1141P Prediction of Cancer Genomic Instability Using MALDI-imaging
Annals of oncology(2021)
摘要
Disruption of the homologous recombination repair (HRR) pathway was reported in at least half of high-grade serous ovarian cancer (HGSOC) patients. HRR deficiency (HRD) is associated with superior response to PARP inhibition maintenance therapy in both primary and relapsed HGSOC. Moreover, HRD is a prerequisite for combination therapy with Bevacizumab and Olaparib following first-line chemotherapy. Aim of this study was to evaluate if a machine learning framework trained on matrix-assisted laser desorption/ionization imaging mass spectroscopy (MALDI-IMS) data can accurately predict the HRD status in HGSOC tumors. We collected during surgery paired formalin-fixed paraffin-embedded (FFPE) tumor samples from primary and at relapse in 50 HGSOC patients. HRD status was previously evaluated using the Myriad myChoice® CDx test, that was conclusive in 88 samples. HRD status served as the reference label for machine learning of MALDI-IMS data generated from the same samples. Models achieved a 96.8% mean accuracy and 0.995 mean area under the curve (AUC) on three randomized subsets of the data. Utilizing a majority vote scheme, all patients could be assigned the correct HRD status. Using an accessible, cheaper and easy to reproduce method such as MALDI-IMS technology combined with a machine learning framework we reached a similar performance to the Myriad myChoice® CDx test in predicting HRD status in HGSOC.
更多查看译文
AI 理解论文
溯源树
样例
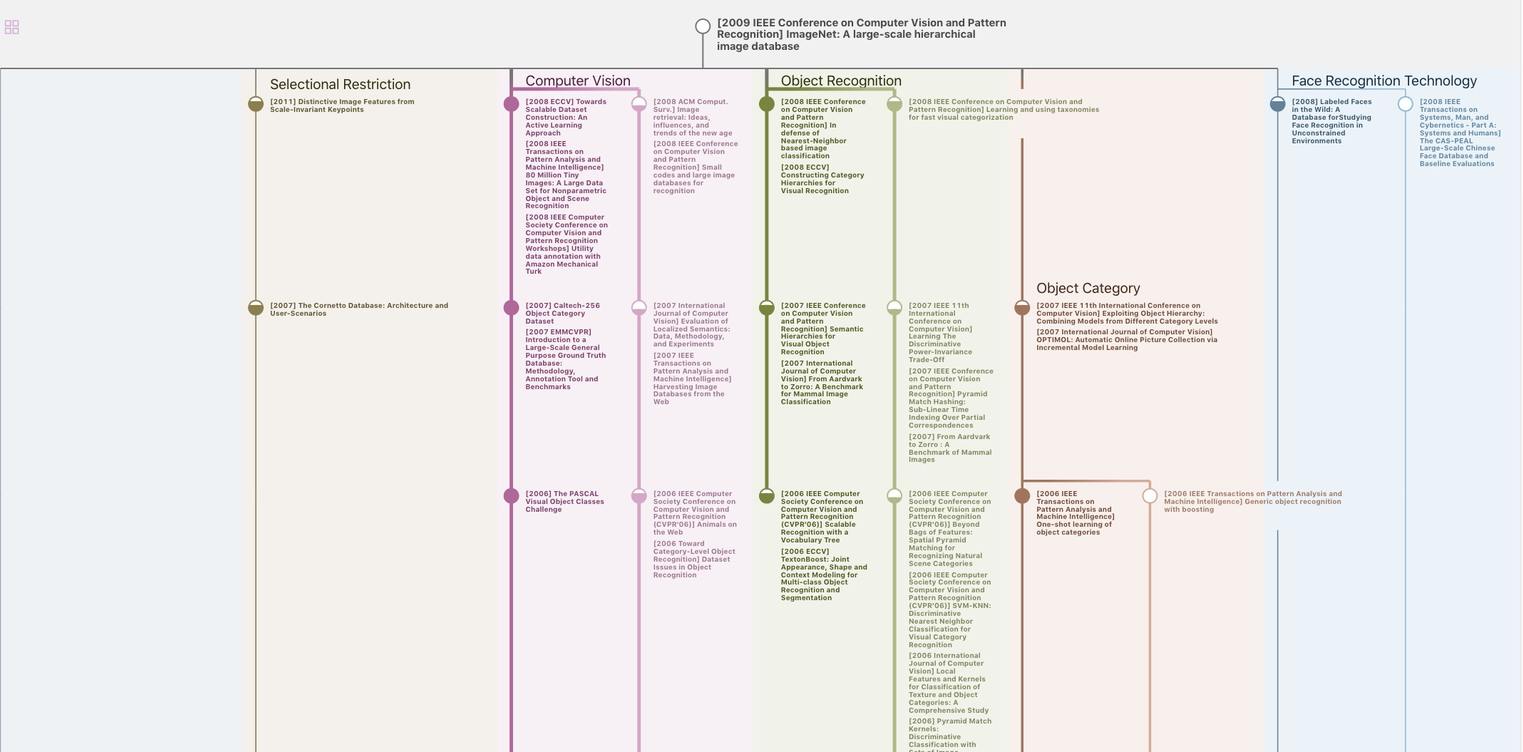
生成溯源树,研究论文发展脉络
Chat Paper
正在生成论文摘要