On Image Classification In Video Analysis Of Omnidirectional Apis Mellifera Traffic: Random Reinforced Forests Vs. Shallow Convolutional Networks
APPLIED SCIENCES-BASEL(2021)
摘要
Omnidirectional honeybee traffic is the number of bees moving in arbitrary directions in close proximity to the landing pad of a beehive over a period of time. Automated video analysis of such traffic is critical for continuous colony health assessment. In our previous research, we proposed a two-tier algorithm to measure omnidirectional bee traffic in videos. Our algorithm combines motion detection with image classification: in tier 1, motion detection functions as class-agnostic object location to generate regions with possible objects; in tier 2, each region from tier 1 is classified by a class-specific classifier. In this article, we present an empirical and theoretical comparison of random reinforced forests and shallow convolutional networks as tier 2 classifiers. A random reinforced forest is a random forest trained on a dataset with reinforcement learning. We present several methods of training random reinforced forests and compare their performance with shallow convolutional networks on seven image datasets. We develop a theoretical framework to assess the complexity of image classification by a image classifier. We formulate and prove three theorems on finding optimal random reinforced forests. Our conclusion is that, despite their limitations, random reinforced forests are a reasonable alternative to convolutional networks when memory footprints and classification and energy efficiencies are important factors. We outline several ways in which the performance of random reinforced forests may be improved.
更多查看译文
关键词
image classification, random forests, convolutional networks, reinforcement learning, random reinforced forests, electronic beehive monitoring, machine learning, video processing, image datasets, apis mellifera
AI 理解论文
溯源树
样例
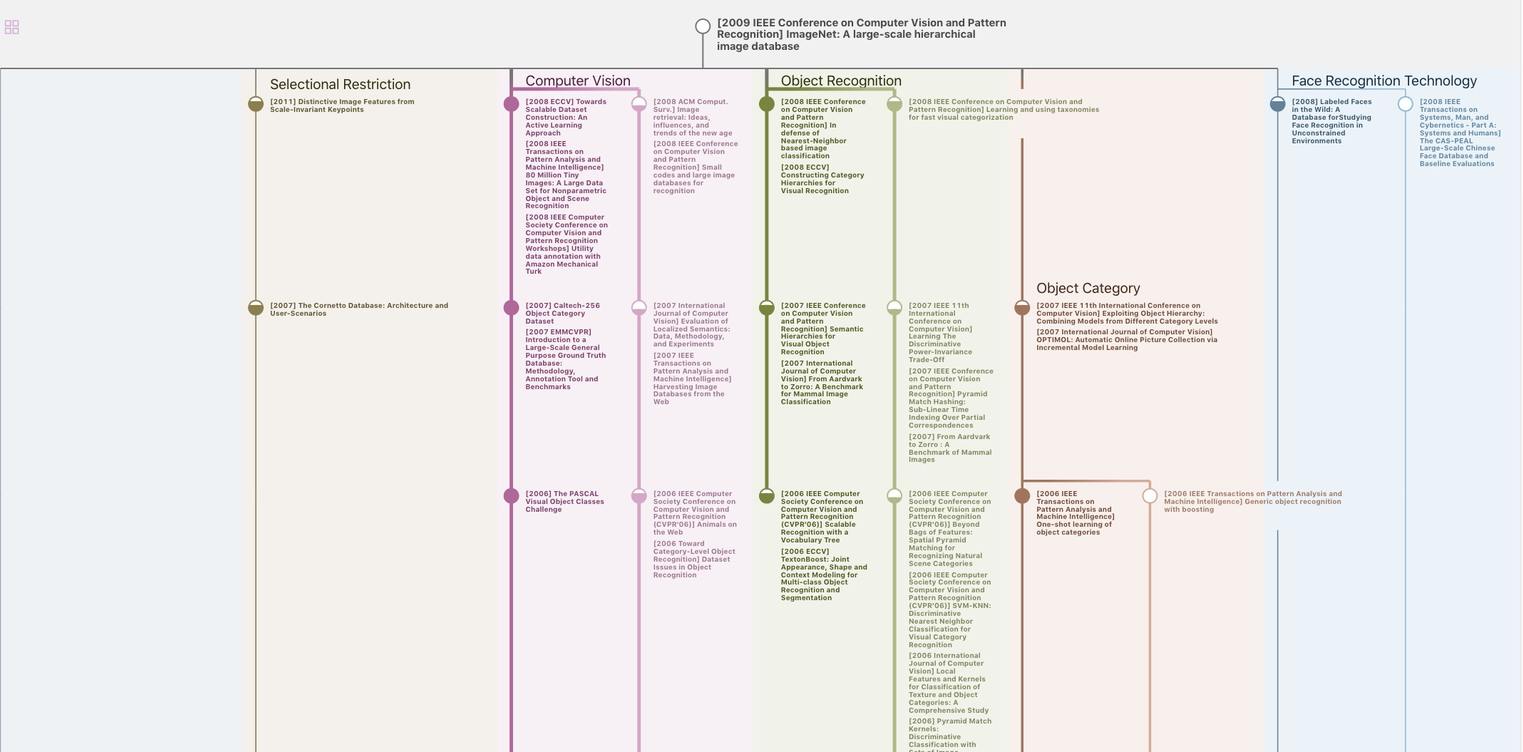
生成溯源树,研究论文发展脉络
Chat Paper
正在生成论文摘要