Evaluating Fairness of Algorithmic Risk Assessment Instruments: the Problem with Forcing Dichotomies
Criminal justice and behavior(2021)
摘要
Researchers and stakeholders have developed many definitions to evaluate whether algorithmic pretrial risk assessment instruments are fair in terms of their error and accuracy. Error and accuracy are often operationalized using three sets of indicators: false-positive and false-negative percentages, false-positive and false-negative rates, and positive and negative predictive value. To calculate these indicators, a threshold must be set, and continuous risk scores must be dichotomized. We provide a data-driven examination of these three sets of indicators using data from three studies on the most widely used algorithmic pretrial risk assessment instruments: the Public Safety Assessment, the Virginia Pretrial Risk Assessment Instrument, and the Federal Pretrial Risk Assessment. Overall, our findings highlight how conclusions regarding fairness are affected by the limitations of these indicators. Future work should move toward examining whether there are biases in how the risk assessment scores are used to inform decision-making.
更多查看译文
关键词
Risk assessment,pretrial,bias,algorithmic fairness,Public Safety Assessment,Virginia Pretrial Risk Assessment Instrument,Federal Pretrial Risk Assessment
AI 理解论文
溯源树
样例
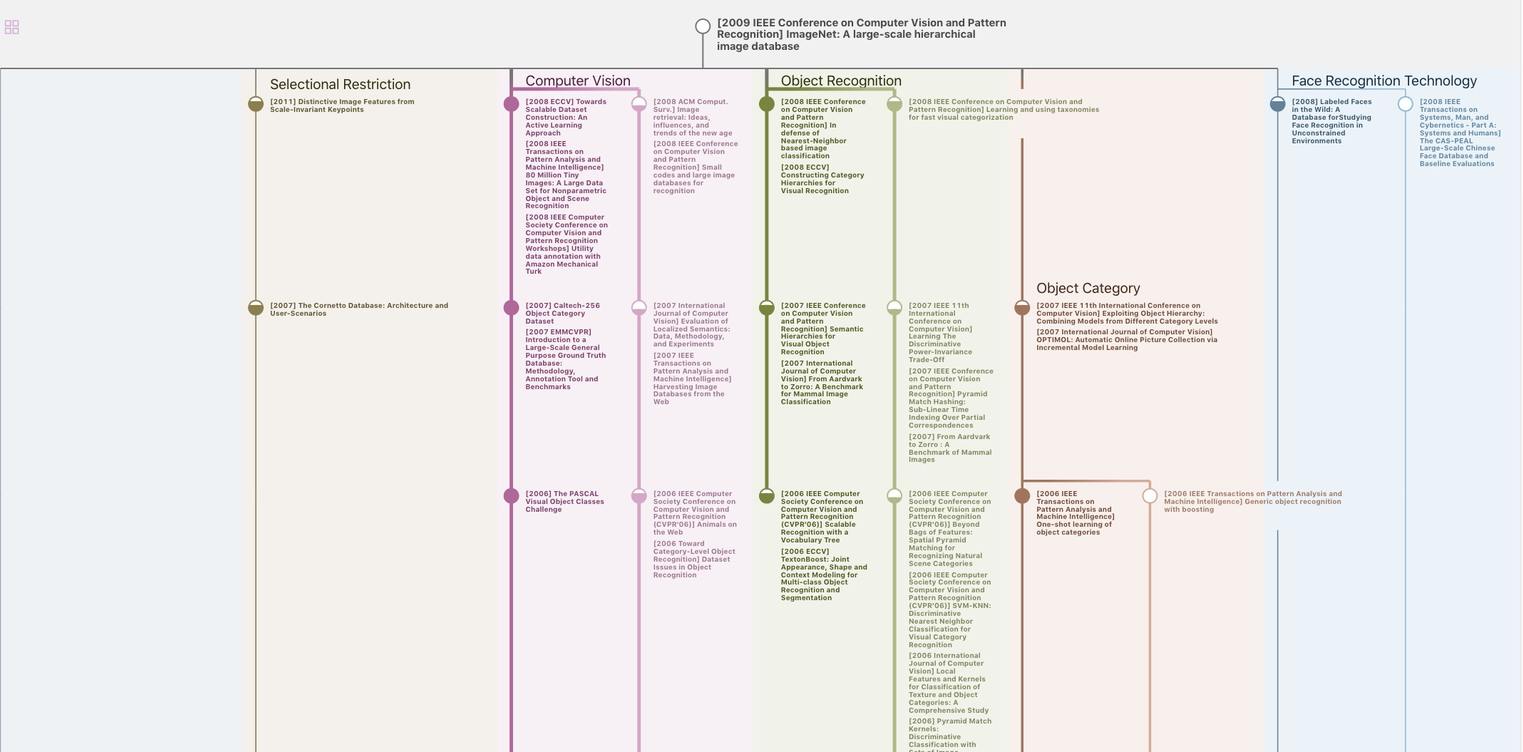
生成溯源树,研究论文发展脉络
Chat Paper
正在生成论文摘要