Likelihood Identification Of High-Beta Disruption In Jt-60u
PLASMA AND FUSION RESEARCH(2021)
摘要
Prediction and likelihood identification of high-beta disruption in JT-60U has been discussed by means of feature extraction based on sparse modeling. In disruption prediction studies using machine learning, the selection of input parameters is an essential issue. A disruption predictor has been developed by using a linear support vector machine with input parameters selected through an exhaustive search, which is one idea of sparse modeling. The investigated dataset includes not only global plasma parameters but also local parameters such as ion temperature and plasma rotation. As a result of the exhaustive search, five physical parameters, i.e., normalized beta beta(N), plasma elongation kappa, ion temperature T-i and magnetic shear s at the q = 2 rational surface, have been extracted as key parameters of high-beta disruption. The boundary between the disruptive and the non-disruptive zones in multidimensional space has been defined as the power law expression with these key parameters. Consequently, the disruption likelihood has been quantified in terms of probability based on this boundary expression. Careful deliberation of the expression of the disruption likelihood, which is derived with machine learning, could lead to the elucidation of the underlying physics behind disruptions. (C) 2021 The Japan Society of Plasma Science and Nuclear Fusion Research
更多查看译文
关键词
tokamak, JT-60U, disruption prediction, sparse modeling, machine learning
AI 理解论文
溯源树
样例
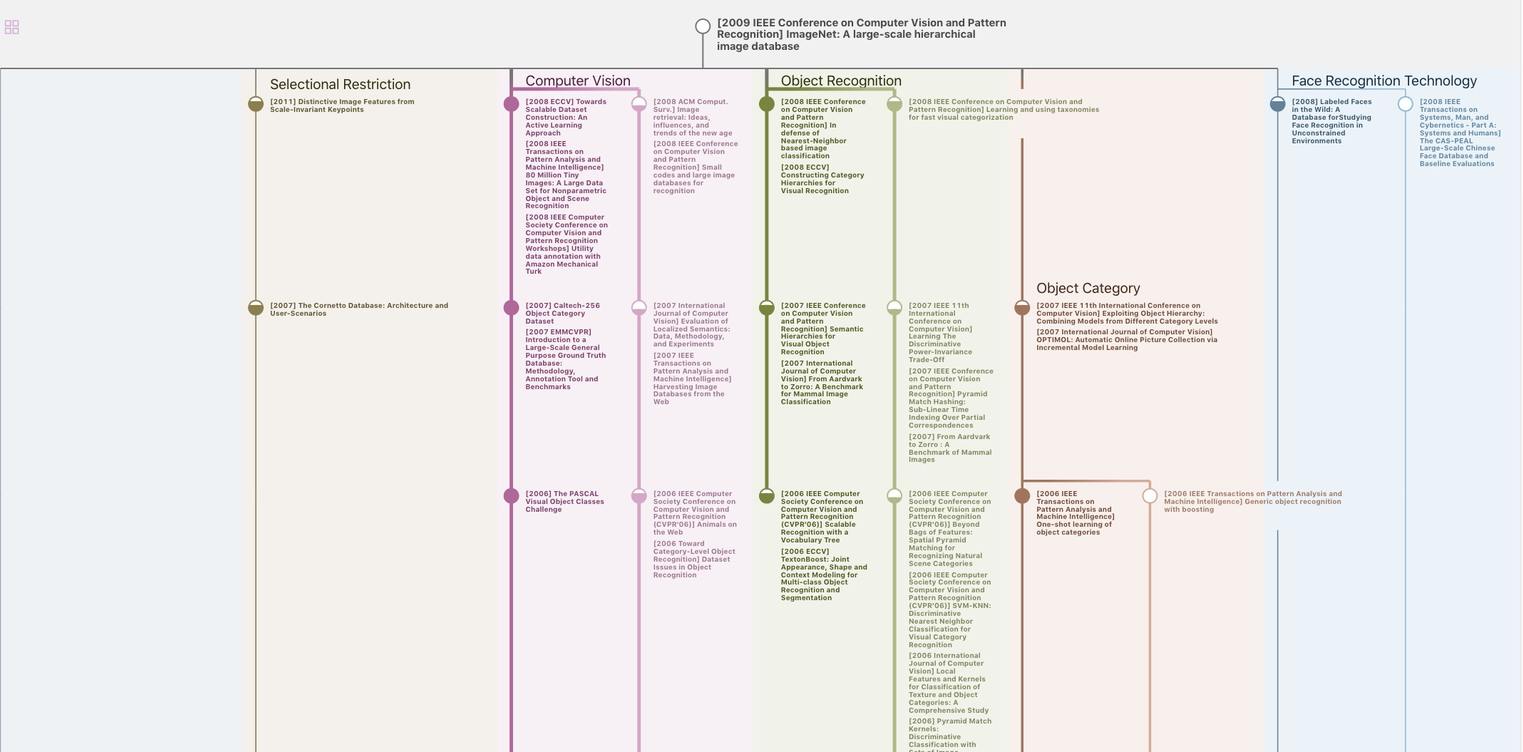
生成溯源树,研究论文发展脉络
Chat Paper
正在生成论文摘要