Classification Of Microscopic Laser Engraving Surface Defect Images Based On Transfer Learning Method
ELECTRONICS(2021)
摘要
Microscopic laser engraving surface defect classification plays an important role in the industrial quality inspection field. The key challenges of accurate surface defect classification are the complete description of the defect and the correct distinction into categories in the feature space. Traditional classification methods focus on the terms of feature extraction and independent classification; therefore, feed handcrafted features may result in useful feature loss. In recent years, convolutional neural networks (CNNs) have achieved excellent results in image classification tasks with the development of deep learning. Deep convolutional networks integrate feature extraction and classification into self-learning, but require large datasets. The training datasets for microscopic laser engraving image classification are small; therefore, we used pre-trained CNN models and applied two fine-tuning strategies. Transfer learning proved to perform well even on small future datasets. The proposed method was evaluated on the datasets consisting of 1986 laser engraving images captured by a metallographic microscope and annotated by experienced staff. Because handcrafted features were not used, our method is more robust and achieves better results than traditional classification methods. Under five-fold-validation, the average accuracy of the best model based on DenseNet121 is 96.72%.
更多查看译文
关键词
microscopic laser engraving surface, defect classification, deep learning technology
AI 理解论文
溯源树
样例
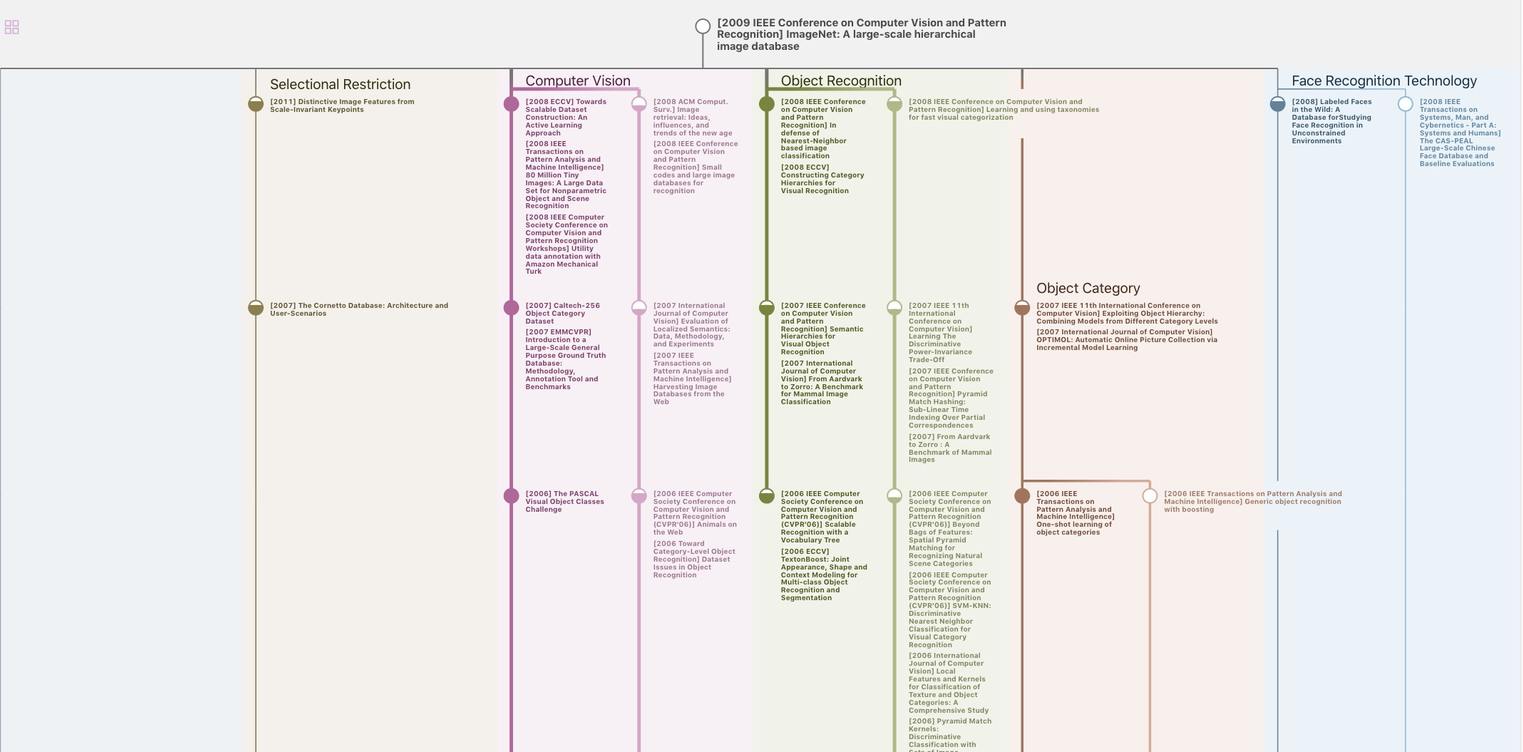
生成溯源树,研究论文发展脉络
Chat Paper
正在生成论文摘要