Medical image segmentation based on dual-channel integrated cross-layer residual algorithm
MULTIMEDIA TOOLS AND APPLICATIONS(2021)
摘要
Segmentation tasks in medical images have always been a hot topic in the medical imaging field. Compared with traditional images, medical images have richer semantics, which increases the difficulty of feature learning. This paper proposes a new end-to-end dual-channel integrated cross-layer residual algorithm (TIC-Net) based on deep learning to fully mine the semantic information between features for medical image segmentation. First, in the encoder, we built a dual-channel network of traditional convolution and dilated convolution using multiple structures to learn different semantic information from the image and from feature fusion and residual calculation to achieve feature joint mining. Second, we added two sets of new integrated modules between the decoder and encoder to fully fuse the global and local features of each layer of the image in the encoder. Finally, in the decoder, we use a cross-layer feature residual fusion strategy to obtain more semantic information. Compared with the existing partial segmentation model, the proposed deep learning algorithm model achieves the best results with the Kaggle and MICCAI datasets.
更多查看译文
关键词
Two-channel network, Deep learning, Integrated module, Medical image segmentation
AI 理解论文
溯源树
样例
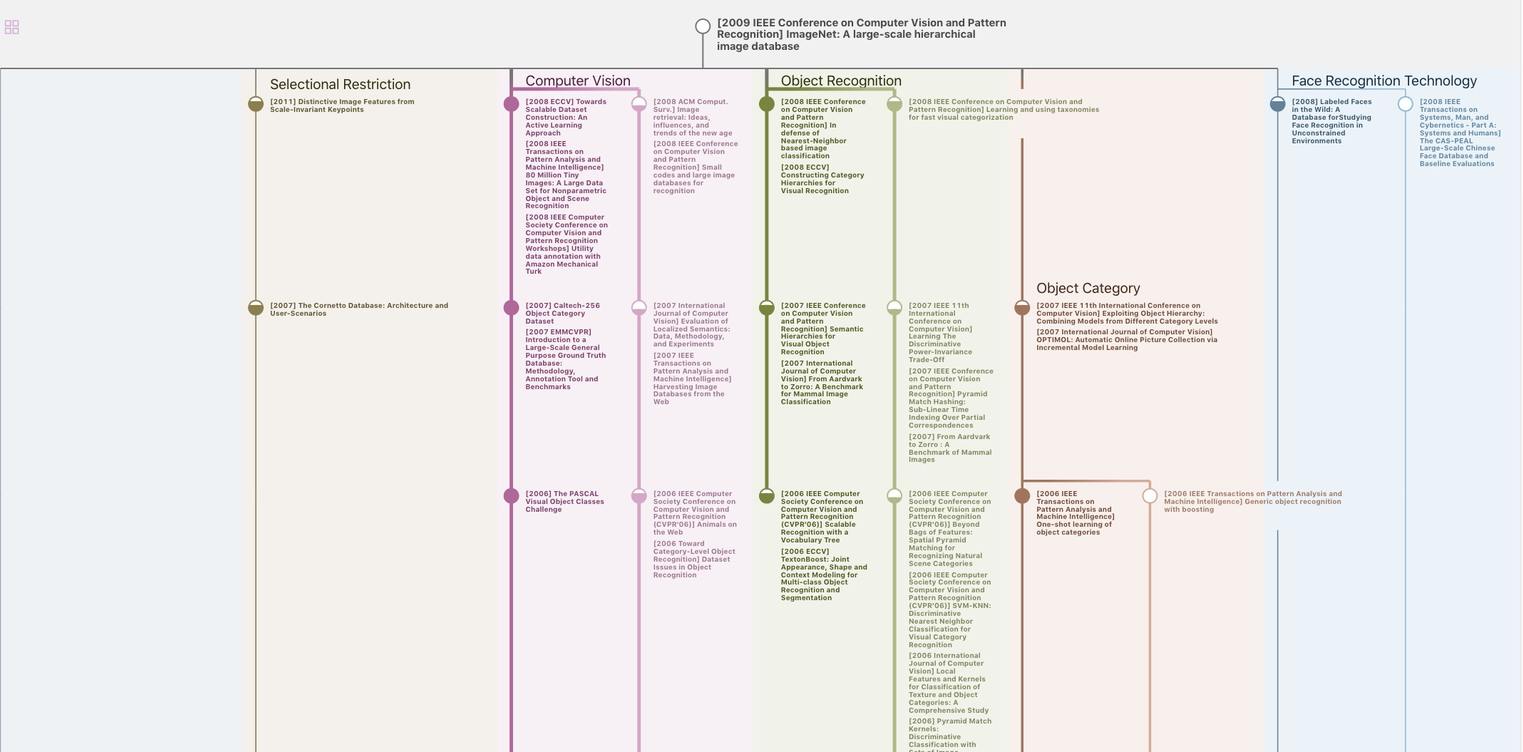
生成溯源树,研究论文发展脉络
Chat Paper
正在生成论文摘要