Spiking Neuron Model for Dopamine-Like Learning of Neuromorphic Systems with Memristive Synaptic Weights
NANOBIOTECHNOLOGY REPORTS(2021)
摘要
— The development of neuromorphic systems based on spiking neural networks with memristive synaptic weights (nanostructured elements of electrically rewritable nonvolatile memory) is a promising direction in hardware design for solving artificial intelligence problems from the standpoint of significantly reducing energy consumption while increasing the performance of neuromorphic computations. Currently, there is active search for optimal algorithms for such computations and original approaches for their machine learning. One promising option is reinforcement learning using an analog of dopamine modulation observed in the central nervous system of humans and animals. Here we propose an electrophysical model and circuit design of an artificial neuron for dopamine-like learning of spiking networks at the hardware level, as well as an analysis of how the parameters of memristive structures influence the features of learning synaptic connections between pairs of such neurons based on them.
更多查看译文
AI 理解论文
溯源树
样例
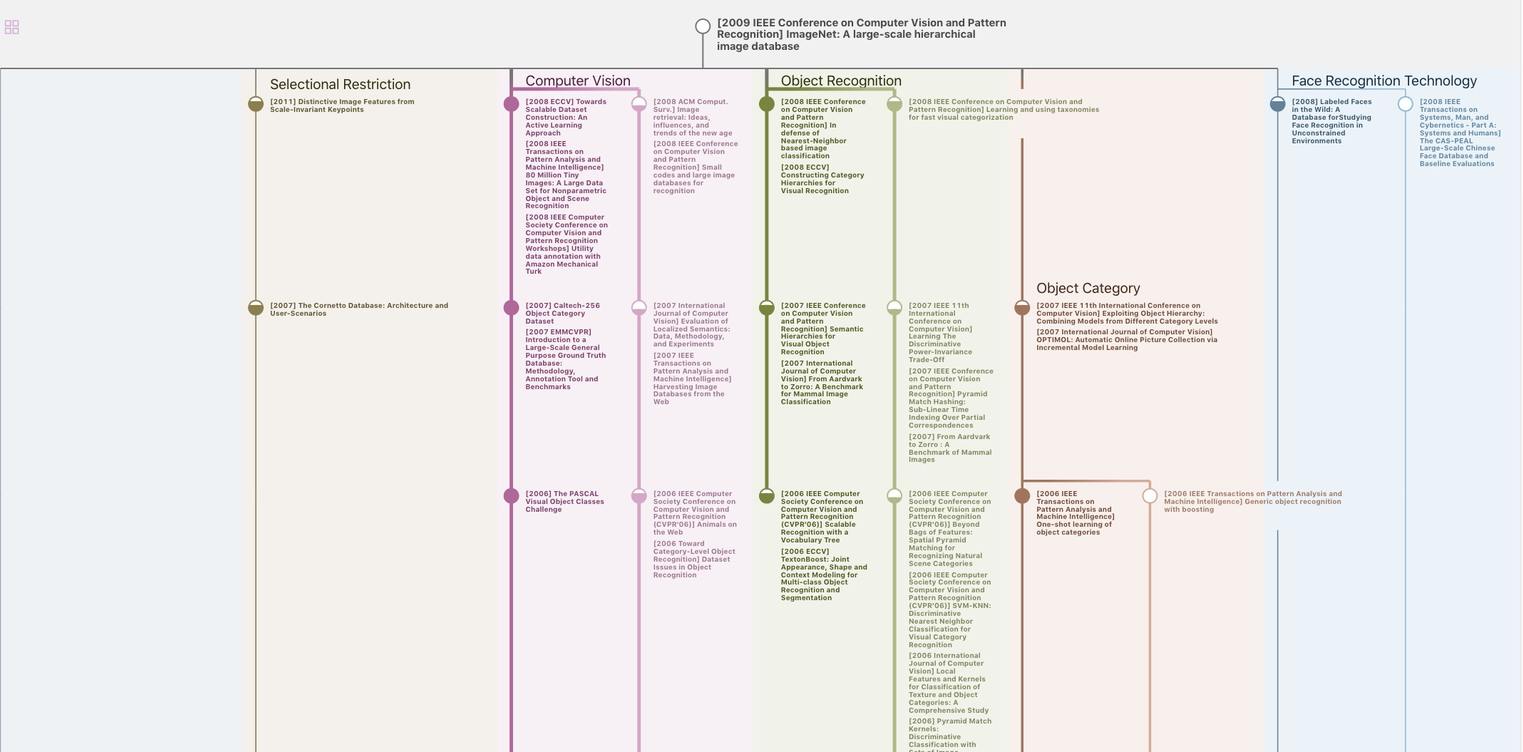
生成溯源树,研究论文发展脉络
Chat Paper
正在生成论文摘要