Large-scale Multiple Inference of Collective Dependence with Applications to Protein Function
Annals of applied statistics/The annals of applied statistics(2021)
摘要
Measuring the dependence of k ≥ 3 random variables and drawing inference from such higher-order dependences are scientifically important yet challenging. Motivated here by protein co-evolution with multivariate categorical features, we consider an information theoretic measure of higher-order dependence. The proposed collective dependence is a symmetrization of differential interaction information, which generalizes the mutual information of a pair of random variables. We show that the collective dependence can be easily estimated and facilitates a test on the dependence of k ≥ 3 random variables. Upon carefully exploring the null space of collective dependence, we devise a Classification-Assisted Large scaLe inference procedure to DEtect significant k-COllective DEpendence among d ≥ k random variables, with the false discovery rate controlled. Finite sample performance of our method is examined via simulations. We apply this method to the multiple protein sequence alignment data to study the residue or position co-evolution for two protein families: the elongation factor P family and the zinc knuckle family. We identify novel functional triplets of amino acid residues, whose contributions to the protein function are further investigated. These confirm that the collective dependence does yield additional information important for understanding the protein co-evolution compared to the pairwise measures.
更多查看译文
关键词
Collective dependence,false discovery rate,information theoretic measure,multiple testing,protein coevolution,structural biology
AI 理解论文
溯源树
样例
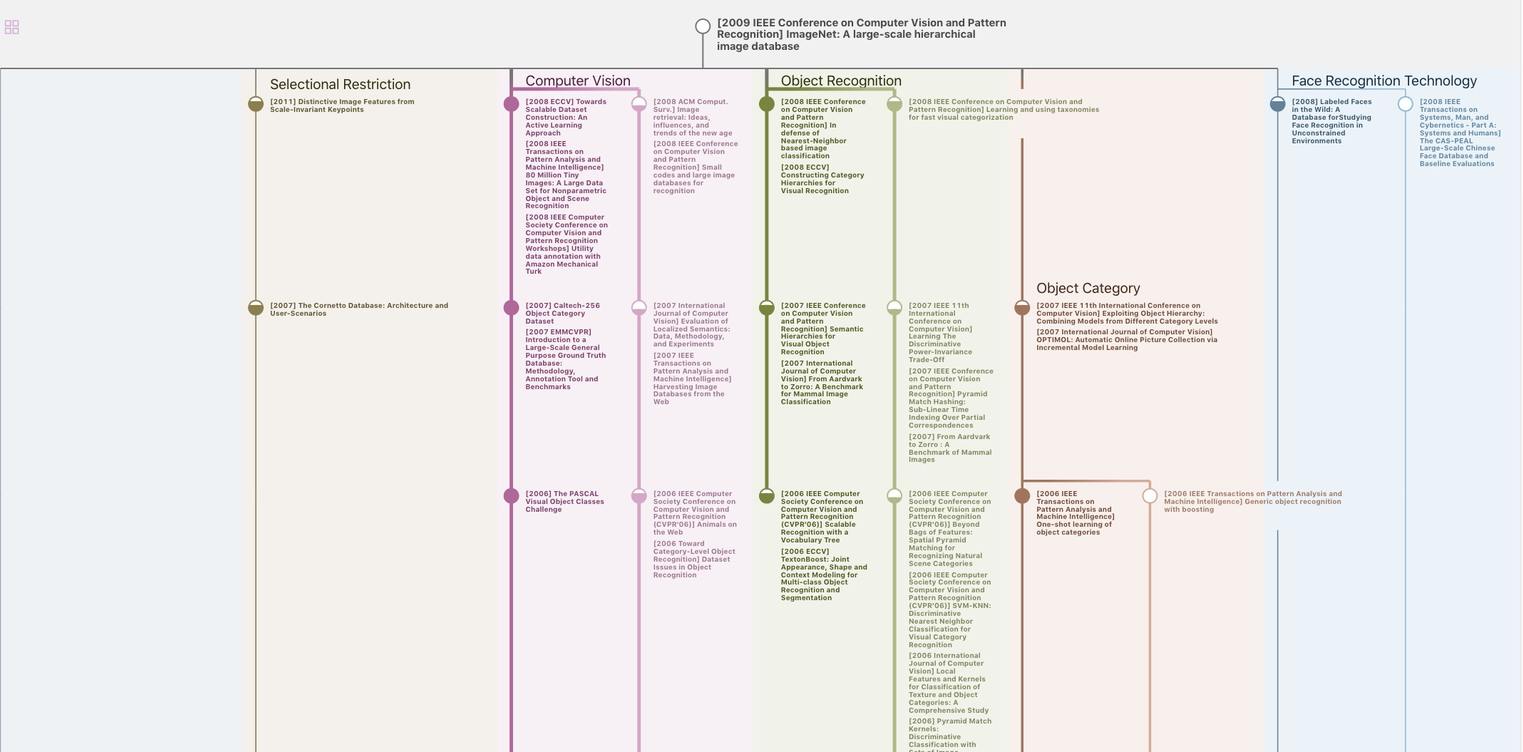
生成溯源树,研究论文发展脉络
Chat Paper
正在生成论文摘要