New and updated global empirical seawater property estimation routines
LIMNOLOGY AND OCEANOGRAPHY-METHODS(2021)
摘要
We introduce three new Empirical Seawater Property Estimation Routines (ESPERs) capable of predicting seawater phosphate, nitrate, silicate, oxygen, total titration seawater alkalinity, total hydrogen scale pH (pH(T)), and total dissolved inorganic carbon (DIC) from up to 16 combinations of seawater property measurements. The routines generate estimates from neural networks (ESPER_NN), locally interpolated regressions (ESPER_LIR), or both (ESPER_Mixed). They require a salinity value and coordinate information, and benefit from additional seawater measurements if available. These routines are intended for seawater property measurement quality control and quality assessment, generating estimates for calculations that require approximate values, original science, and producing biogeochemical property context from a data set. Relative to earlier LIR routines, the updates expand their functionality, including new estimated properties and combinations of predictors, a larger training data product including new cruises from the 2020 Global Data Analysis Project data product release, and the implementation of a first-principles approach for quantifying the impacts of anthropogenic carbon on DIC and pH(T). We show that the new routines perform at least as well as existing routines, and, in some cases, outperform existing approaches, even when limited to the same training data. Given that additional training data has been incorporated into these updated routines, these updates should be considered an improvement over earlier versions. The routines are intended for all ocean depths for the interval from 1980 to similar to 2030 c.e., and we caution against using the routines to directly quantify surface ocean seasonality or make more distant predictions of DIC or pH(T).
更多查看译文
关键词
estimation,property
AI 理解论文
溯源树
样例
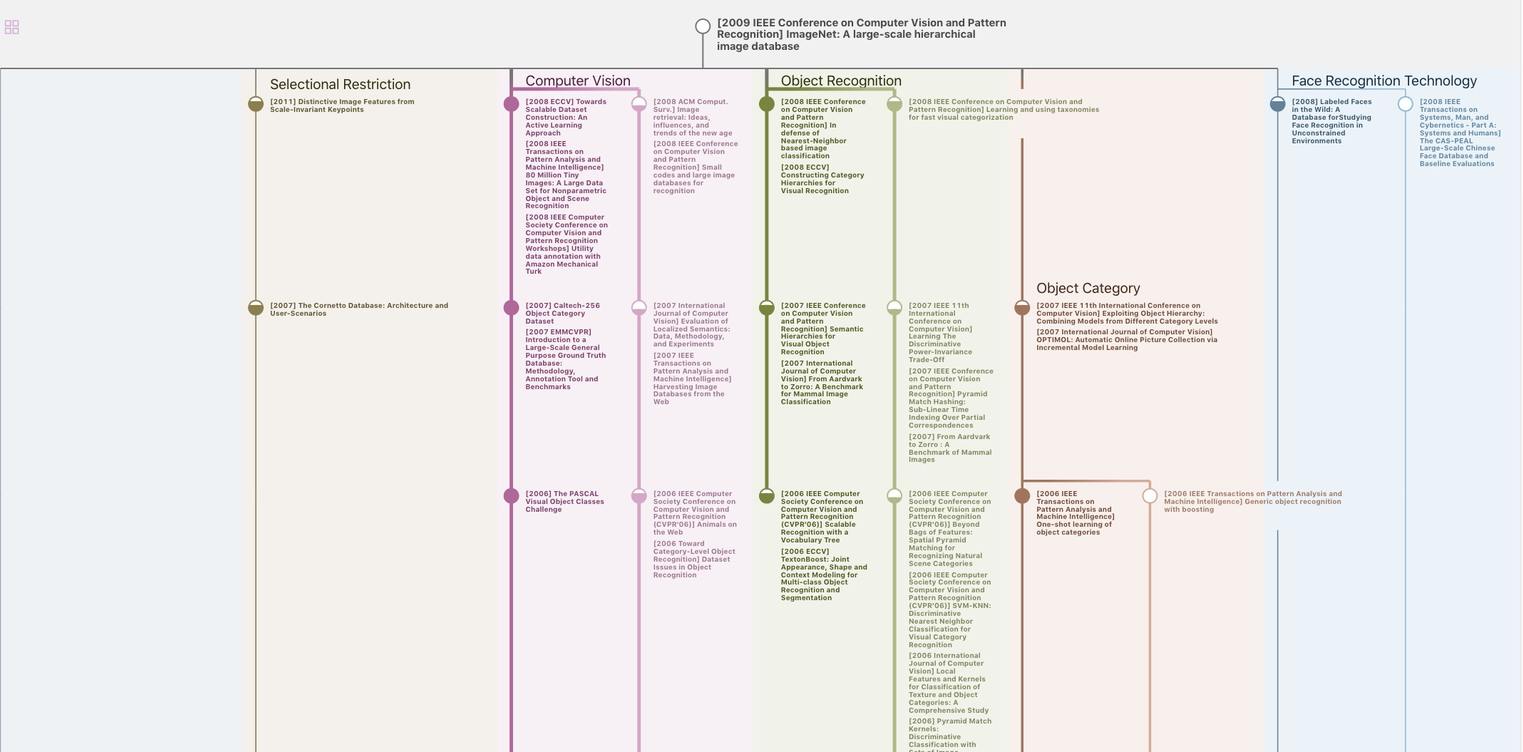
生成溯源树,研究论文发展脉络
Chat Paper
正在生成论文摘要