Multi-source data driven method for assessing the rock mass quality of a NATM tunnel face via hybrid ensemble learning models
International Journal of Rock Mechanics and Mining Sciences(2021)
摘要
Current assessments of rock mass quality of a NATM tunnel face are important in the practice of tunnel excavation. This study establishes a multi-source database and proposes a data driven method for the assessment. Thirteen multi-source variables describing the tunnel faces are considered as inputs, and the rock mass rating (RMR) values computed by the empirical formula are the target outputs. We adopted two meta machine learning models (classification and regression tree (CART) and multiple layers perceptron (MLP)) and two ensemble learning models (gradient boosting regression tree (GBRT)) and random forest (RF)) to capture the relationships between the inputs and outputs. The tree-structured Parzen estimator (TPE) algorithm is applied to automatically determine the optimized model hyper-parameters. The experimental results suggest that the proposed hybrid ensemble learning models (TPE-RF and TPE-GBRT) perform well at assessing rock mass quality. The feature importance ranks of the input variables are determined by a sensitivity analysis, which enhances the knowledge on assessing the rock mass quality of a tunnel face.
更多查看译文
关键词
Tunnel face,Rock mass quality,Data driven,Machine learning,Multi-source information
AI 理解论文
溯源树
样例
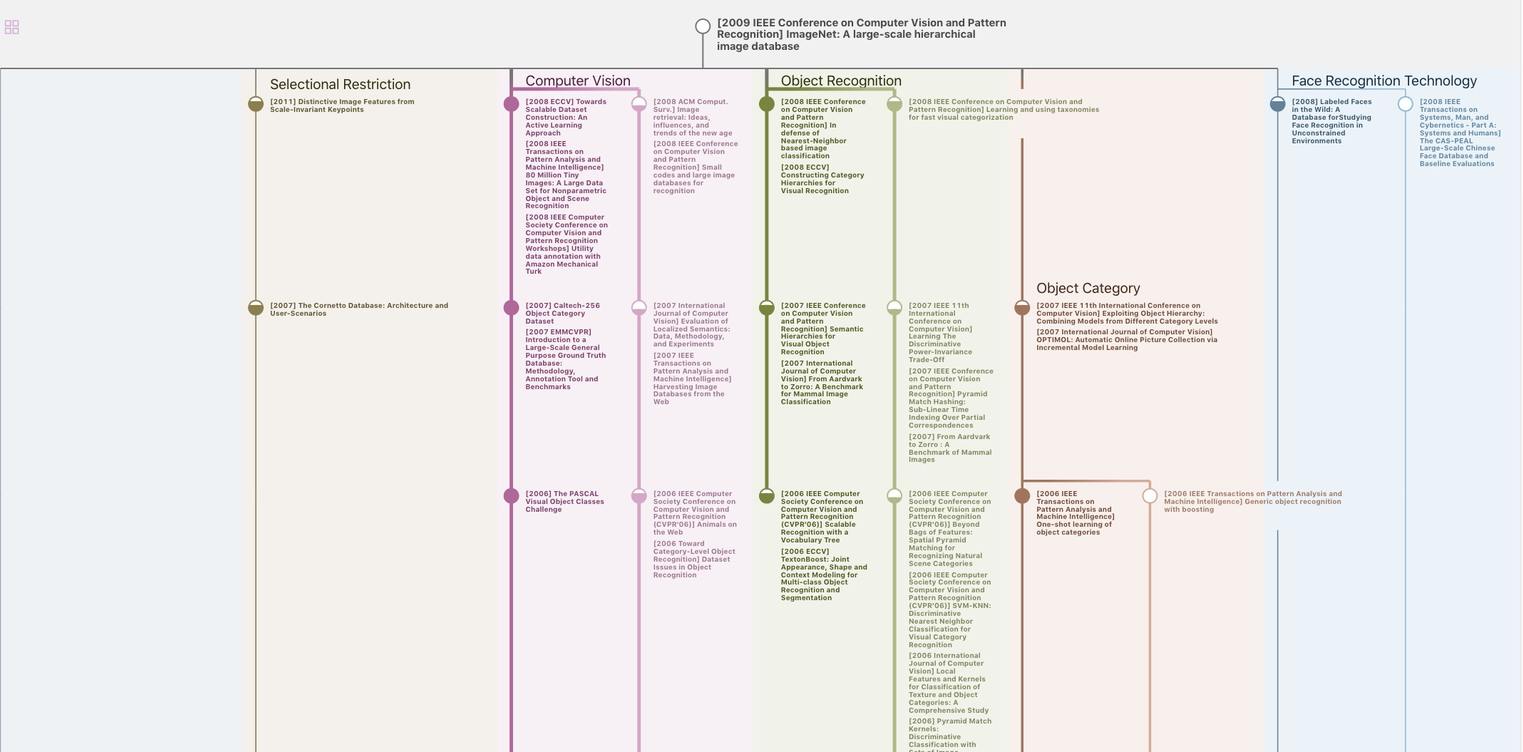
生成溯源树,研究论文发展脉络
Chat Paper
正在生成论文摘要