A Machine Learning Approach To Detect Ffpe Artefacts Leads To Accurate Estimation Of Tumor Mutational Burden From Sequencing Data Without A Matched Normal.
CANCER RESEARCH(2021)
摘要
Abstract Tumor mutational burden (TMB) is emerging as a promising predictive biomarker to select cancer patients who will benefit from immunotherapy. However, accurate TMB estimation from sequencing data remains hampered by the ability to correctly distinguish somatic variants from both germline variants and spurious base changes introduced by sample preparation and sequencing processes. The latter is particularly prevalent in poor quality FFPE material where degradation and chemical modifications of the DNA manifest as variant artefacts leading to over-estimation of TMB. One method of removing germline variants and artefacts is to compare tumor variant calls to those obtained from matched normal tissue, however inclusion of a matched normal adds significant logistical and financial challenges to clinical trial assays. Therefore, there remains a critical need to establish alternative approaches enabling accurate TMB estimation from FFPE tumor-only samples. To address these challenges, we have developed a tumor-only somatic variant calling pipeline for accurate determination of TMB from FFPE material profiled by whole exome sequencing (WES). The process is underpinned by two key features (a) enhanced germline variant filtering that uses a systematic rule-based strategy, (b) FFPE artefact removal using an ensemble machine learning model incorporating multiple variant call QC parameters. The germline filtering approach was developed on a matched fresh frozen (FF)/FFPE/blood dataset of 23 cancer patients. The FFPE artefact filtering model was built on a dataset of 59 FF and FFPE matched pairs, where FFPE artefacts were defined as variants present in FFPE but absent in the FF sample. Benchmarked against internal and TCGA datasets of somatic variant calls from >30 FF samples with a matched normal, our germline and FFPE filtering steps decreased false discovery rates by over 20%. This translated into improved TMB scoring performance with tumor-only pipeline estimates from three SeraCare reference standards closely matching expected TMB scores. Critically, we establish the clinical relevance of our approach on a published cohort of 98 patients treated with an immune checkpoint inhibitor. Here we observed increased association between patient response and TMB estimated from our pipeline (p=0.042) compared to a standard pipeline with no enhanced germline or FFPE artefact filters (p=0.56). Overall, our combined germline and FFPE artefact filtering pipeline results in significantly improved somatic variant detection and TMB scoring performance in FFPE samples where a matched normal is unavailable. It is applicable across multiple disease types and adaptable to other platforms. Therefore, the pipeline has utility in clinical laboratories where accurate somatic variant detection is crucial to successful deployment of predictive biomarkers such as TMB. Citation Format: Hui Sun Leong, Emma O'Connor, Nuala McCabe, Sinead Donegan, Steven M. Walker, Jayna Mistry, Karen Keating, Denis P. Harkin, Richard D. Kennedy, Laura A. Knight, James R. Bradford. A machine learning approach to detect FFPE artefacts leads to accurate estimation of tumor mutational burden from sequencing data without a matched normal [abstract]. In: Proceedings of the American Association for Cancer Research Annual Meeting 2021; 2021 Apr 10-15 and May 17-21. Philadelphia (PA): AACR; Cancer Res 2021;81(13_Suppl):Abstract nr 152.
更多查看译文
AI 理解论文
溯源树
样例
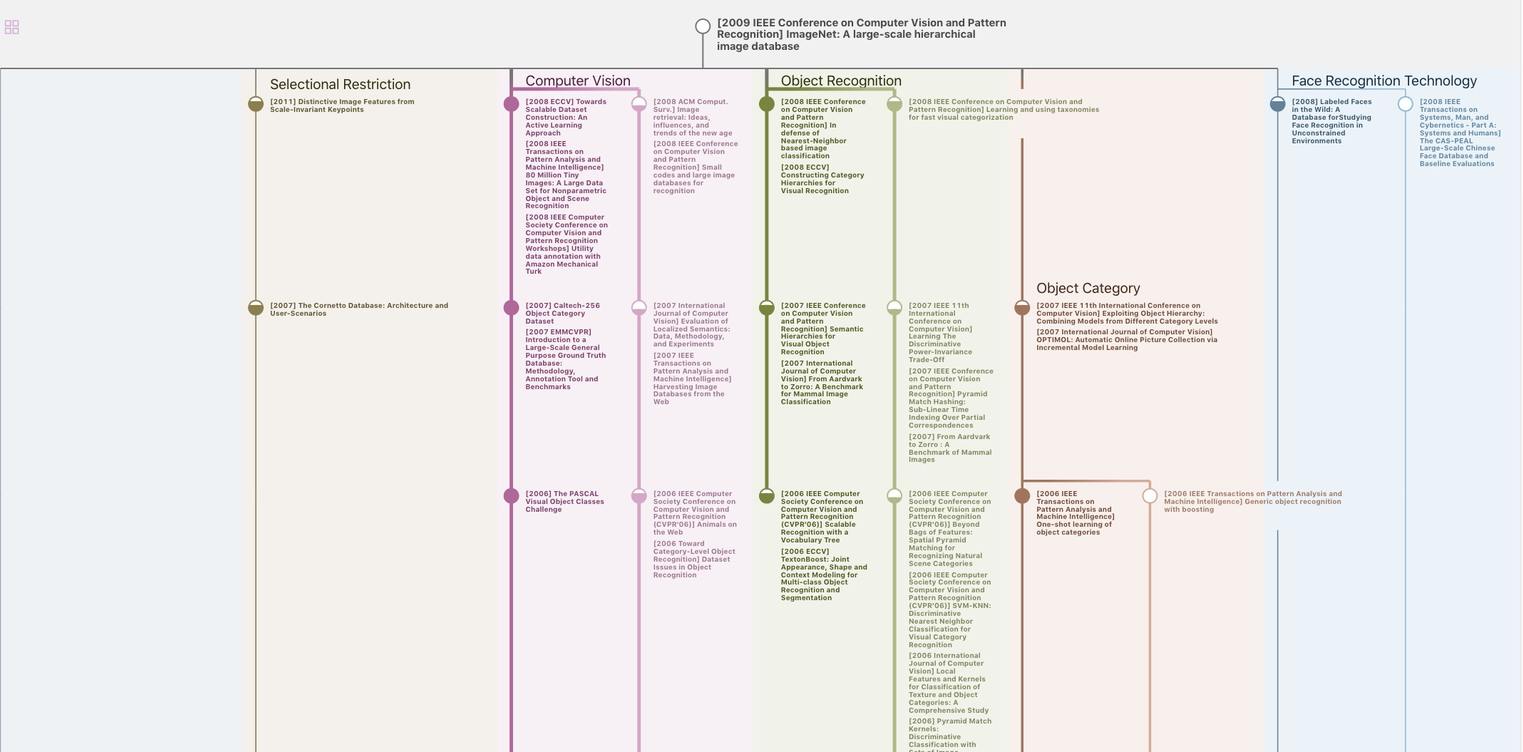
生成溯源树,研究论文发展脉络
Chat Paper
正在生成论文摘要