Patterns And Regulation Of Post Translational Modifications In Cancer.
CANCER RESEARCH(2021)
摘要
Abstract Post-translational modifications (PTMs) (e.g., ubiquitylation, phosphorylation, acetylation) have been studied for their key role in cell signaling and in regulating cell physiology. Advances in mass spectrometry now enable measuring PTMs and studying their role and prevalence in cancer. Although most studies have focused on a single PTM and its impact on downstream signaling in a single cancer type, understanding PTM-driven commonalities, disparities, and their crosstalk across cancer types will be critical in understanding fundamental oncogenic principles governed by PTMs. Thus, we aim to understand the underlying patterns of PTMs in molecular signaling pathways shared across multiple cancer types by studying changes in protein acetylation and phosphorylation within the largest collection of in-depth genomic, transcriptomic, proteomic, and PTM profiles compiled from 11 cancer types generated across the CPTAC projects.We used matrix factorization methods to extract expression signatures jointly from transcriptomic, proteomic, and PTM data across multiple cancer types. Preliminary analysis of 6 tumor types identified 18 pan-cancer, multi-omic expression signatures. Characterization of these signatures using ranked gene-set enrichment analysis (GSEA) and site-specific PTM signature enrichment analysis (PTM-SEA) highlighted pathways including DNA damage response, immune inactivation/deactivation, tumor invasiveness, and metabolic pathways. To investigate the effect of driver alterations on PPIs, we (i) compute rank correlations of pan-cancer mutational signatures to both expression levels and our multi-omic expression signatures, and (ii) map PTMs and mutations to available crystal structures. We additionally investigate mutations that recur across cancer types (e.g., TP53, PIK3CA, CTNNB1) and impact expression profiles of downstream PTMs. We further connect pan-cancer phosphoproteomic data to a library of biochemical specificities of the human kinome using a novel, unbiased computational platform that builds on extensive and experimentally validated kinase-substrate relationships. We identified activated and deactivated kinases/acetylases based on their altered PTM sites within and across the multi-omic subtypes and compared the effects on different targets. We also characterize the crosstalk between acetylation and phosphorylation across cancer, and, systematically identify acetylation events that prime and enhance kinase activity. Finally, we define kinases, acetylases, and PTM outliers as potential druggable targets (using The Drug Gene Interaction Database and DepMap). Overall, this study presents the landscape of PTMs across cancer and will serve as a community resource to promote a deeper understanding of PTM-governed processes leading to cancer progression, with the potential to improve current therapies or help design new treatment approaches against cancer. Citation Format: Yifat Geffen, Shankara Anand, Yo Akiyama, Tommer M. Yaron, Alexander Kerelsky, Jared L. Johnson, Karsten Krug, David Heiman, Shankha Satpathy, Karl Clauser, Michael Gillette, D. R. Mani, Chet Birger, Steven Carr, Lewis C. Cantley, Francois Aguet, Gad Getz, Oncogenic Drivers and Pathways Group, Clinical Proteomic Tumor Analysis Consortium (CPTAC). Patterns and regulation of post translational modifications in cancer [abstract]. In: Proceedings of the American Association for Cancer Research Annual Meeting 2021; 2021 Apr 10-15 and May 17-21. Philadelphia (PA): AACR; Cancer Res 2021;81(13_Suppl):Abstract nr 16.
更多查看译文
关键词
mRNA modification,Pathway Analysis
AI 理解论文
溯源树
样例
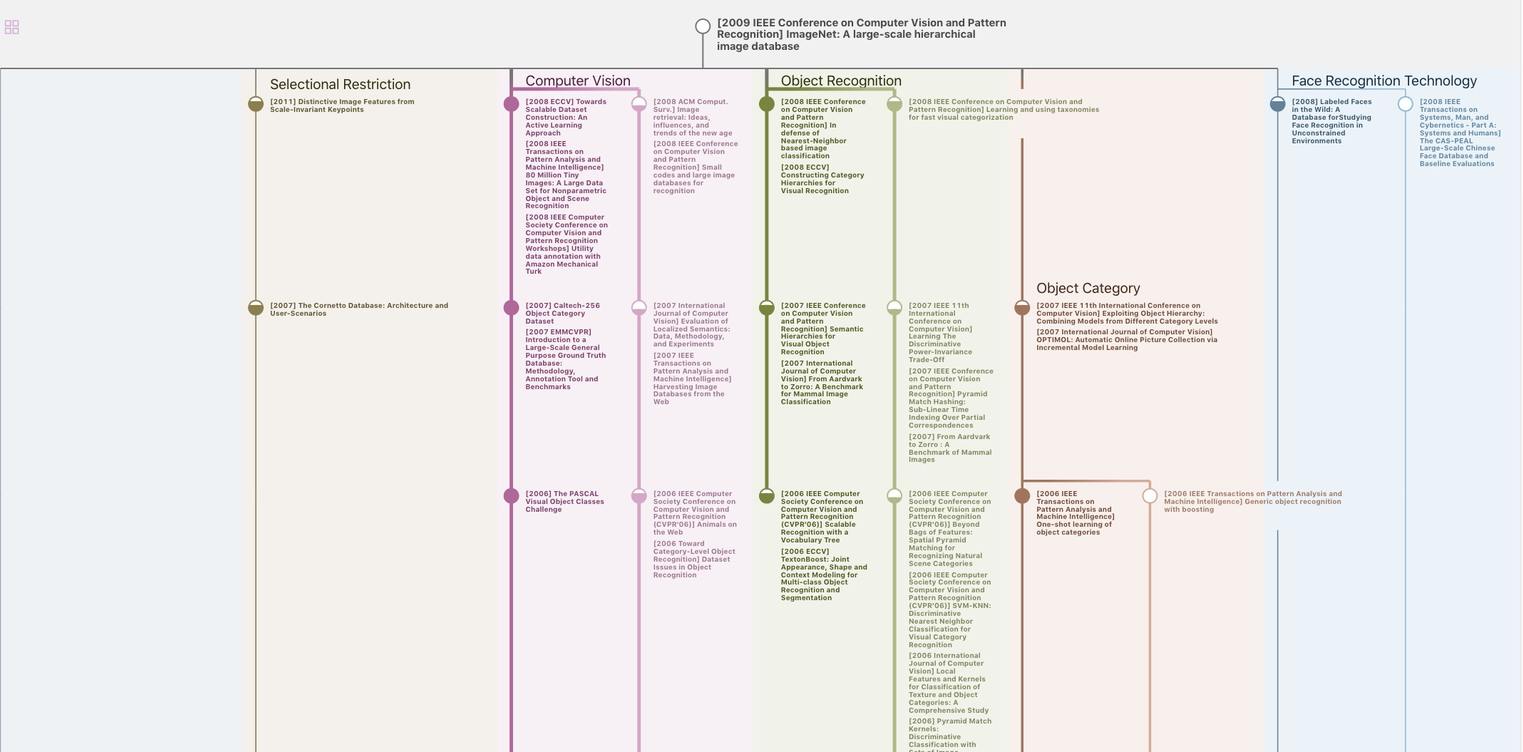
生成溯源树,研究论文发展脉络
Chat Paper
正在生成论文摘要