Deep Learning Enabled Beam Tracking For Non-Line Of Sight Millimeter Wave Communications
IEEE OPEN JOURNAL OF THE COMMUNICATIONS SOCIETY(2021)
摘要
To solve the complex beam alignment issue in non-line-of-sight (NLOS) millimeter wave communications, this paper presents a deep neural network (DNN) based procedure to predict the angle of arrival (AOA) and angle of departure (AOD) both in terms of azimuth and elevation, i.e., AAOA/AAOD and EAOA/EAOD. In order to evaluate the performance of the proposed procedure under practical assumptions, we employ a trajectory prediction method by considering dynamic window approach (DWA) to estimate the location information of the user equipment (UE), which is utilized as the input parameter of the trained DNN to generate the prediction of AAOA/AAOD and EAOA/EAOD. The robustness of the prediction procedure is analyzed in the presence of prediction errors, which proves that the proposed DNN is a promising tool to predict AOA and AOD in NLOS scenarios based on the estimated UE location. Simulation results shows that the prediction errors of the AOA and AOD can be maintained within an acceptable range of +/- 2 degrees.
更多查看译文
关键词
Trajectory, Neural networks, Training, Predictive models, Millimeter wave communication, Mathematical model, Array signal processing, Deep learning, mmWave, NLOS, trajectory prediction, estimation
AI 理解论文
溯源树
样例
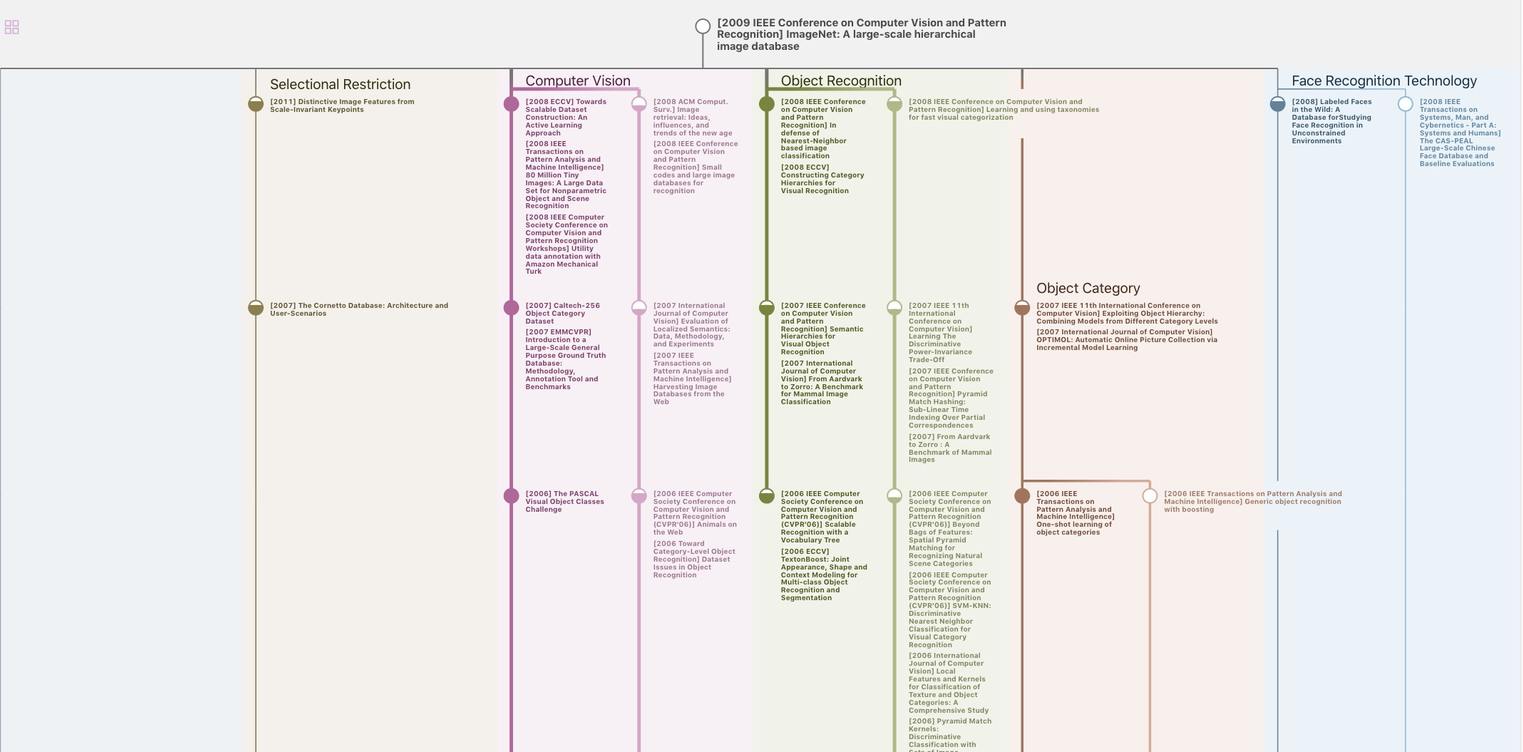
生成溯源树,研究论文发展脉络
Chat Paper
正在生成论文摘要