Improving the speed of global parallel optimization on PDE models with processor affinity scheduling
COMPUTER-AIDED CIVIL AND INFRASTRUCTURE ENGINEERING(2022)
摘要
Parallel global optimization of expensive simulation models like nonlinear partial differential equations (PDEs) can speed up model calibration or project design decisions, but the impact of memory management on the efficiency of using parallel global optimization methods has not been previously studied. This paper quantifies cache memory limitations arising during parallel optimization of expensive PDE models. An efficient parallel optimization algorithm is applied to model calibration for two different, expensive real-world PDEs (i.e., hydrodynamic and water quality analysis for a 250-hectare lake). One of these two lake models takes 4.5 h per simulation in serial, but that PDE simulation time per simulation increases to 12 h with parallel optimization if default processor scheduling strategy is used on a modern nonuniform memory access multicore platform. We proposed a novel mixed affinity scheduling strategy for parallel simulation optimization that increases computational efficiency by as much as 20% over the default affinity strategy.
更多查看译文
AI 理解论文
溯源树
样例
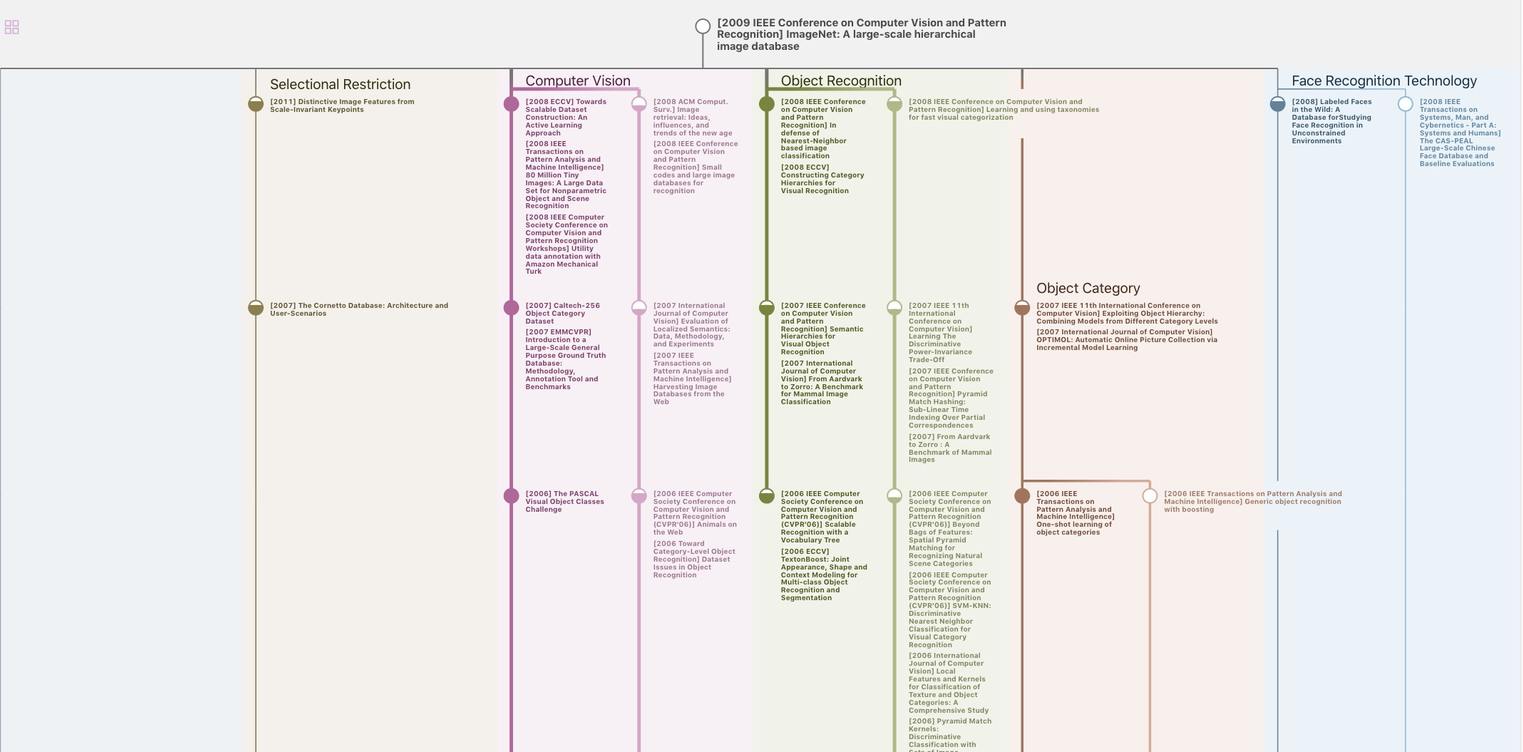
生成溯源树,研究论文发展脉络
Chat Paper
正在生成论文摘要