Exploring the Effect of Temporal Aggregation on SCADA Data for Wind Turbine Prognosis Using a Normality Model
Applied sciences(2021)
Abstract
Today, the use of SCADA data for predictive maintenance and forecasting of wind turbines in wind farms is gaining popularity due to the low cost of this solution compared to others that require the installation of additional equipment. SCADA data provides four statistical measures (mean, standard deviation, maximum value, and minimum value) of hundreds of wind turbine magnitudes, usually in a 5-min or 10-min interval. Several studies have analysed the loss of information associated with the reduction of information when using five minutes instead of four seconds as a sampling frequency, or when compressing a time series recorded at 5 min to 10 min, concluding that some, but not all, of these magnitudes are seriously affected. However, to our knowledge, there are no studies on increasing the time interval beyond 10 min to take these four statistical values, and how this aggregation affects prognosis models. Our work shows that, despite the irreversible loss of information that occurs in the first 5 min, increasing the time considered to take the four representative statistical values improves the performance of the predicted targets in normality models.
MoreTranslated text
Key words
wind turbine,fault diagnosis,renewable energy,extreme learning machines (ELM),feature engineering,normal behaviour models
AI Read Science
Must-Reading Tree
Example
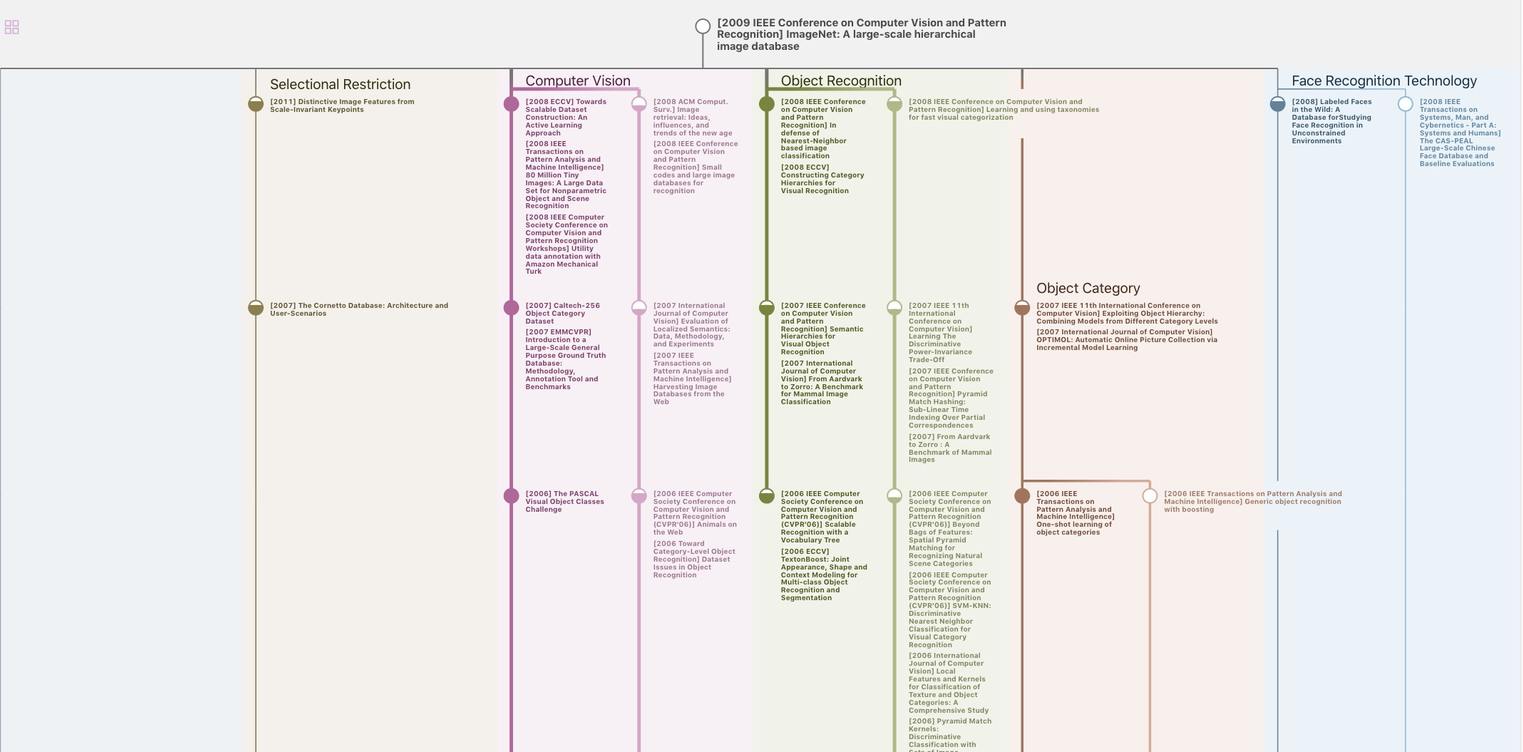
Generate MRT to find the research sequence of this paper
Chat Paper
Summary is being generated by the instructions you defined