Shape-Supervised Super-Resolution Convolutional Neural Network for Melt Droplet Images
MICROGRAVITY SCIENCE AND TECHNOLOGY(2021)
摘要
The containerless method is generally used to study the intrinsic properties of materials, especially the thermophysical properties of melt droplets. The calculation of the melt droplet density and thermal expansion coefficient is related to its volume, while density is the dependent variable for determining the surface tension and viscosity coefficient. Evidently, the accuracy of the thermophysical properties of materials essentially depend on the precision of volume measurement. The melt droplet volume is obtained by analysing the image, thus, the precise volume of the melt droplet depends on the image quality and contour extraction algorithm. Restricted by external conditions, most of the obtained melt droplet images are of low quality and are severely polluted by noise, which complicates the determination of the thermophysical characteristics. Herein, a shape-supervised super-resolution convolutional neural network method is presented to improve image resolution and using its sub-network to extract the contour of the melt droplet directly and accurately. Compared with the existing method, this approach improves the accuracy of evaluating the thermophysical properties of the material and reduces the computational complexity by simplifying the two-step calculation process to a one-step procedure.
更多查看译文
关键词
Melt droplet thermophysical properties, Image super-resolution, Shape supervised, Contour extraction
AI 理解论文
溯源树
样例
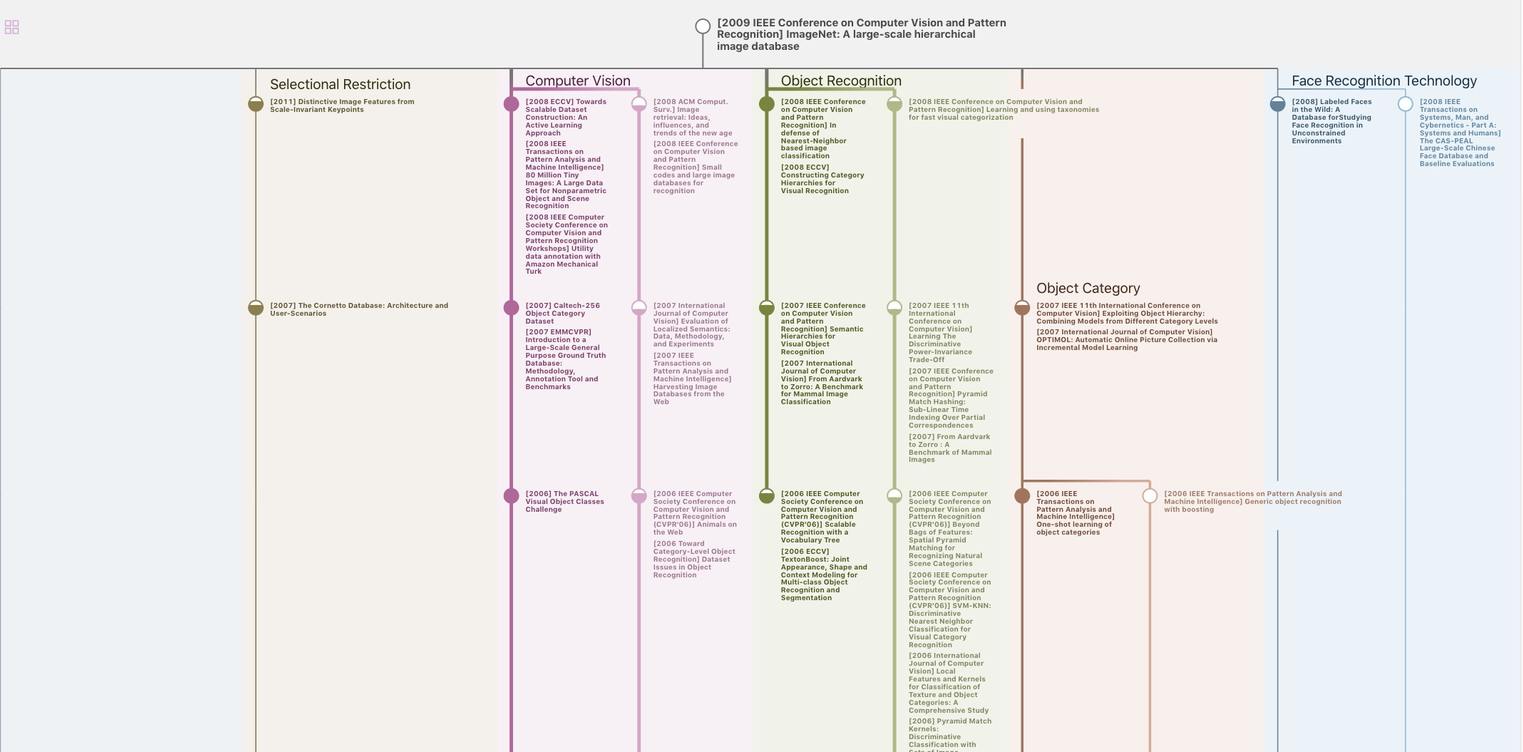
生成溯源树,研究论文发展脉络
Chat Paper
正在生成论文摘要