fabrication of HAR devices. Here, we developed a machine learning-based approach to identify collapsed nanostructures from a large patterned array of vertical Si nanopillars with 99.84% accuracy. Furthermore, we show that the pattern collapse can be reversed by selectively etching the native surface SiO2 layer of the nanopillars at their adhesions. Our approach for accurate and rapid identification of the collapsed nanostructures combined with the method to reverse this damage provides a versatile platform for developing high-yield fabrication processes for nanoscale semiconductor devices.
更多查看译文
关键词
liquid-phase TEM, machine learning, nanofabrication, nanostructures, pattern collapse
AI 理解论文
溯源树
样例
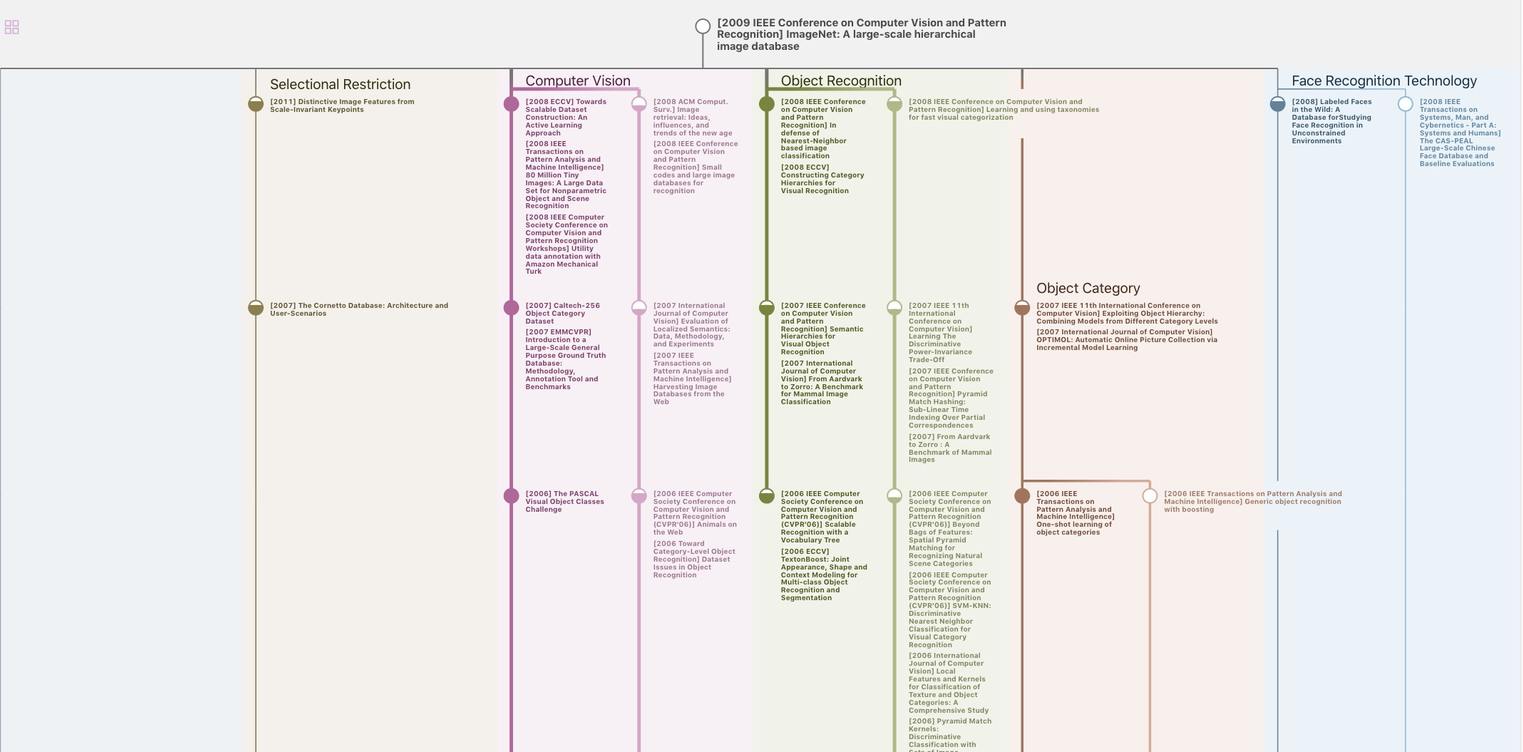
生成溯源树,研究论文发展脉络
Chat Paper
正在生成论文摘要