An Asynchronous Quasi-Cloud/Edge/Client Collaborative Federated Learning Mechanism For Fault Diagnosis
CHINESE JOURNAL OF ELECTRONICS(2021)
摘要
Although the federated learning method has the ability to balance data and protect data privacy by means of model aggregation, while the existing methods are difficult to achieve the effectiveness of centralized learning under data sharing. The existing federated structure only has a certain degree of confidentiality for data privacy, that is to say, each client can reconstruct a part of the information of other clients based on the model parameters shared between the server and the clients under certain conditions. In order to make the federated learning mechanism more confidential, we breaks the existing mechanism that the parameters between the federated model and the client model are completely shared, and establishes a new asynchronous quasi-cloud/edge/client collaborative federated learning mechanism. We construct a hierarchical multi-level confidential communication network, where the network parameters are shared in a way of quasi-cloud/edge/client coordination without data communication. The cloud and the edges respectively use the sequential Kalman filter algorithm to perform an asynchronous fusion of the network parameters uploaded in their respective fusion centers for the next round of updates; The effectiveness of the proposed algorithm is verified on the data of a type of rotating machinery
更多查看译文
关键词
Federated learning, Quasi-cloud, edge, client collaboration, Multi-level confidentiality, Asynchronous fusion, Fault diagnosis
AI 理解论文
溯源树
样例
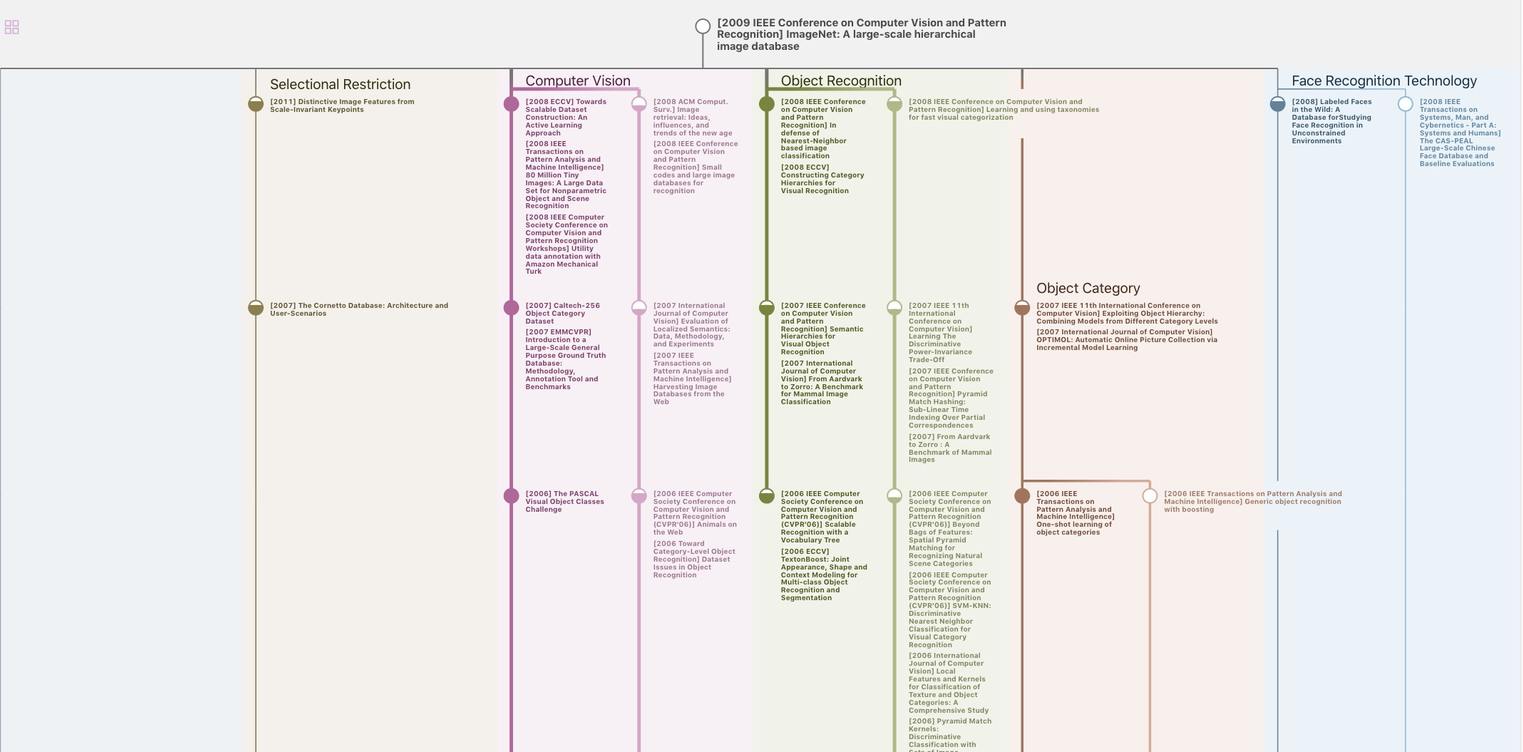
生成溯源树,研究论文发展脉络
Chat Paper
正在生成论文摘要