Task Assignment In Mobile Edge Computing Networks: A Deep Reinforcement Learning Approach
SENSORS AND SYSTEMS FOR SPACE APPLICATIONS XIV(2021)
摘要
Mobile Edge Computing (MEC) is a key technology to support the emerging low-latency Internet of Things (IoT) applications. With computing servers deployed at the network edge, the computational tasks generated by mobile users can be offloaded to these MEC servers and executed there with low latency. Meanwhile, with the ever-increasing number of mobile users, the communication resource for offloading and the computational resource allocated to each user would become quite limited. As a result, it would be difficult for the MEC servers alone to process all the tasks in a timely manner. An effective approach to deal with this challenge is offloading a proportion of the tasks at MEC servers to the cloud servers, such that both types of servers are efficiently utilized to reduce latency. Given multiple MEC and cloud servers and the dynamics of communication latency, intelligent task assignment between different servers is required. In this paper, we propose a deep reinforcement learning (DRL) based task assignment scheme for MEC networks, aiming to minimize the average task processing latency. Two design parameters of task assignment are optimized, including cloud server selection and task partitioning. Such a problem is formulated as a Markov Decision Process (MDP) and solved with a DRL-based approach, which enables the edge servers to capture the system dynamics and make optimized task assignment strategies accordingly. Simulation results show that the proposed scheme can significantly lower the average task completion latency.
更多查看译文
关键词
Mobile edge computing, task assignment, deep reinforcement learning
AI 理解论文
溯源树
样例
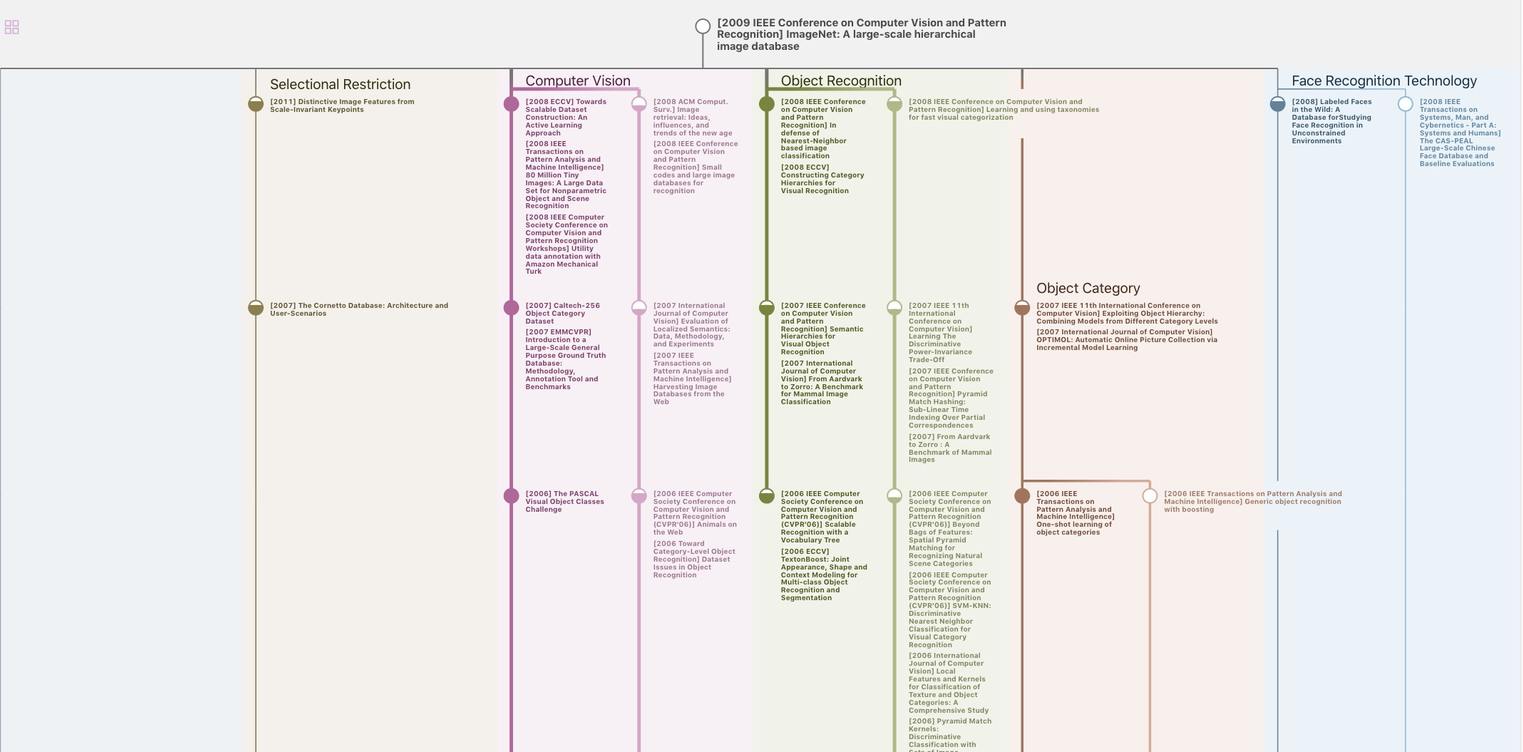
生成溯源树,研究论文发展脉络
Chat Paper
正在生成论文摘要