Faster R-CNN Based Defect Detection of Micro-precision Glass Insulated Terminals
PROCEEDINGS OF THE 2021 INTERNATIONAL CONFERENCE ON ARTIFICIAL LIFE AND ROBOTICS (ICAROB 2021)(2021)
摘要
Micro-precision glass insulated terminals (referred to as glass terminals) are the core components used in precision electronic equipment. As glass terminal, its quality has a huge impact on the performance of precision electronic equipment. Due to limitations in materials and production processes, some of the glass terminals produced have defects such as missing blocks, bubbles, and cracks. At present, it is difficult to ensure product quality and production efficiency with manual inspection methods. However, the defect characteristics of glass terminals are quite different, and it is difficult for traditional defect detection technology to design an ideal feature extractor for detection. Therefore, this paper proposes to use deep learning technology to detect missing blocks. First, preprocess the sample pictures of missing block defects of glass terminals, and then train the deep learning network based on Faster RCNN. According to the test results, the algorithm has an accuracy of over 90% in detecting missing defects in glass terminals.
更多查看译文
关键词
Micro-precision Glass Insulated Terminal, Faster R-CNN, Missing Block Detection
AI 理解论文
溯源树
样例
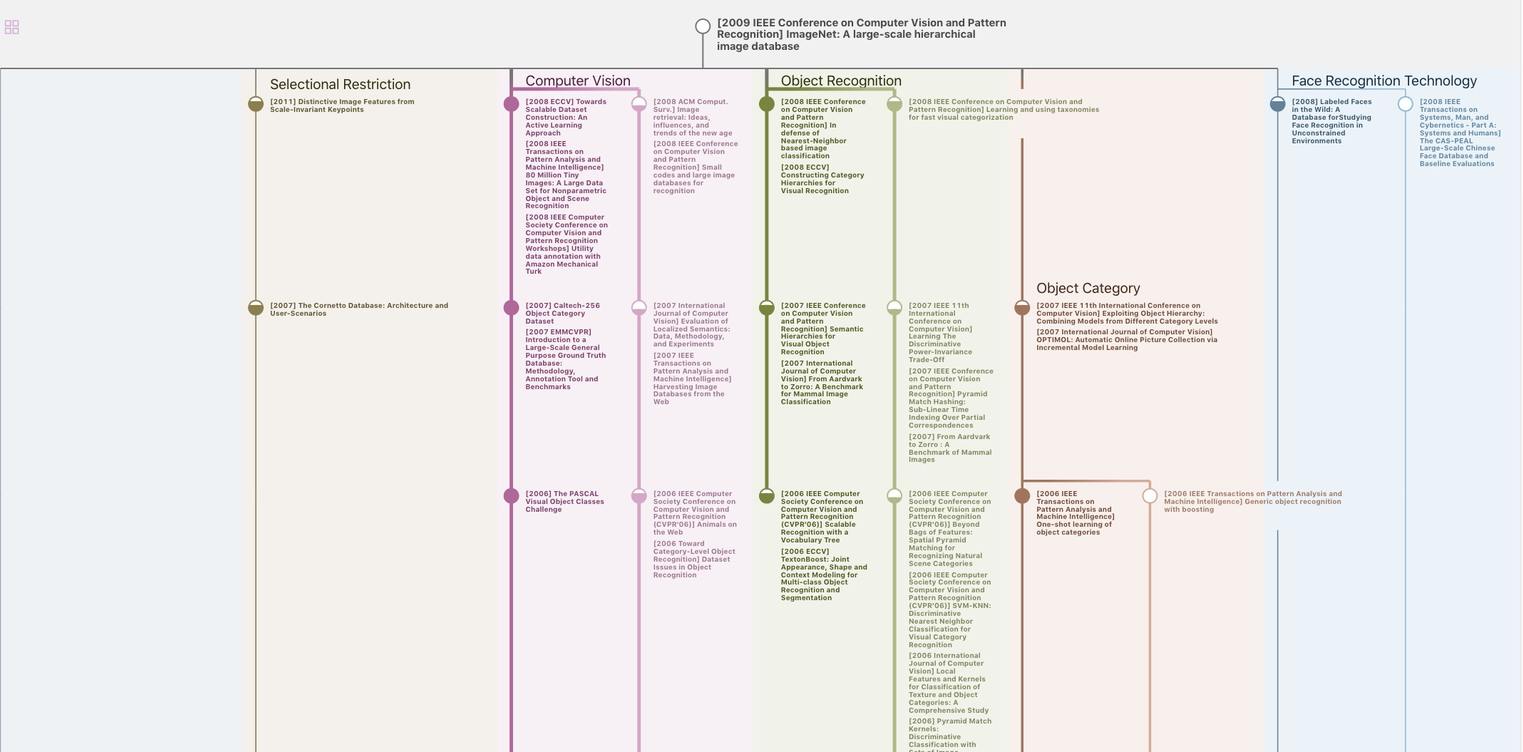
生成溯源树,研究论文发展脉络
Chat Paper
正在生成论文摘要