Bayesian identification of bots using temporal analysis of tweet storms
SOCIAL NETWORK ANALYSIS AND MINING(2021)
摘要
The key to identifying automated activity on social media is to isolate and analyze individual tweet storms that show how an account interacts with the twitterverse over time. In this work we propose the Dynamic Wavelet Fingerprint (DWFP) as a way to identify and flag this activity. Time-series representations of tweet storms are constructed using post metadata, and the DWFP converts these into binary images using a wavelet transform. To describe each tweet storm, features are extracted from the account metadata, tweet metadata, and DWFP images and then passed to a probabilistic classifier. We test three Bayesian Inference models: Multinomial Naïve Bayes, Gaussian Naïve Bayes, and Ensemble Naïve Bayes (ENB). Using Bayesian Inference structures allows us to propagate information between tweet storms by passing the posterior bot probability from one tweet storm as the prior assumption for the following tweet storm. For this proof-of-concept work we use a small, unambiguous dataset of 777 verified humans and 223 known bot accounts. We find the ENB model with four classifiers in the ensemble—decision tree, support vector machine, multi-layer perceptron, and logistic regression—provides the best results with a classification accuracy of 98.5%, and an f-score of 0.96 on the withheld validation data.
更多查看译文
关键词
Twitter, Bots, DWFP, Bayesian inference, Social media
AI 理解论文
溯源树
样例
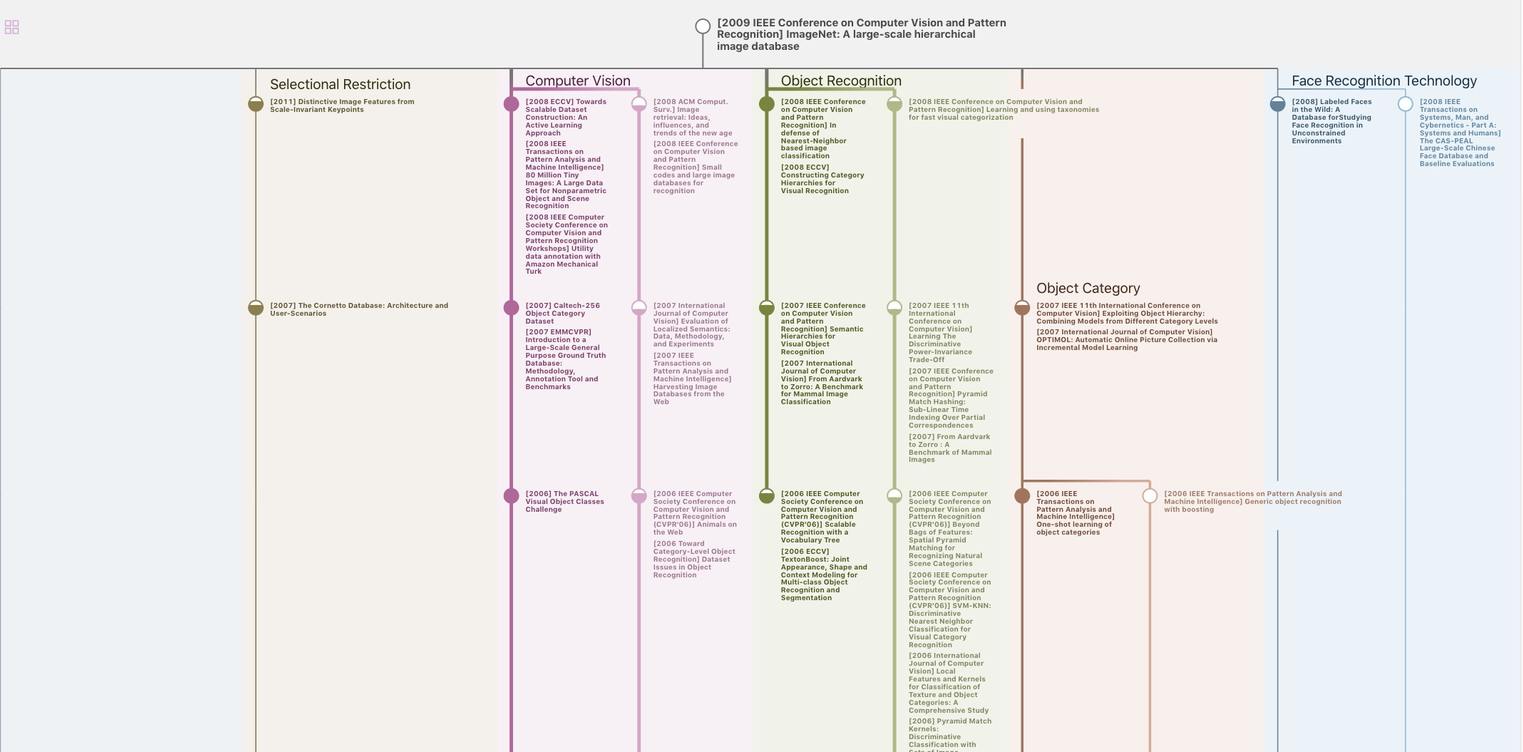
生成溯源树,研究论文发展脉络
Chat Paper
正在生成论文摘要