An Attention-Basedcnn-Lstm-Bilstmmodel For Short-Term Electric Load Forecasting In Integrated Energy System
INTERNATIONAL TRANSACTIONS ON ELECTRICAL ENERGY SYSTEMS(2021)
摘要
In recent years, diverse energy has been integrated into the power system, which constitutes a regional integrated energy system (IES). However, the coupling and complementation of multiple energy sources make load forecasting more difficult. For the time-sequence and non-linear characteristics of electric load and the complementarity of different energy in IES, this paper proposed an attention-based convolutional neural network (CNN) combined with long short-term memory (LSTM) and bidirectional long short-term memory (BiLSTM) model for short-term load forecasting in IES. The historical load, temperature, cooling load, and gas consumption of the past 5 days are used as the input features. CNN integrated with attention block is utilized to extract effective features of the load impact factors. Then the load of the next hour is forecasted by the LSTM combined with BiLSTM layers. Finally, the model is verified by the data from an integrated energy park in North China. The results show that the proposed method has better forecasting performance than CNN-BiLSTM, CNN-LSTM, BiLSTM, LSTM, backpropagation neural network (BPNN), random forest regression (RFR), and support vector machine regression (SVR).
更多查看译文
关键词
attention mechanism, bidirectional, convolutional neural network, integrated energy system, long short-term memory, short-term electric load forecasting
AI 理解论文
溯源树
样例
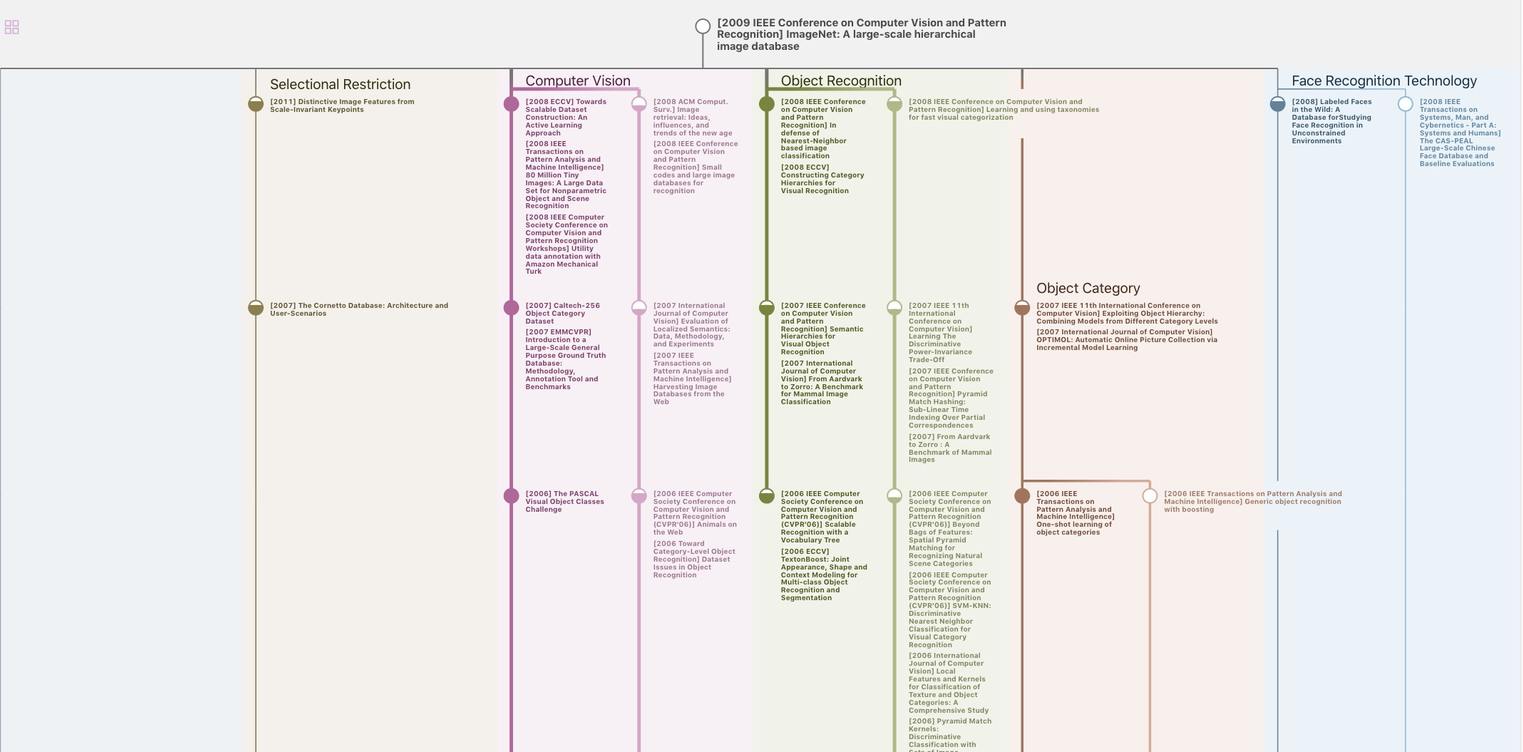
生成溯源树,研究论文发展脉络
Chat Paper
正在生成论文摘要