Different-factor compact-form model-free adaptive control with neural networks for MIMO nonlinear systems
ASIAN JOURNAL OF CONTROL(2022)
摘要
In this paper, a different-factor structure-based compact-form model-free adaptive control method with neural networks (DF-CFMFAC-NN) is proposed for a class of general multiple-input and multiple-output (MIMO) nonlinear systems. Its novelty lies in that it is a pure data-driven control method using merely input/output data without any model information involved. Moreover, by virtue of the different-factor structure, it addresses the problem in the prototype CFMFAC that mainly deals with a class of MIMO systems with similar channel characteristics under a fixed topology. Aiming at different characteristics between control channels, widely existing in MIMO systems, DF-CFMFAC-NN with learning parameters shows powerful tracking ability and flexible design for each control channel, and learning parameters are auto-tuned by back propagation neural networks (BPNNs) online based on their self-learning and self-adapting properties. In the tuning process, a one-step-ahead partial derivative is directly derived by the dynamic linearization technique utilized in DF-CFMFAC, which greatly improves the prediction accuracy of parameters. The convergence of tracking error and the stability of the tuning process are guaranteed by rigorous theoretical analysis. A coal mill system is provided to demonstrate its effectiveness and practicability, demonstrating it to be a promising control method for MIMO nonlinear systems.
更多查看译文
关键词
back propagation neural network,coal mill system,compact-form model-free adaptive control,different-factor structure,parameter self-tuning
AI 理解论文
溯源树
样例
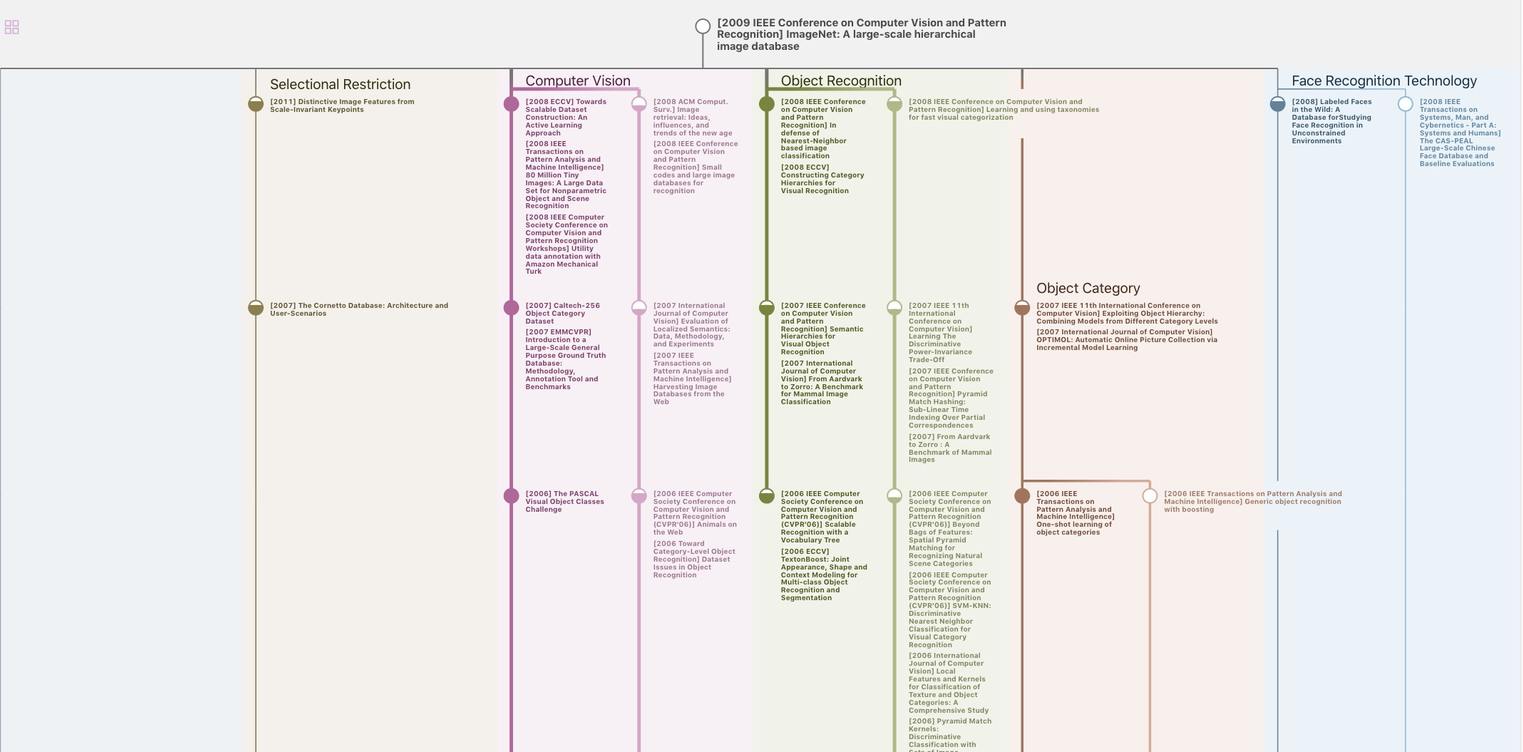
生成溯源树,研究论文发展脉络
Chat Paper
正在生成论文摘要