Macro: Multi-Attention Convolutional Recurrent Model For Subject-Independent Erp Detection
IEEE SIGNAL PROCESSING LETTERS(2021)
摘要
Due to the low signal-to-noise ratio, limited training samples, and large inter-subject variabilities in electroencephalogram (EEG) signals, developing a subject-independent brain-computer interface (BCI) system used for new users without any calibration is still challenging. In this letter, we propose a novel Multi-Attention Convolutional Recurrent mOdel (MACRO) for EEG-based event-related potential (ERP) detection in the subject-independent scenario. Specifically, the convolutional recurrent network is designed to capture the spatial-temporal features, while the multi-attention mechanism is integrated to focus on the most discriminative channels and temporal periods of EEG signals. Comprehensive experiments conducted on a benchmark dataset for RSVP-based BCIs show that our method achieves the best performance compared with the five state-of-the-art baseline methods. This result indicates that our method is able to extract the underlying subject-invariant EEG features and generalize to unseen subjects. Finally, the ablation studies verify the effectiveness of the designed multi-attention mechanism in MACRO for EEG-based ERP detection.
更多查看译文
关键词
Electroencephalography, Integrated circuits, Feature extraction, Convolution, Kernel, Brain modeling, Training, EEG, ERP detection, subject-independent, attention mechanism, deep learning
AI 理解论文
溯源树
样例
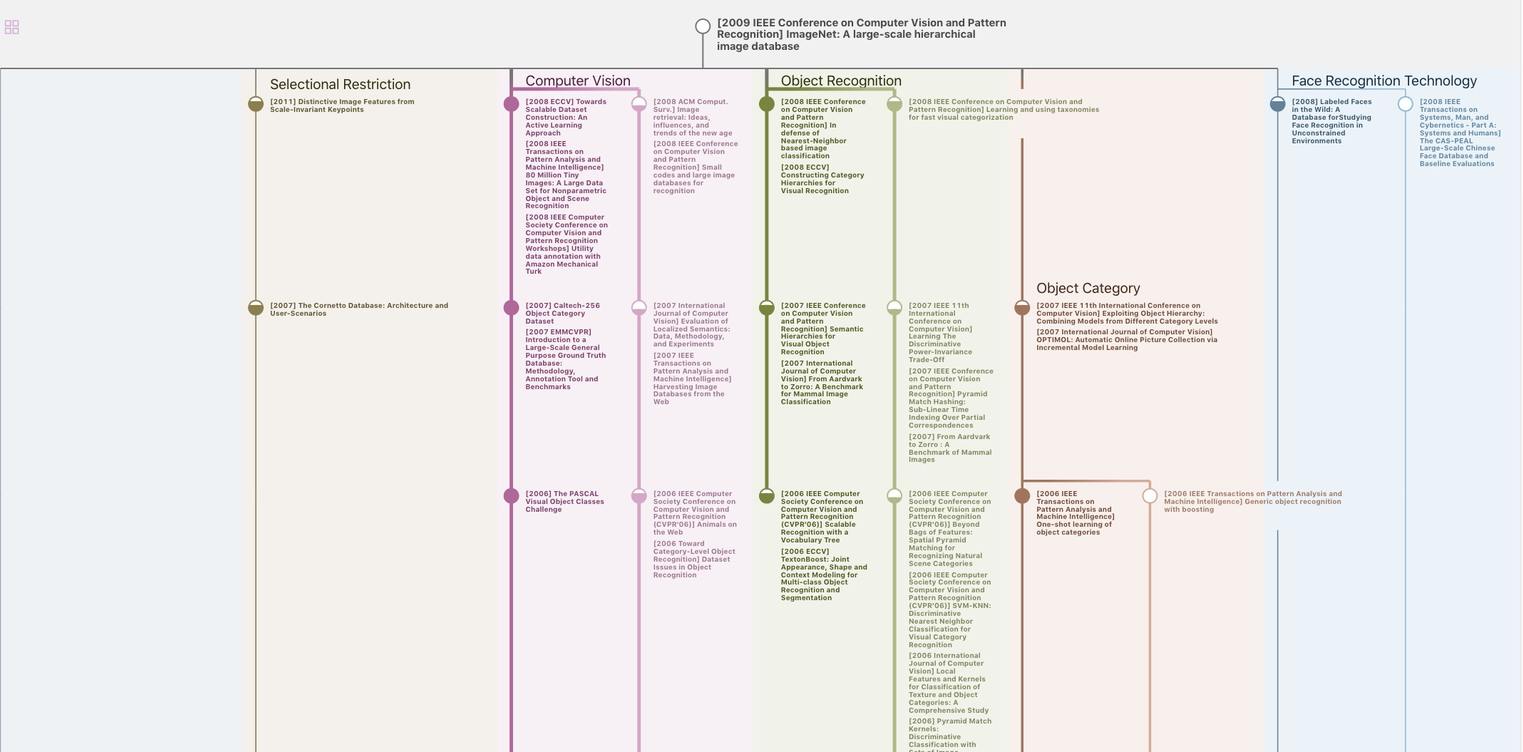
生成溯源树,研究论文发展脉络
Chat Paper
正在生成论文摘要