Familiarity-dependent computational modelling of indoor landmark selection for route communication: a ranking approach
INTERNATIONAL JOURNAL OF GEOGRAPHICAL INFORMATION SCIENCE(2022)
摘要
Landmarks play key roles in human wayfinding and mobile navigation systems. Existing computational landmark selection models mainly focus on outdoor environments, and aim to identify suitable landmarks for guiding users who are unfamiliar with a particular environment, and fail to consider familiar users. This study proposes a familiarity-dependent computational method for selecting suitable landmarks for communicating with familiar and unfamiliar users in indoor environments. A series of salience measures are proposed to quantify the characteristics of each indoor landmark candidate, which are then combined in two LambdaMART-based learning-to-rank models for selecting landmarks for familiar and unfamiliar users, respectively. The evaluation with labelled landmark preference data by human participants shows that people's familiarity with environments matters in the computational modelling of indoor landmark selection for guiding them. The proposed models outperform state-of-the-art models, and achieve hit rates of 0.737 and 0.786 for familiar and unfamiliar users, respectively. Furthermore, semantic relevance of a landmark candidate is the most important measure for the familiar model, while visual intensity is most informative for the unfamiliar model. This study enables the development of human-centered indoor navigation systems that provide familiarity-adaptive landmark-based navigation guidance.
更多查看译文
关键词
Indoor navigation, landmark selection, familiarity with an environment, computational landmark salience model, learning to rank
AI 理解论文
溯源树
样例
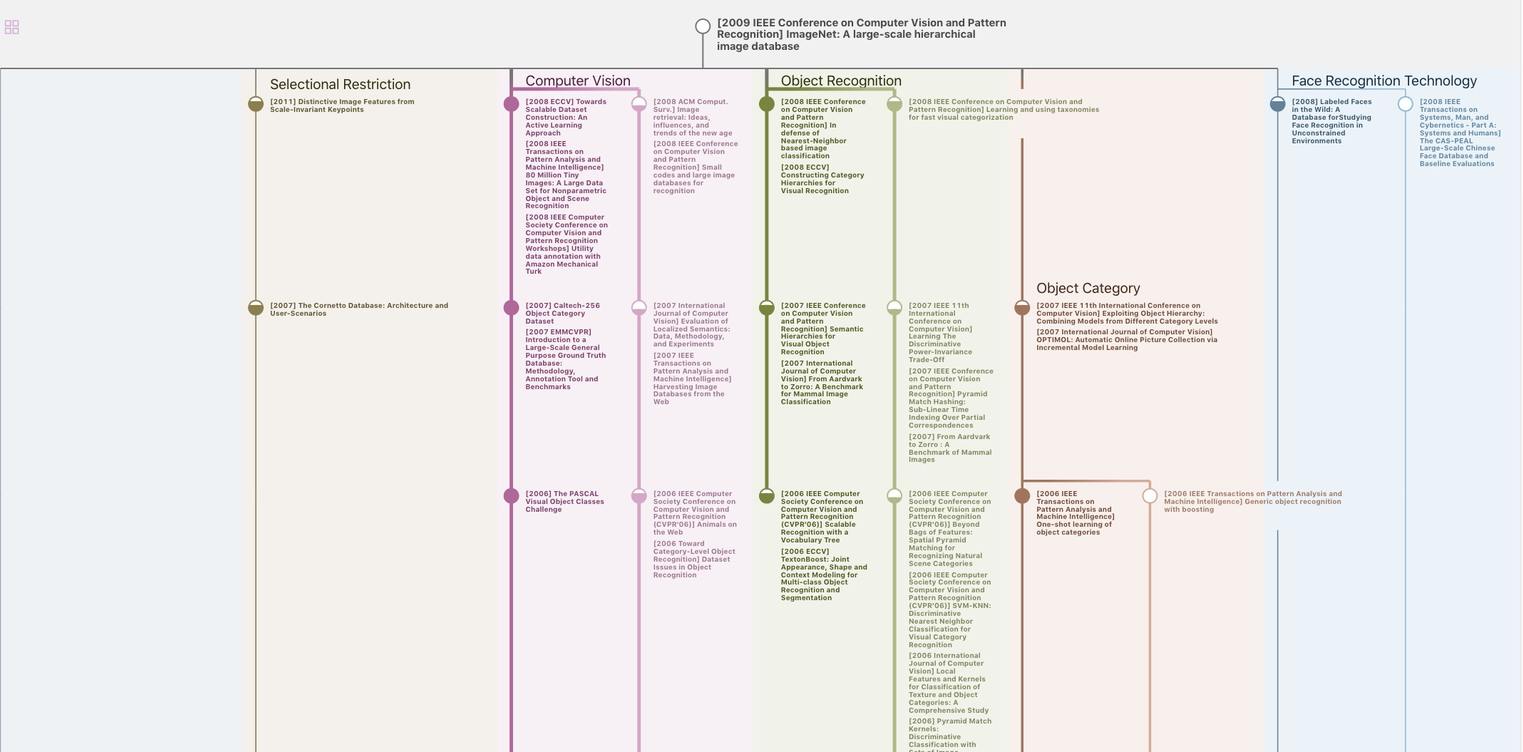
生成溯源树,研究论文发展脉络
Chat Paper
正在生成论文摘要