Online Bus Speed Prediction With Spatiotemporal Interaction: A Laplace Approximation-Based Bayesian Approach
IEEE ACCESS(2021)
摘要
This study proposes a novel Bayesian hierarchical approach for online bus speed prediction by explicitly accounting for the spatiotemporal interaction (STI) of speed observations. The use of Laplace approximation can expedite the estimation of Bayesian models and enable the implementation of online prediction. Large numbers of trials are carried out to identify significant predictors and the optimal length of the look-back time window to achieve the highest prediction accuracy. The spatiotemporal interacting patterns are also explored, and results show that the Type IV model assuming the structured spatial effect interacts with the structured temporal effect can best accommodate the bus speed data. Besides, prediction errors of the Type IV model randomly distribute over time and space. The proposed model can achieve high prediction accuracy and computational efficiency without compromising the interpretability of the contributing factors and the unobserved spatiotemporal heterogeneity. The proposed model can be used to assist public transit operation and management, such as bus scheduling, congestion warning, and the development of proactive measures to mitigate bus delays.
更多查看译文
关键词
Predictive models, Computational modeling, Bayes methods, Spatiotemporal phenomena, Data models, Roads, Correlation, Bus speed prediction, Bayesian hierarchical approach, spatiotemporal interaction, Laplace approximation, public transit operation
AI 理解论文
溯源树
样例
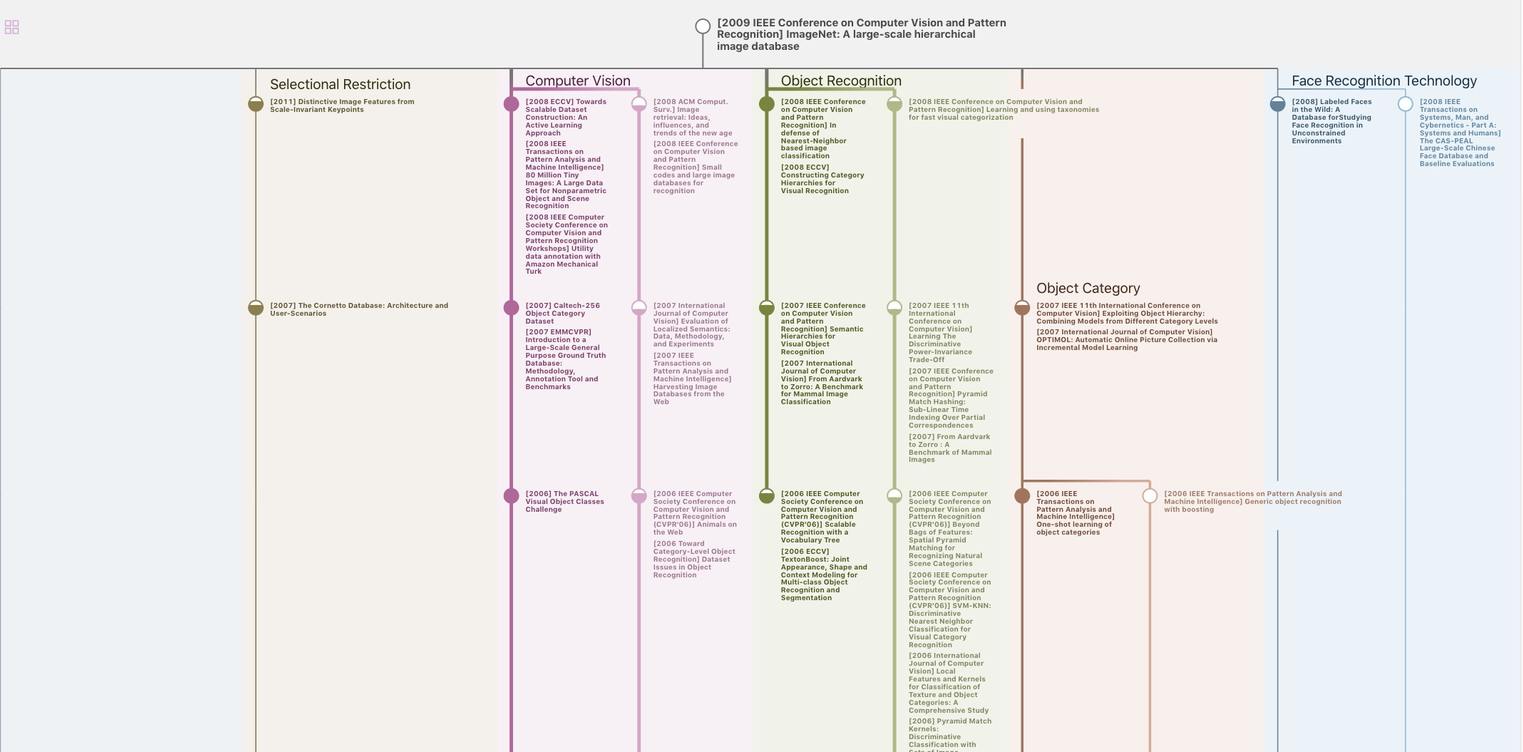
生成溯源树,研究论文发展脉络
Chat Paper
正在生成论文摘要