Multi-Label Lstm Autoencoder For Non-Intrusive Appliance Load Monitoring
ELECTRIC POWER SYSTEMS RESEARCH(2021)
摘要
This work follows the multi-label classification based paradigm for non-intrusive load monitoring (NILM). Power consumption signals used for NILM are inherently time varying. However prior multi-label classification techniques could not model this dynamical behaviour. They used off-the-shelf algorithms for classifying static signals on NILM problems. This is the first work that shows how to account for the temporal variability of input signals in a multi-label classification framework. Results on benchmark datasets like REDD and Pecan Street show considerable improvement over several state-of-the-art shallow and deep techniques.
更多查看译文
关键词
Deep learning, LSTM, Autoencoder, Non-intrusive load monitoring, Energy disaggregation
AI 理解论文
溯源树
样例
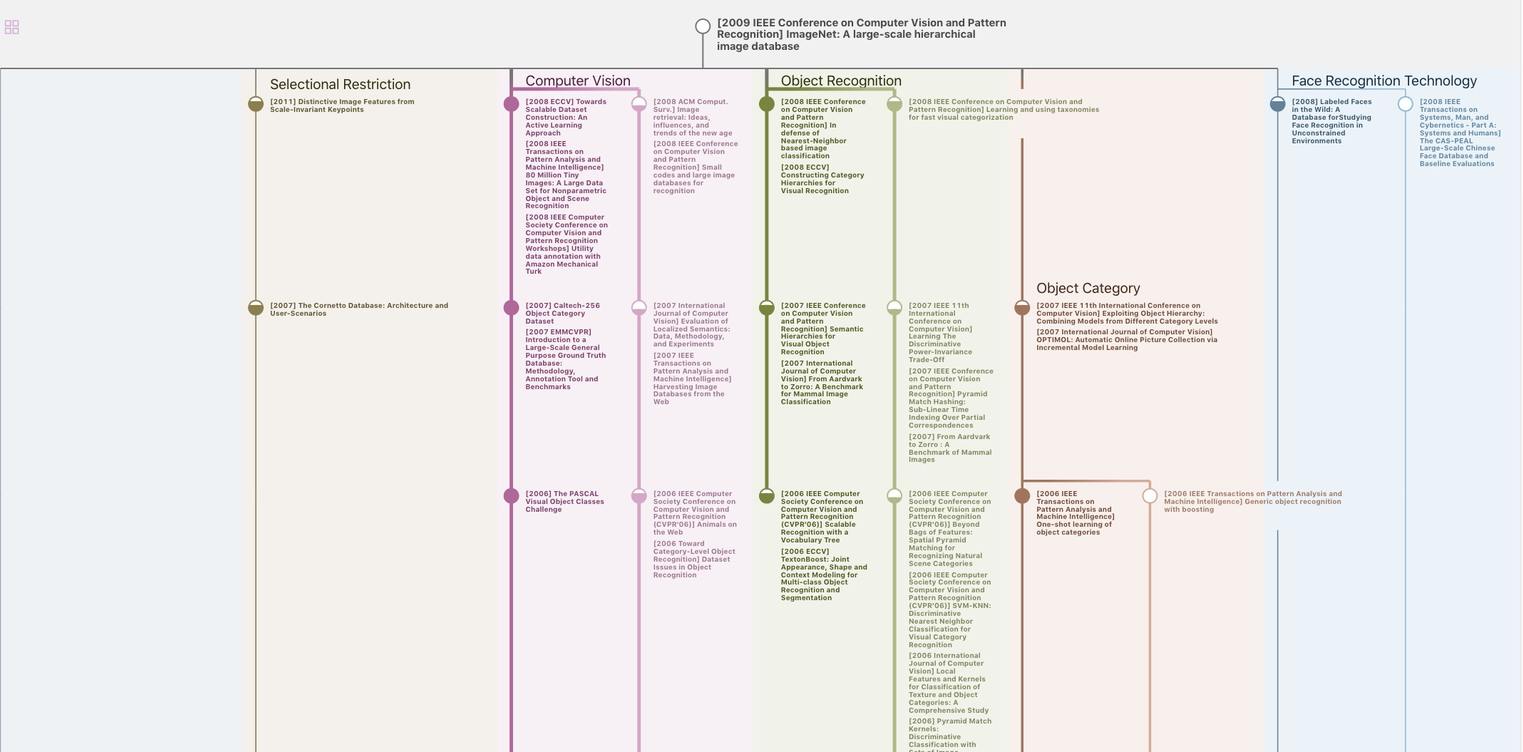
生成溯源树,研究论文发展脉络
Chat Paper
正在生成论文摘要