Organelle Topology Is A New Breast Cancer Cell Classifier
FASEB JOURNAL(2021)
摘要
Breast cancer is a highly heterogenous disease, both phenotypically and genetically. Importantly a correlation between intra-tumoral heterogeneity, drug resistance and negative clinical outcome has been established. Previously, quantity or subcellular location of protein biomarkers have been compared to identify tumor tissue types. To-date, mass cytometry and single-cell sequencing studies have been used to assess spatial intra-tumor heterogeneity by identifying a range of phenotypic and genetic alterations across different regions of a single tumor. Recently, cell morphology has been indicated as a direct readout of the functional phenotypic state of an individual cancer cell. Here, we hypothesize that the spatial context of organelles within cancer cells, specifically their subcellular location and inter-organelle relationships (topology), can be used to inform breast cancer cell classification. Since numerous correlations between biologic behaviors and pathologic findings have been well-established, we propose that organelle topological heterogeneity reveals the long-term adaptation of organelles and cytoskeletal networks to match breast cancer cell type status. Thus, this study aims to investigate the heterogeneity of organelle topology and morphology in breast cancer cells to increase our basic understanding of breast cancer biology on a subcellular level. We have introduced a novel approach that quantifies, for the first time, the feature of organelles in breast cancer cells, removing the bias of visual interpretation to classify different cell lines based on organelle morphology and topology. This method was tested on three different organelle datasets: mitochondria, early endosomes and recycling endosomes in a panel of human breast cancer cells, including T47D (estrogen receptor-positive), MDAMB231, MDAMB436 and MDAMB468 (triple negative) and AU565 (HER2 positive), as well as non-cancerous mammary epithelial MCF10A cells. High resolution Airyscan microscopy was used to collect z-stacks across cells labeled with fluorescently labeled-transferrin and immunostained with anti-Tom20 and anti-EEA1 to label the recycling, early endosomal and mitochondria networks, respectively. Subsequently, 3D surface rendering of organelle objects was performed using IMARIS image analysis software. A morphometric evaluation of mitochondrial and endosomal compartments resulted in 34 topology and morphology parameters. Application of Random Forest machine learning based classification to 18 of these 34 parameters generated the highest accuracy in breast cancer cell classification. We systematically evaluated how different parameter combinations affected the machine learning-based cancer cell classification and discovered that topology parameters were crucial to achieve the highest accuracy. Based on organelle spatial distribution and their interaction with neighbor organelles (topology) a classification accuracy over 95% was achieved to distinguish between a variety of human breast cancer cell lines of differing subtype and aggressiveness. These findings lay the groundwork for using quantitative topological organelle features as an effective method to analyze and classify breast cancer cell phenotypes.
更多查看译文
关键词
Phenotypic Profiling
AI 理解论文
溯源树
样例
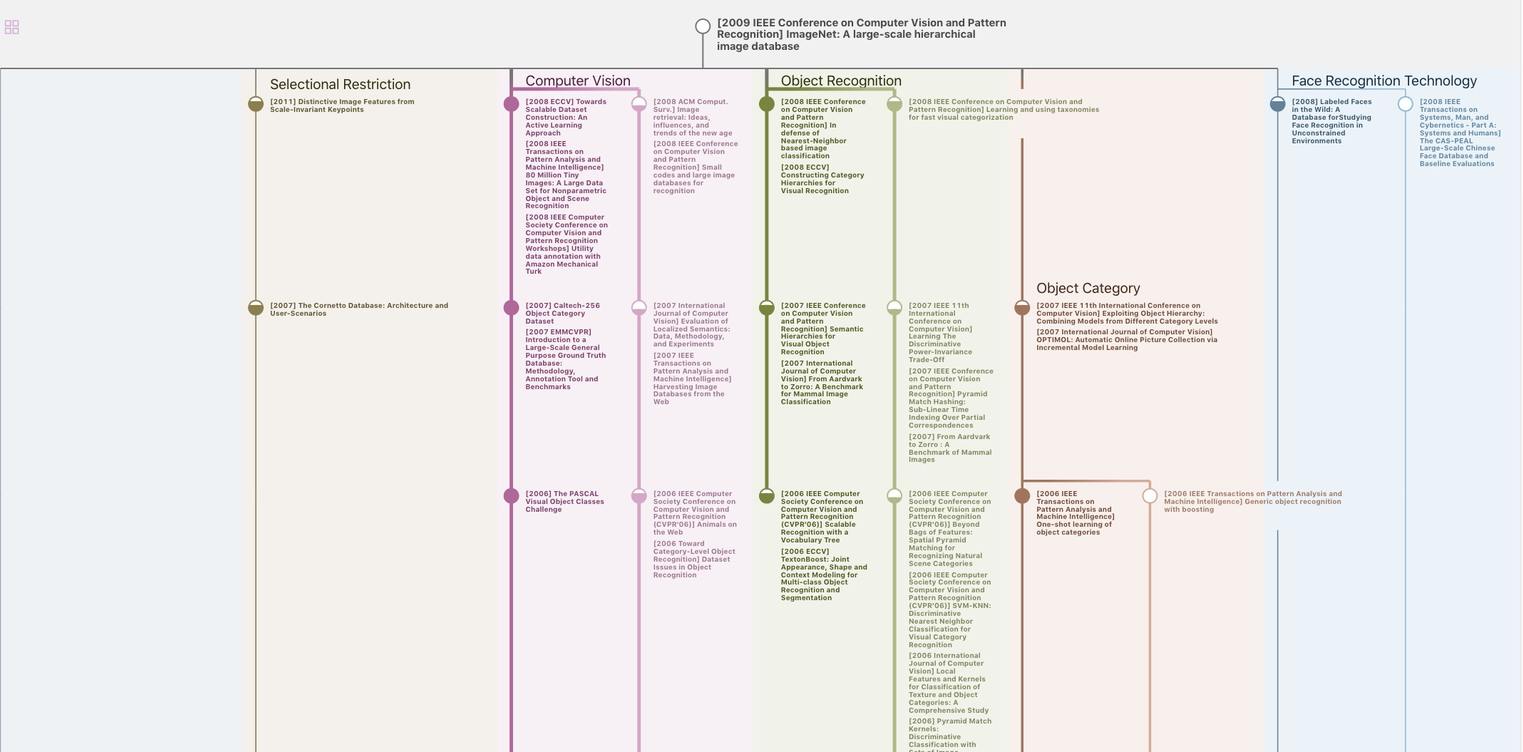
生成溯源树,研究论文发展脉络
Chat Paper
正在生成论文摘要