Effectively clustering researchers in scientific collaboration networks: case study on ResearchGate
SOCIAL NETWORK ANALYSIS AND MINING(2021)
摘要
Social networks play a significant role in sharing knowledge. Scientific collaboration online networks allow scientific articles and research results to be shared, and the interaction and possible collaboration between researchers. These networks have many users and store varied data about each of them, and which of the data are used to characterize and grouping similar users. The number of attributes available about each instance (user) can reach several hundred, making this a problem with high dimensionality. Thus, dimensionality reduction is indispensable to remove redundant and irrelevant attributes to improve machine learning algorithms’ performance and make models more understandable. In order to produce an efficient recommendation system for collaborative research, one of the main challenges of dimensionality reduction techniques is guaranteeing that the information of the data is represented in the reduced dataset after the reduction. In our dimensionality reduction, we used Factor Analysis, as it preserves the relationships between the variables. In this study, we characterize the profiles of ResearchGate users after applying dimensionality reduction to two different datasets. A dataset of continuous attributes composed of profile metrics and a dataset of dichotomous attributes contained interest topics. We evaluated our methodology using two recommendation applications: (1) Identifying groups of researchers through a global profile extraction process; and (2) Identifying profiles similar to a reference profile. For both applications, we used hierarchical clustering techniques to identify the groups of user profiles. Our experiments show that the Factor Analysis transformation was able to preserve the relevant information in the data, resulting in an effective clustering process for the recommendation system for collaborative networks of researchers.
更多查看译文
关键词
Scientific collaboration network, Continuous and dichotomous attributes, Knowledge extraction, Attributes reduction
AI 理解论文
溯源树
样例
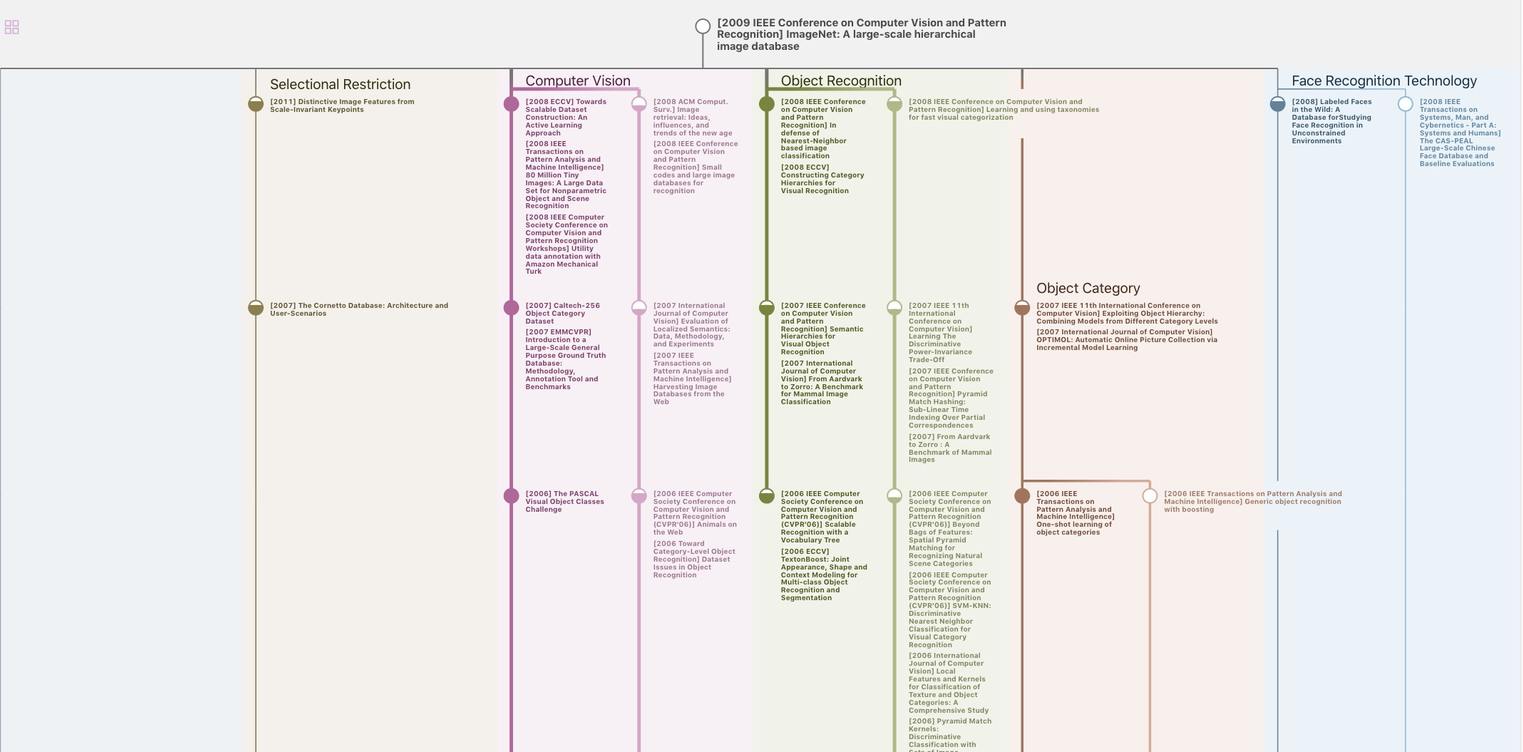
生成溯源树,研究论文发展脉络
Chat Paper
正在生成论文摘要