Distribution adaptation deep transfer learning method for cross-structure health monitoring using guided waves
STRUCTURAL HEALTH MONITORING-AN INTERNATIONAL JOURNAL(2022)
摘要
Deep learning algorithm can effectively obtain damage information using labeled samples, and has become a promising feature extraction tool for ultrasonic guided wave detection. But it is difficult to apply the monitoring expertise of structure A to structure B in most cases due to the differences in the dispersion and receiving modes of different waveguides. For multi-structure monitoring at the system level, how to transfer a trained structural health monitoring model to another different structure remains a major challenge. In this article, a cross-structure ultrasonic guided wave structural health monitoring method based on distribution adaptation deep transfer learning is proposed to solve the feature generalization problem in different monitoring structures. First, the joint distribution adaptation method is employed to adapt both the marginal distribution and conditional distribution of the guided wave signals from different structures. Second, convolutional long short-term memory network is constructed to learn the mapping relationship from adapted training samples in source domain. Batch normalization layer is implemented to balance the input tensors of each sample to the same distribution. Finally, the multi-sensor damage indexes are utilized to visually present the damage by probability imaging. The experimental results show that proposed method can utilize the single-sensor monitoring data in one structure to implement the multi-sensor damage monitoring in another structure and achieve the damage imaging visualization. The imaging performance is significantly superior to the existing principal component analysis, transfer component analysis, and other state-of-art comparison methods.
更多查看译文
关键词
Guided wave, deep learning, structural health monitoring, transfer learning, probability imaging
AI 理解论文
溯源树
样例
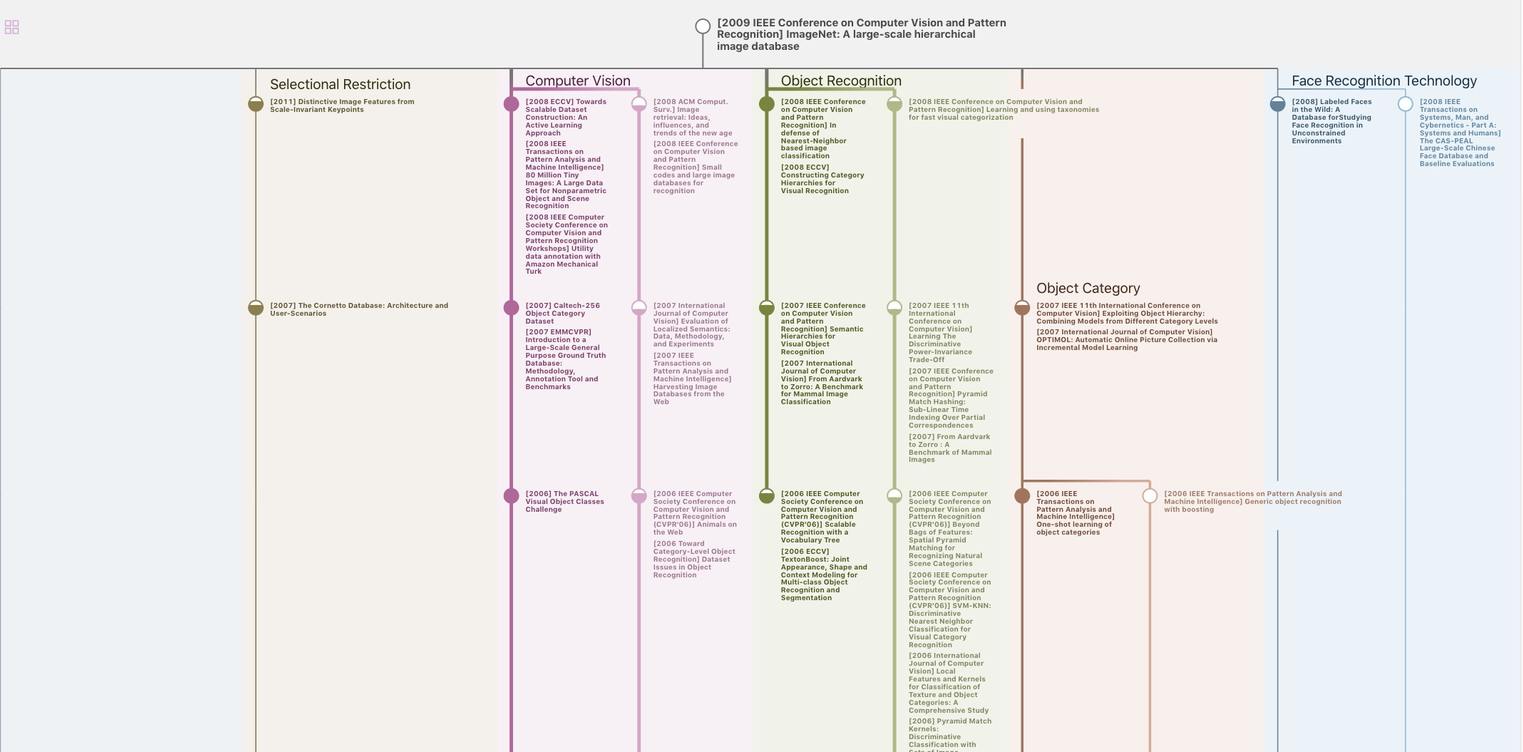
生成溯源树,研究论文发展脉络
Chat Paper
正在生成论文摘要