A deep hypersphere approach to high-dimensional anomaly detection
Applied Soft Computing(2022)
摘要
The term of Curse of Dimensionality implicitly expresses the challenge for anomaly detection in a high-dimensional space. Because the distribution of anomalies in the high-dimensional spatial data is usually too sparse to provide sufficient information for detecting anomalies. In addition, irrelevant attributes may be seen as noise in the input data, which masks the true anomalies, so that it is difficult to choose a subspace of the input data that highlights the relevant attributes. In this case, the task becomes even harder if one aims at learning a compact boundary to distinguish anomalies from normal data. To address this issue, we proposed a detection method using the combination of an autoencoder and a hypersphere. In addition, an angle kernel and a radius kernel are also derived in order to learn a compact boundary of distinguishing anomalous and normal instances. Results show that our method outperforms the state-of-the-art detection methods in anomalous detection accuracy and the ability of learning a compact boundary. Moreover, our method also addresses the issue of blurred boundary in searching normal data in high dimensional dataset and when the information is insufficient due to a limited number of potential anomalies. We find that the measurement of angle similarity between data points during searching gains more advantages for learning a compact boundary than using the measurement of distance similarity. Since angle similarity is not only helpful for flexibly controlling search in normal data region, but also tightens the searched region of anomalies nearby the boundary. We also find that noise in data as a negative factor can deteriorate detection accuracy much more quickly than dimensionality does. Our findings indicate that the determination of hypersphere radius relies more on data dimensionality in a high-dimensional space than that in a low-dimensional space. However, in a low-dimensional space the radius is more likely correlated with data volume.
更多查看译文
关键词
Anomaly detection,Boundaries,Deep networks,High-dimensional data,Hypersphere
AI 理解论文
溯源树
样例
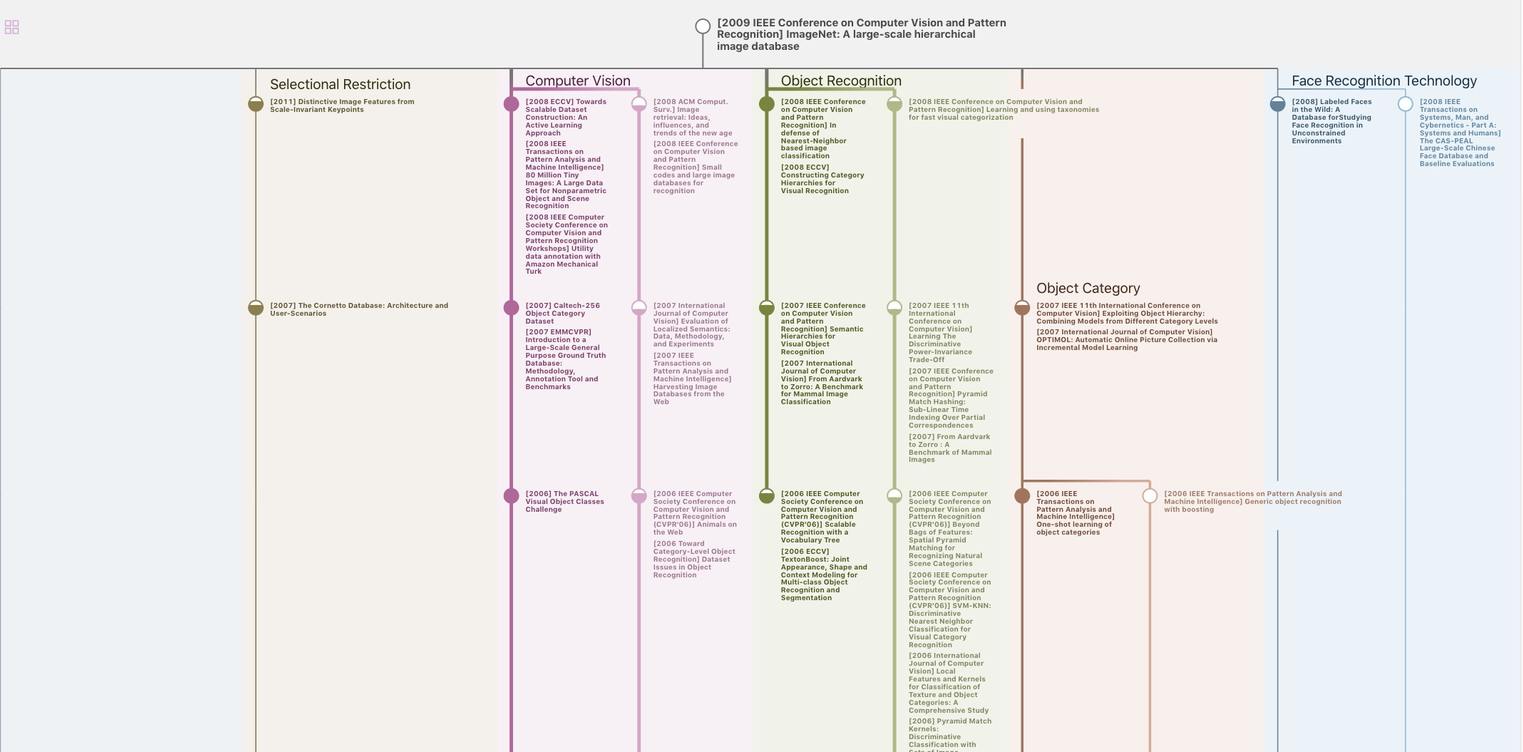
生成溯源树,研究论文发展脉络
Chat Paper
正在生成论文摘要