Linking herbivore monitoring with interpolation to map regional risk of pest species
JOURNAL OF PEST SCIENCE(2021)
摘要
Long-term monitoring networks that generate data on pest abundance are the foundation of integrated pest management. Monitoring can estimate local risk from pests and identify when pests first arrive in particular fields. However, while data on pest abundance are collected for the purpose of making management decisions in individual fields, it is often unclear how such data can be used to make landscape scale predictions of risk from pests. We addressed this by using inverse-distance weighted interpolation models to generate predictions of abundance for multiple potato crop pests from long-term monitoring data. Specifically, we collected 30,999 abundance datapoints of four pests for 4 to 11 years and used interpolation to predict abundance of each pest across a 40,250 km 2 area; these predictions were compared to observed pest data to validate our approach. We show predicted pest abundance was strongly and positively correlated with observed abundance for each of the four pests studied, with Spearman rank correlations from 0.5 to 0.8. Moreover, our interpolation approach was robust to variation in the type of monitoring data used, although interpolation parameters that produced the best fit to observed data differed slightly across species and ranged from 1 to 2. This suggests that variation in the biological traits of pests can affect interpolation models, and that models should be tailored to individual species. Overall, our study shows that interpolation is a powerful tool to integrate pest monitoring data into predictive maps that can guide management for crop pests across broad spatial scales.
更多查看译文
关键词
Geographical information systems,Integrated pest management,Landscape ecology,Potato,Predictive modeling
AI 理解论文
溯源树
样例
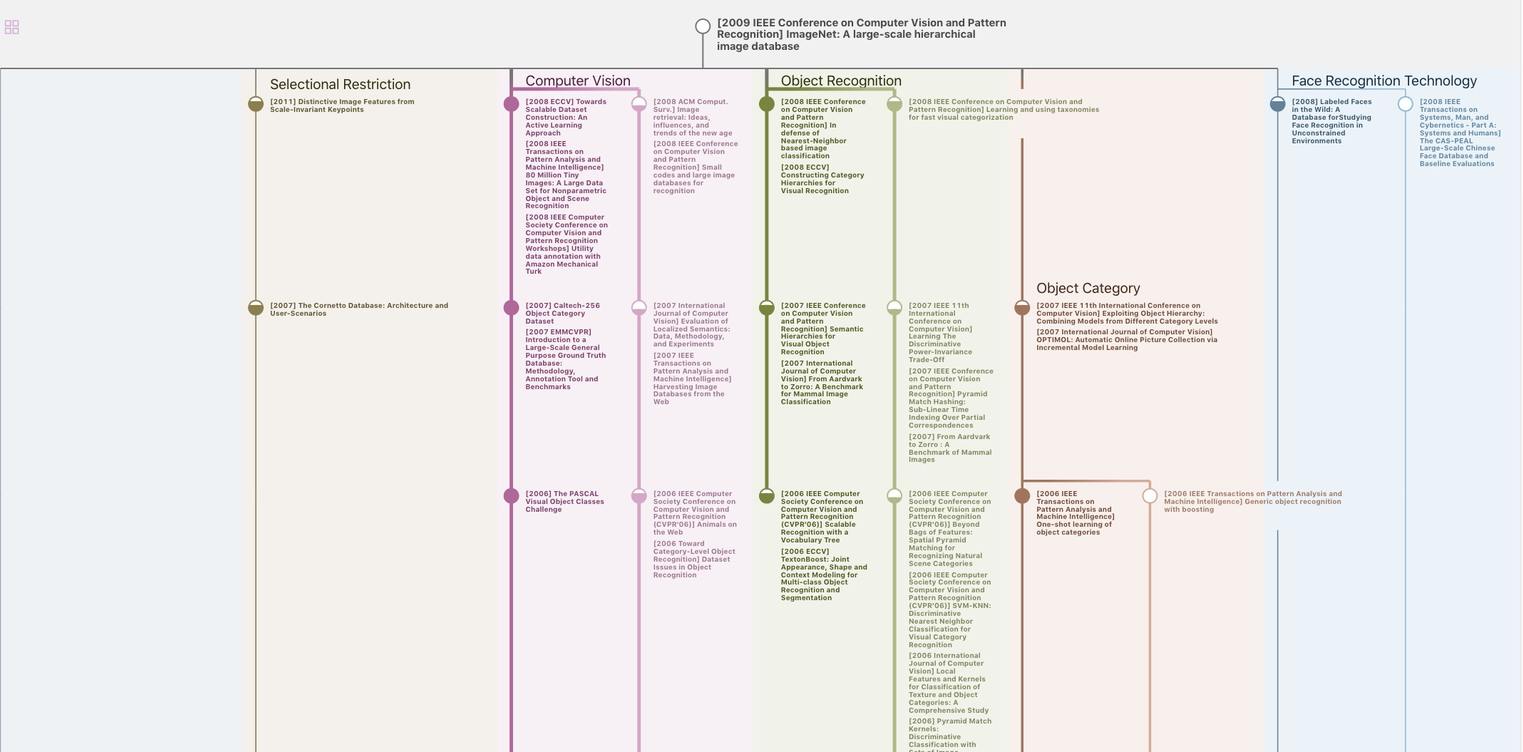
生成溯源树,研究论文发展脉络
Chat Paper
正在生成论文摘要