A Bayesian approach to calibrate system dynamics models using Hamiltonian Monte Carlo
SYSTEM DYNAMICS REVIEW(2021)
摘要
Model calibration is an essential test that dynamic hypotheses must pass in order to serve as tools for decision-making. In short, it is the search for a match between actual and simulated behaviours using parameter inference. Here, we approach such an inference process from a Bayesian perspective. Under this paradigm, we provide statements about the parameters (viewed as random variables) and data in probabilistic terms. These statements stem from a posterior distribution whose solution is often found via statistical simulation. However, the uptake of these methods within the system dynamics field has been somewhat limited, and state-of-the-art algorithms have not been explored. Therefore, we introduce Hamiltonian Monte Carlo (HMC), an efficient algorithm that outperforms random-walk methods in exploring complex parameter spaces. We apply HMC to calibrate an SEIR model and frame the process within a practical workflow. In doing so, we also recommend visualisation tools that facilitate the communication of results. (c) 2021 The Authors. System Dynamics Review published by John Wiley & Sons Ltd on behalf of System Dynamics Society.
更多查看译文
关键词
system dynamics models,<scp>bayesian</scp>
AI 理解论文
溯源树
样例
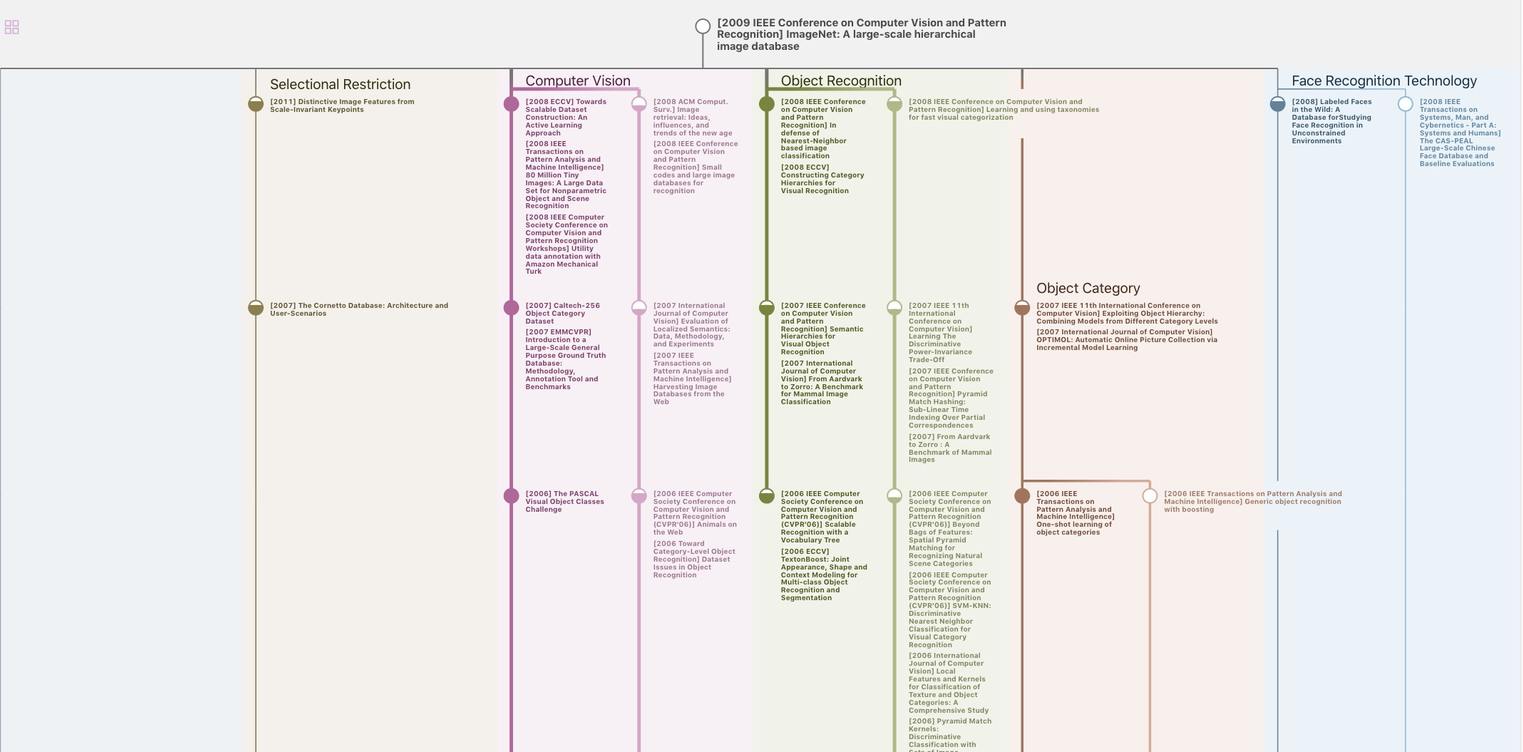
生成溯源树,研究论文发展脉络
Chat Paper
正在生成论文摘要