Fault Diagnosis Method of the Construction Machinery Hydraulic System Based on Artificial Intelligence Dynamic Monitoring
Periodicals(2021)
摘要
AbstractThis paper aims to study the fault diagnosis method of the mechanical hydraulic system based on artificial intelligence dynamic monitoring. According to the characteristics of functional principal component analysis (FPCA) and neural network in the fault diagnosis method in the feature extraction process, the fault diagnosis method combining functional principal component analysis and BP neural network is studied and it is applied to the fault of the coordinator hydraulic system diagnosis. This article mainly completed the following tasks: analyzing the structure and working principle of the mechanical hydraulic system, studying the failure mechanism and failure mode of the mechanical hydraulic system, summarizing the common failures of the hydraulic system and the individual failures of the mechanical hydraulic system, and establishing the mechanical hydraulic system. Description of failure mode and effects analysis (FMEA): then, a joint simulation model of the mechanical hydraulic system was established in ADAMS and AMESim, and the fault detection signal of the hydraulic system was determined and compared with the experimental data. At the same time, the simulation data of the cosimulation model were compared with the simulation data of the hydraulic model in MATLAB to further verify the correctness of the model. The functional principal component analysis is used to perform functional processing on sample data, feature parameters are extracted, and the BP neural network is used to train the mapping relationship between feature parameters and fault parameters. The consistency is verified, and the fault diagnosis method is finally completed. The experimental results show that the diagnostic accuracy rates are 0.9848 and 0.9927, respectively, the reliability is significantly improved, close to 100%, and the uncertainty is basically 0, which significantly improves the accuracy of fault diagnosis.
更多查看译文
AI 理解论文
溯源树
样例
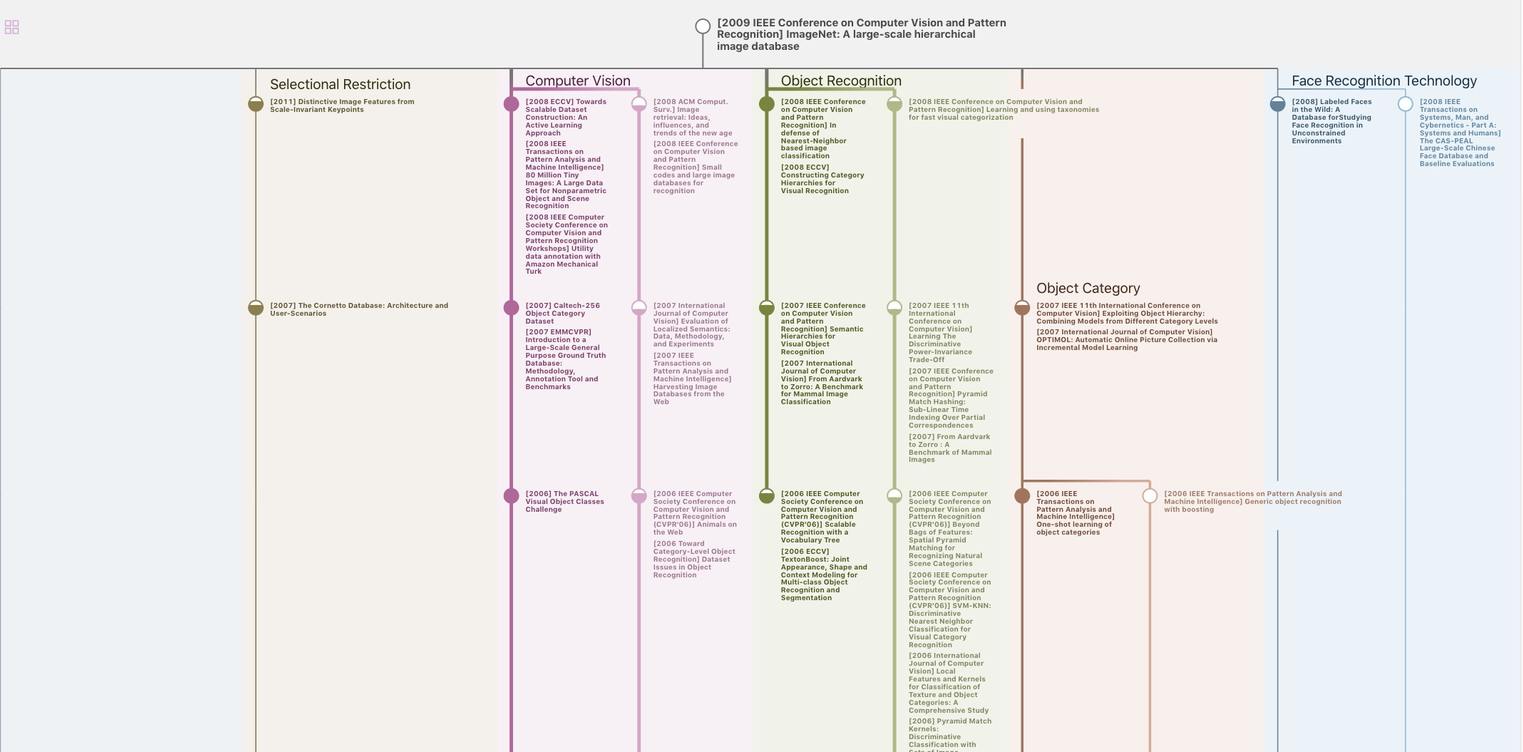
生成溯源树,研究论文发展脉络
Chat Paper
正在生成论文摘要