GSA-ELM: A hybrid learning model for short-term traffic flow forecasting
IET INTELLIGENT TRANSPORT SYSTEMS(2022)
摘要
Accurate and timely short-term traffic flow forecasting is an essential component for intelligent traffic management systems. However, developing an effective and robust forecasting model is challenging due to the inherent randomness and nonlinear characteristic of the traffic flow. In this paper, a gravitational search algorithm optimized extreme learning machine, termed GSA-ELM, is proposed to unlock the potential performance for short-term traffic flow forecasting. The extreme learning machine avoids the tedious backpropagation by analytically determining the optimal solution. The gravitational search algorithm globally searches the optimal parameters for the extreme learning machine. The forecasting performance of the GSA-ELM is evaluated on four benchmark datasets by comparing several state-of-the-art models. The four benchmark datasets are real-world traffic flow data from highways A1, A2, A4, A8 near the ring road of Amsterdam. The MAPEs of the GSA-ELM model are 11.69%, 10.25%, 11.72% and 12.05% on four benchmark datasets, respectively, whereas the RMSEs of the GSA-ELM model are 287.89, 203.04, 221.39 and 163.24, respectively. The experimental results demonstrate the superior performance of the proposed model.
更多查看译文
关键词
Optimisation techniques,Traffic engineering computing,Other topics in statistics,Combinatorial mathematics,Machine learning (artificial intelligence),Neural nets
AI 理解论文
溯源树
样例
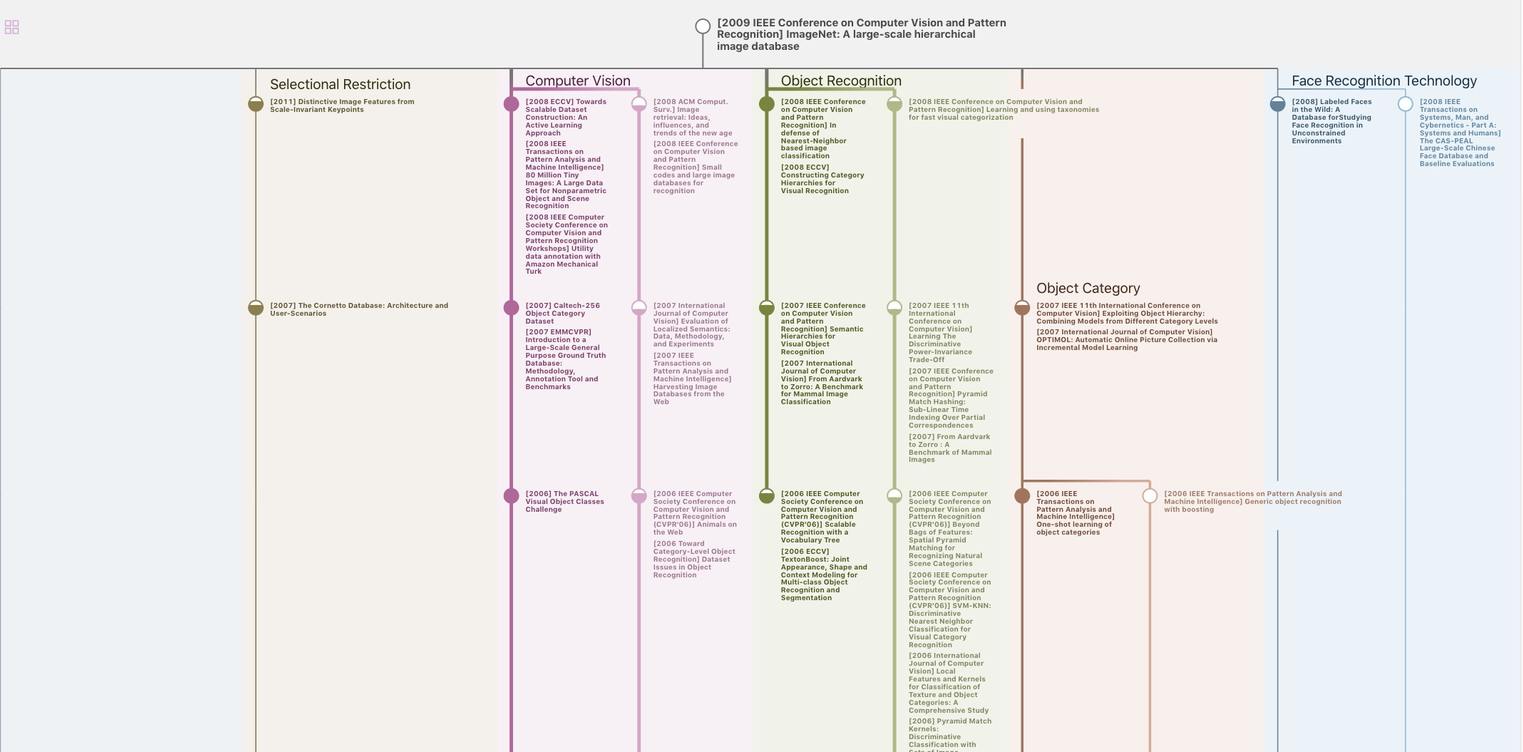
生成溯源树,研究论文发展脉络
Chat Paper
正在生成论文摘要