Applying Reinforcement Learning And Tree Search To The Unit Commitment Problem
APPLIED ENERGY(2021)
摘要
Recent advances in artificial intelligence have demonstrated the capability of reinforcement learning (RL) methods to outperform the state of the art in decision-making problems under uncertainty. Day-ahead unit commitment (UC), scheduling power generation based on forecasts, is a complex power systems task that is becoming more challenging in light of increasing uncertainty. While RL is a promising framework for solving the UC problem, the space of possible actions from a given state is exponential in the number of generators and it is infeasible to apply existing RL methods in power systems larger than a few generators. Here we present a novel RL algorithm, guided tree search, which does not suffer from an exponential explosion in the action space with increasing number of generators. The method augments a tree search algorithm with a policy that intelligently reduces the branching factor. Using data from the GB power system, we demonstrate that guided tree search outperforms an unguided method in terms of computational complexity, while producing solutions that show no performance loss in terms of operating costs. We compare solutions against mixed-integer linear programming (MILP) and find that guided tree search outperforms a solution using reserve constraints, the current industry approach. The RL solutions exhibit complex behaviours that differ qualitatively from MILP, demonstrating its potential as a decision support tool for human operators.
更多查看译文
关键词
Unit commitment, Reinforcement learning, Tree search, Deep learning, Power systems
AI 理解论文
溯源树
样例
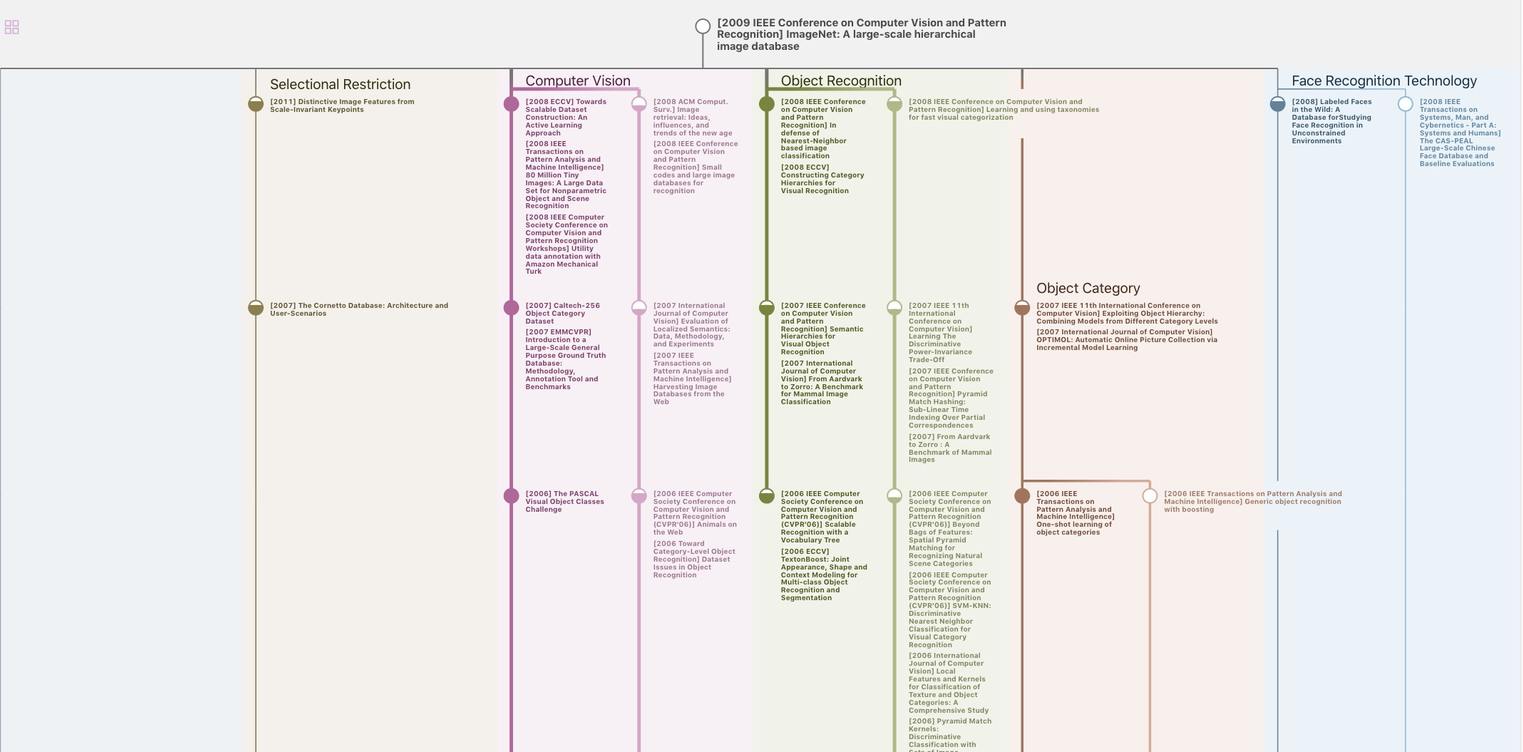
生成溯源树,研究论文发展脉络
Chat Paper
正在生成论文摘要