Estimating fine-scale movement rates and habitat preferences using multiple data sources
FISH AND FISHERIES(2021)
摘要
Fisheries scientists and managers must track rapid shifts in fish spatial distribution to mitigate stakeholder conflict and optimize survey designs, and these spatial shifts result in part from animal movement. Information regarding animal movement can be obtained from selection experiments, tagging studies, flux through movement gates (e.g. acoustic arrays), fishery catch-per-unit effort (CPUE), resource surveys and genetic/chemical markers. However, there are few accessible approaches to combine these data types while accounting for spatially correlated residual patterns. We therefore discuss a movement model involving diffusion (random movement), taxis (movement towards preferred habitat) and advection (passive drift following ocean currents). We specifically outline how these movement processes can be fitted to data while discretizing space and time and estimating non-linear habitat preferences using environmental layers as well as spatial process errors. Finally, we introduce an R package, ATM, by fitting the model to bottom trawl survey, longline fishery and tagging data for Pacific cod (Gadus macrocephalus, Gadidae) in the Bering Sea during winter/summer seasons from 1982 to 2019. Combining data types predicts an increasing proportion of cod residing in the northern Bering Sea from 2013 to 2019, and estimates are informative in a recent stock assessment model. We fit sensitivity analyses by dropping tag, survey or fishery data, and this analysis shows that tagging data are necessary to identify diffusion rates, while survey data are informative about movement among biogeographic strata. This "hybrid" species distribution model can help explain poleward movement, project distributions under future climate conditions and evaluate alternative tag-deployment scenarios to optimize tagging designs.
更多查看译文
关键词
advection, diffusion, habitat preference, instantaneous movement, Pacific cod, taxis
AI 理解论文
溯源树
样例
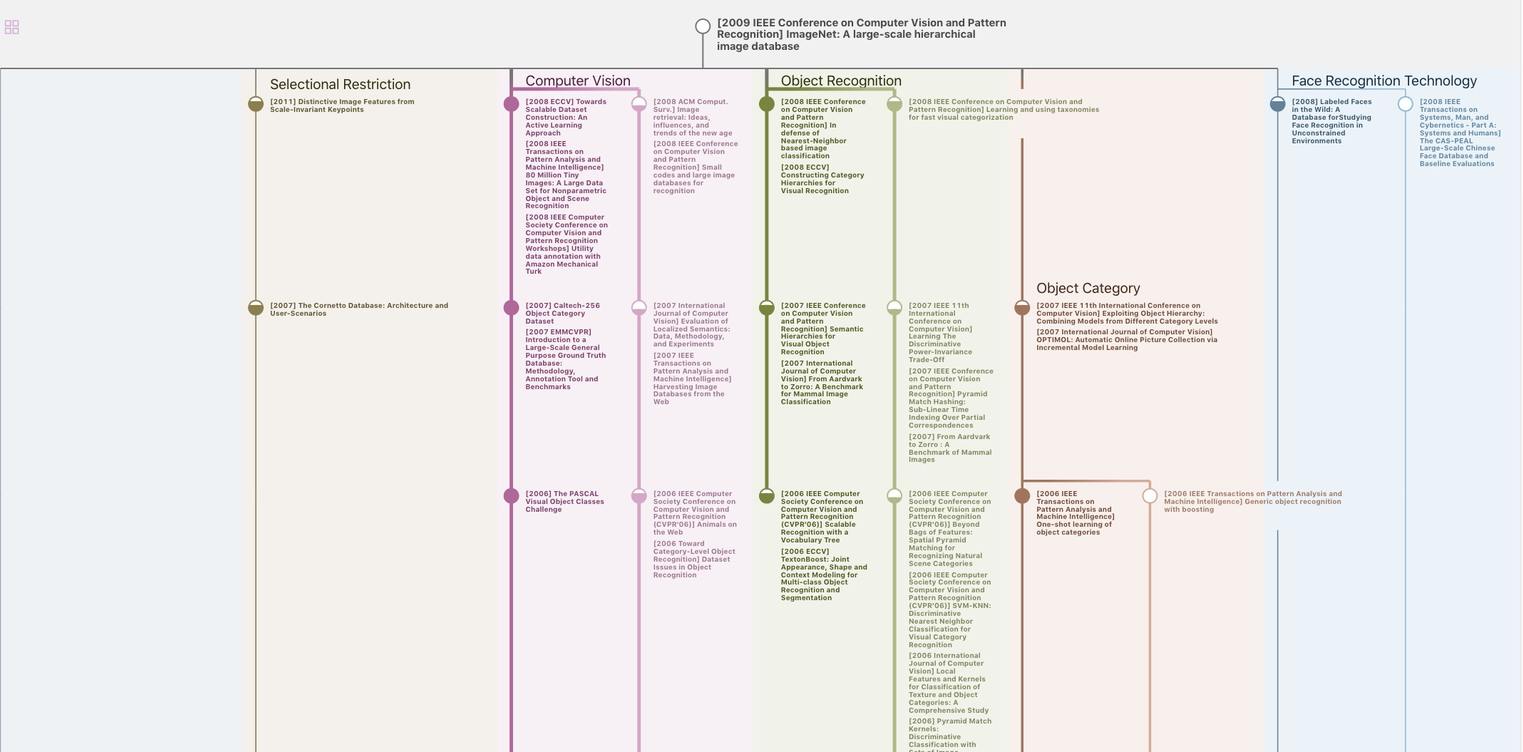
生成溯源树,研究论文发展脉络
Chat Paper
正在生成论文摘要